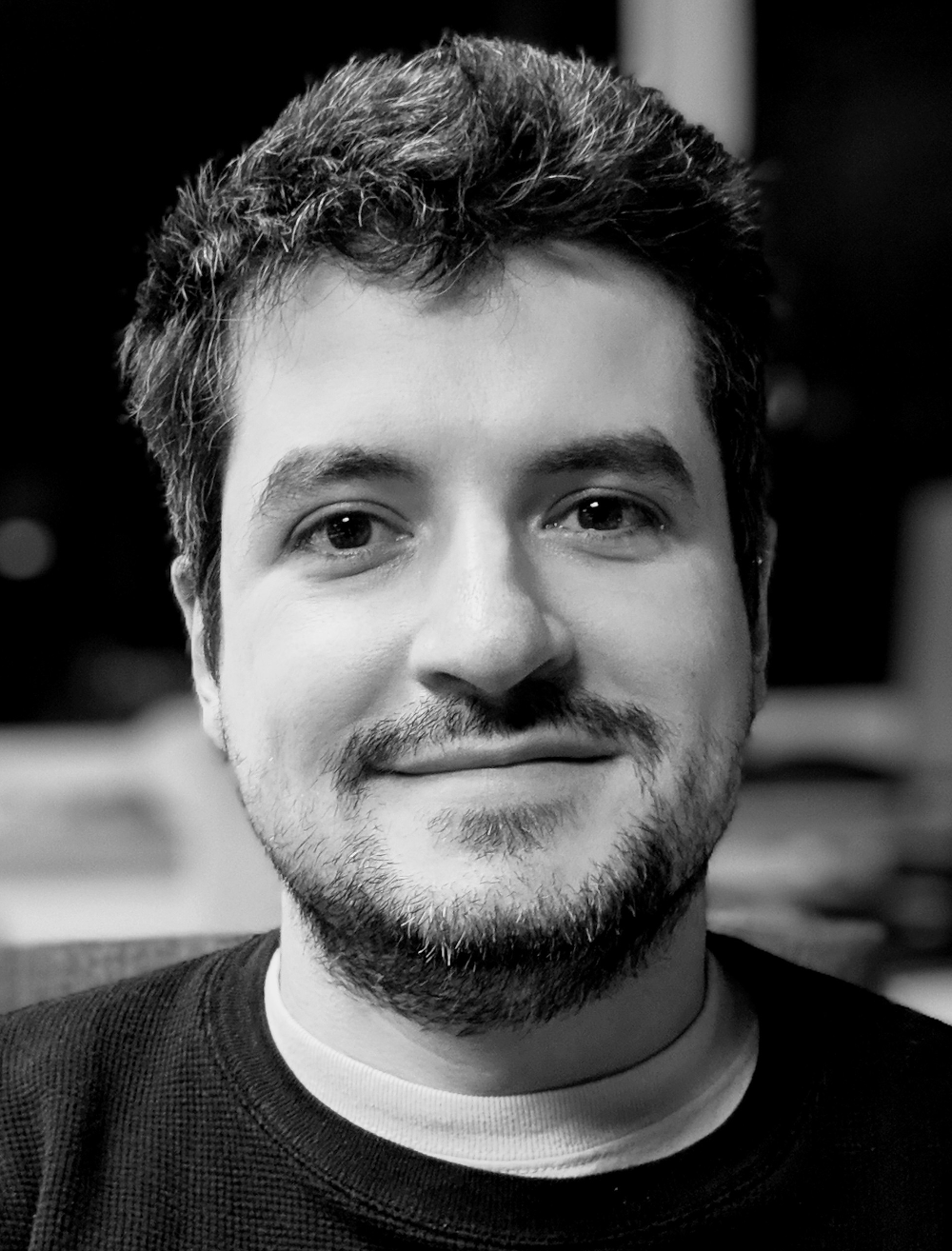
Federico Tombari
Federico Tombari is a Senior Staff Research Scientist and manager at Google, where he leads an applied research team on computer vision and machine learning. He is also a Lecturer (PrivatDozent) at the Technical University of Munich (TUM). He has 200+ peer-reviewed publications in the field of 3D computer vision and machine learning and their applications to robotics, autonomous driving, healthcare and augmented reality. He got his PhD in 2009 from the University of Bologna and his Habilitation from TUM in 2018. In 2018-19 he was co-founder and managing director of Pointu3D, a Munich-based startup on 3D perception for AR and robotics. He regularly serves as Chair and Ass. Editor for international conferences and journals in the field (ECCV18, 3DV19, ICMVA19, 3DV20, IROS20, ICRA20, RA-L among others). He was the recipient, among others, of two Google Faculty Research Awards (in 2015 and 2018), an Amazon Research Award (in 2017), 2 CVPR Outstanding Reviewer Awards (2017, 2018).
Research Areas
Authored Publications
Sort By
Preview abstract
Image-Text pretraining on a web-scale image caption dataset has become the default recipe for open vocabulary classification and retrieval models thanks to the success of CLIP and its variants. Several works have also used CLIP features for dense prediction tasks and have shown the emergence of open-set abilities. However, the contrastive objective only focuses on image and text alignment and does not incentivise image feature learning for dense prediction tasks. In this work, we propose the simple addition of local-to-global correspondence learning by self-distillation as an additional objective for contrastive pre-training to propose SILC. We show that distilling local image features from an EMA teacher model significantly improves model performance on tasks including classification, retrieval, and especially segmentation. We further show that SILC scales better with the same training duration compared to the baselines. Our improved SILC sets a new state-of-the-art for zero-shot classification, few shot classification, image retrieval, zero-shot segmentation, and open vocabulary segmentation.
View details
SceneFun3D: Fine-Grained Functionality and Affordance Understanding in 3D Scenes
Delitzas Alexandros
Ayça Takmaz
Marc Pollefeys
Francis Engelmann
CVPR (2024) (to appear)
Preview abstract
Existing 3D scene understanding methods are heavily focused on 3D semantic and instance segmentation. However, identifying objects and their parts only constitutes an
intermediate step towards a more fine-grained goal, which is effectively interacting with the functional interactive elements (e.g., handles, knobs, buttons) in the scene to accomplish diverse tasks. To this end, we introduce SceneFun3D, a large-scale dataset with more than 14.8k highly accurate interaction annotations for 710 high-resolution real-world 3D indoor scenes. We accompany the annotations with motion parameter information, describing how to interact with these elements, and a diverse set of natural language descriptions of tasks that involve manipulating them in the scene context. To showcase the value of our dataset, we introduce three novel tasks, namely functionality segmentation, task-driven affordance grounding and 3D motion estimation, and adapt existing state-of-the-art methods to tackle them. Our experiments show that solving these tasks in real 3D scenes remains challenging despite recent progress in closed-set and open-set 3D scene understanding methods.
View details
TextMesh: Generation of Realistic 3D Meshes From Text Prompts
Christina Tsalicoglou
Fabian Manhardt
Michael Niemeyer
3DV 2024 (2024)
Preview abstract
The ability to generate highly realistic 2D images from mere text prompts has recently made huge progress in terms of speed and quality, thanks to the advent of image diffusion models. Naturally, the question arises if this can be also achieved in the generation of 3D content from such text prompts. To this end, a new line of methods recently emerged trying to harness diffusion models, trained on 2D images, for supervision of 3D model generation using view dependent prompts. While achieving impressive results, these methods, however, have two major drawbacks. First, rather than commonly used 3D meshes, they instead generate neural radiance fields (NeRFs), making them impractical for most real applications. Second, these approaches tend to produce over-saturated models, giving the output a cartoonish looking effect. Therefore, in this work we propose a novel method for generation of highly realistic-looking 3D meshes. To this end, we extend NeRF to employ an SDF backbone, leading to improved 3D mesh extraction. In addition, we propose a novel way to finetune the mesh texture, removing the effect of high saturation and improving the details of the output 3D mesh.
View details
Preview abstract
We introduce the task of open-vocabulary 3D instance segmentation.
Traditional approaches for 3D instance segmentation largely rely on existing 3D annotated datasets, which are restricted to a closed-set of objects.
This is an important limitation for real-life applications in which an autonomous agent might need to perform tasks guided by novel, open-vocabulary queries related to objects from a wider range of categories.
Recently, open-vocabulary 3D scene understanding methods have emerged to address this problem by learning queryable features per each point in the scene. While such a representation can be directly employed to perform semantic segmentation, existing methods have no notion of object instances.
In this work, we address the open-vocabulary 3D instance segmentation problem, and propose OpenMask3D, which is a zero-shot approach for open-vocabulary 3D instance segmentation.
Guided by predicted class-agnostic 3D instance masks, our model aggregates per-mask features via multi-view fusion of CLIP-based image embeddings.
We conduct experiments and ablation studies on the ScanNet200 dataset to evaluate the performance of OpenMask3D, and provide insights about the task of open-vocabulary 3D instance segmentation. We show that our approach outperforms other open-vocabulary counterparts particularly on the long-tail distribution.
View details
LatentSwap3D: Swapping Latent Codes for Semantic Edits
Enis Simsar
Evin Pınar Örnek
Proceedings of the IEEE/CVF International Conference on Computer Vision (2023)
Preview abstract
3D GANs have the ability to generate latent codes for entire 3D volumes rather than only 2D images. These models offer desirable features like high-quality geometry and multi-view consistency, but, unlike their 2D counterparts, complex semantic image editing tasks for 3D GANs have only been partially explored. To address this problem, we propose LatentSwap3D, a semantic edit approach based on latent space discovery that can be used with any off-the-shelf 3D or 2D GAN model and on any dataset. LatentSwap3D relies on identifying the latent code dimensions corresponding to specific attributes by feature ranking using a random forest classifier. It then performs the edit by swapping the selected dimensions of the image being edited with the ones from an automatically selected reference image. Compared to other latent space control-based edit methods, which were mainly designed for 2D GANs, our method on 3D GANs provides remarkably consistent semantic edits in a disentangled manner and outperforms others both qualitatively and quantitatively. We show results on seven 3D GANs (?-GAN, GIRAFFE, StyleSDF, MVCGAN, EG3D, StyleNeRF, and VolumeGAN) and on five datasets (FFHQ, AFHQ, Cats, MetFaces, and CompCars).
View details
Socratic Models: Composing Zero-Shot Multimodal Reasoning with Language
Andy Zeng
Brian Ichter
Stefan Welker
Aveek Purohit
Michael Ryoo
Pete Florence
arXiv (2022)
Preview abstract
Large pretrained (e.g., "foundation") models exhibit distinct capabilities depending on the domain of data they are trained on. While these domains are generic, they may only barely overlap. For example, visual-language models (VLMs) are trained on Internet-scale image captions, but large language models (LMs) are further trained on Internet-scale text with no images (e.g., spreadsheets, SAT questions, code). As a result, these models store different forms of commonsense knowledge across different domains. In this work, we show that this diversity is symbiotic, and can be leveraged through Socratic Models (SMs): a modular framework in which multiple pretrained models may be composed zero-shot i.e., via multimodal-informed prompting, to exchange information with each other and capture new multimodal capabilities, without requiring finetuning. With minimal engineering, SMs are not only competitive with state-of-the-art zero-shot image captioning and video-to-text retrieval, but also enable new applications such as (i) answering free-form questions about egocentric video, (ii) engaging in multimodal assistive dialogue with people (e.g., for cooking recipes) by interfacing with external APIs and databases (e.g., web search), and (iii) robot perception and planning. Prototypes are available at socraticmodels.github.io
View details
Opportunistic Interfaces for Augmented Reality: Transforming Everyday Objects into Tangible 6DoF Interfaces Using Ad hoc UI
Mathieu Le Goc
Alex Olwal
Shengzhi Wu
Danhang "Danny" Tang
Jun Zhang
David Joseph New Tan
Extended Abstracts of the 2022 CHI Conference on Human Factors in Computing Systems, ACM
Preview abstract
Real-time environmental tracking has become a fundamental capability in modern mobile phones and AR/VR devices. However, it only allows user interfaces to be anchored at a static location. Although fiducial and natural-feature tracking overlays interfaces with specific visual features, they typically require developers to define the pattern before deployment. In this paper, we introduce opportunistic interfaces to grant users complete freedom to summon virtual interfaces on everyday objects via voice commands or tapping gestures. We present the workflow and technical details of Ad hoc UI (AhUI), a prototyping toolkit to empower users to turn everyday objects into opportunistic interfaces on the fly. We showcase a set of demos with real-time tracking, voice activation, 6DoF interactions, and mid-air gestures and prospect the future of opportunistic interfaces.
View details
Preview abstract
Point clouds are often the default choice for many applications as they exhibit more flexibility and efficiency than volumetric data. Nevertheless, their unorganized nature--points are stored in an unordered way--makes them less suited to be processed by deep learning pipelines. In this paper, we propose a method for 3D object completion and classification based on point clouds. We introduce a new way of organizing the extracted features based on their activations, which we name soft pooling. For the decoder stage, we propose regional convolutions, a novel operator aimed at maximizing the global activation entropy. Furthermore, inspired by the local refining procedure in Point Completion Network (PCN), we also propose a patch-deforming operation to simulate deconvolutional operations for point clouds. This paper proves that our regional activation can be incorporated in many point cloud architectures like AtlasNet and PCN, leading to better performance for geometric completion. We evaluate our approach on different 3D tasks such as object completion and classification, achieving state-of-the-art accuracy.
View details
A Divide et Impera Approach for 3D Shape Reconstruction from Multiple Views
Riccardo Spezialetti
David Joseph New Tan
Keisuke Tateno
International Virtual Conference on 3D Vision (2020) (to appear)
Preview abstract
Estimating the 3D shape of an object from a single or multiple images has gained popularity thanks to the recent breakthroughs powered by deep learning.
Most approaches regress the full object shape in a canonical pose, possibly extrapolating the occluded parts based on the learned priors.
However, their viewpoint invariant technique often discards the unique structures visible from the input images.
In contrast, this paper proposes to rely on viewpoint variant reconstructions by merging the visible information from the given views.
Our approach is divided into three steps.
Starting from the sparse views of the object, we first align them into a common coordinate system by estimating the relative pose between all the pairs.
Then, inspired by the traditional voxel carving, we generate an occupancy grid of the object taken from the silhouette on the images and their relative poses.
Finally, we refine the initial reconstruction to build a clean 3D model which preserves the details from each viewpoint.
To validate the proposed method, we perform a comprehensive evaluation on the ShapeNet reference benchmark in terms of relative pose estimation and 3D shape reconstruction.
View details