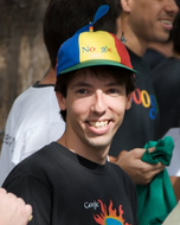
Felipe Goldstein
Research Areas
Authored Publications
Sort By
Recognizing Multimodal Entailment (tutorial at ACL 2021)
Afsaneh Hajiamin Shirazi
Blaž Bratanič
Christina Liu
Gabriel Fedrigo Barcik
Georg Fritz Osang
Jared Frank
Lucas Smaira
Ricardo Abasolo Marino
Roma Patel
Vaiva Imbrasaite
(2021) (to appear)
Preview abstract
In the recent years, a new form of content type has become ubiquitous in the web. These are small and noisy text snippets, created by users of social networks such as Twitter and Facebook. The full interpretation of those microposts by machines impose tremendous challenges, since they strongly rely on context. In this paper we propose a task which is much simpler than full interpretation of microposts: we aim to build classification systems to detect keywords that unambiguously refer to a single dominant concept, even when taken out of context. For example, in the context of this task, apple would be classified as ambiguous whereas microsoft would not. The contribution of this work is twofold. First, we formalize this novel classification task that can be directly applied for extracting information from microposts. Second, we show how high precision classifiers for this problem can be built out of Web data and search engine logs, combining traditional information retrieval metrics, such as inverted document frequency, and new ones derived from search query logs. Finally, we have proposed and evaluated relevant applications for these classifiers, which were able to meet precision ≥ 72% and recall ≥ 56% on unambiguous keyword extraction from microposts. We also compare those results with closely related systems, none of which could outperform those numbers.
View details