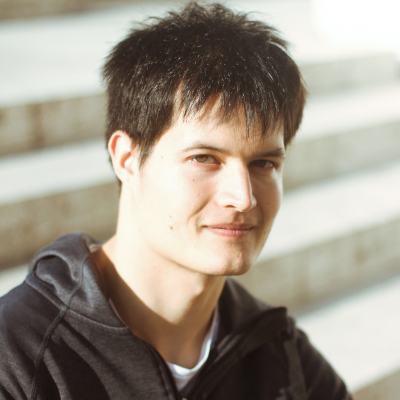
Geza Kovacs
Geza's research focuses on using LLMs for machine translation and LLM multilinguality. Prior to Google, he was Principal Research Scientist at Lilt. He obtained his PhD in Computer Science from Stanford University.
Authored Publications
Sort By
Google
Mitigating metric bias in minimum bayes risk decoding
Proceedings of the Ninth Conference on Machine Translation (2024), pp. 1063-1094
Large Language Models are Few-Shot Health Learners
Daniel McDuff
Isaac Galatzer-Levy
Jake Sunshine
Jiening Zhan
Shun Liao
Paolo Di Achille
Shwetak Patel
ArXiv (2023)