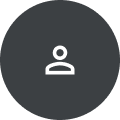
Golnaz Ghiasi
Research Areas
Authored Publications
Sort By
Google
SpineNet: Learning Scale-Permuted Backbone for Recognition and Localization
Xianzhi Du
Tsung-Yi Lin
Yin Cui
Xiaodan Song
CVPR (2020)
Exploring the structure of a real-time, arbitrary neural artistic stylization network
Honglak Lee
Manjunath Kudlur
Jonathon Shlens
Proceedings of the 28th British Machine Vision Conference (BMVC) (2017)