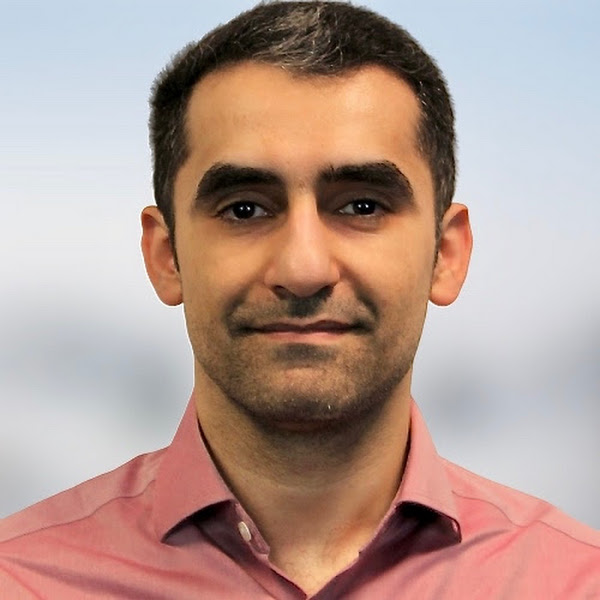
Hamza Harkous
I am a Staff Research Scientist at Google, Zürich. I currently work on techniques and systems for revamping synthetic data generation with large language models, taking agentic approaches focused on data diversity and complexity.
Before that, I lead an effort to transform the data curation and model building process with LLMs, driving advancements in privacy, safety, security, and beyond across Google's products.
I previously architected the machine learning models behind Google Checks, the privacy compliance service. I also built core models behind Hark, one of Google's internal systems for feedback analysis, through which all user feedback goes every day.
Prior to Google, I worked at Amazon Alexa on natural language understanding and generation. I received my PhD in Computer Science from the Swiss Federal Institute of Technology in Lausanne (EPFL), where I also served as a postdoctoral researcher. During that time, I researched and developed tools for improving users’ comprehension of privacy practices and for automatically analyzing privacy policies.
You can find more about my work on my personal homepage.
Authored Publications
Sort By
Google
Website Data Transparency in the Browser
Sebastian Zimmeck
Daniel Goldelman
Owen Kaplan
Logan Brown
Justin Casler
Judeley Jean-Charles
Joe Champeau
24th Privacy Enhancing Technologies Symposium (PETS 2024), PETS (to appear)
A Decade of Privacy-Relevant Android App Reviews: Large Scale Trends
Omer Akgul
Michelle Mazurek
Benoit Seguin
2024
On the Potential of Mediation Chatbots for Mitigating Multiparty Privacy Conflicts - A Wizard-of-Oz Study
Kavous Salehzadeh Niksirat
Diana Korka
Kévin Huguenin
Mauro Cherubini
The 26th ACM Conference On Computer-Supported Cooperative Work And Social Computing (CSCW) (2023) (to appear)
Hark: A Deep Learning System for Navigating Privacy Feedback at Scale
Rishabh Khandelwal
2022 IEEE Symposium on Security and Privacy (SP)
Have Your Text and Use It Too! End-to-End Neural Data-to-Text Generation with Semantic Fidelity
Isabel Groves
Amir Saffari
The 28th International Conference on Computational Linguistics (COLING 2020) (to appear)