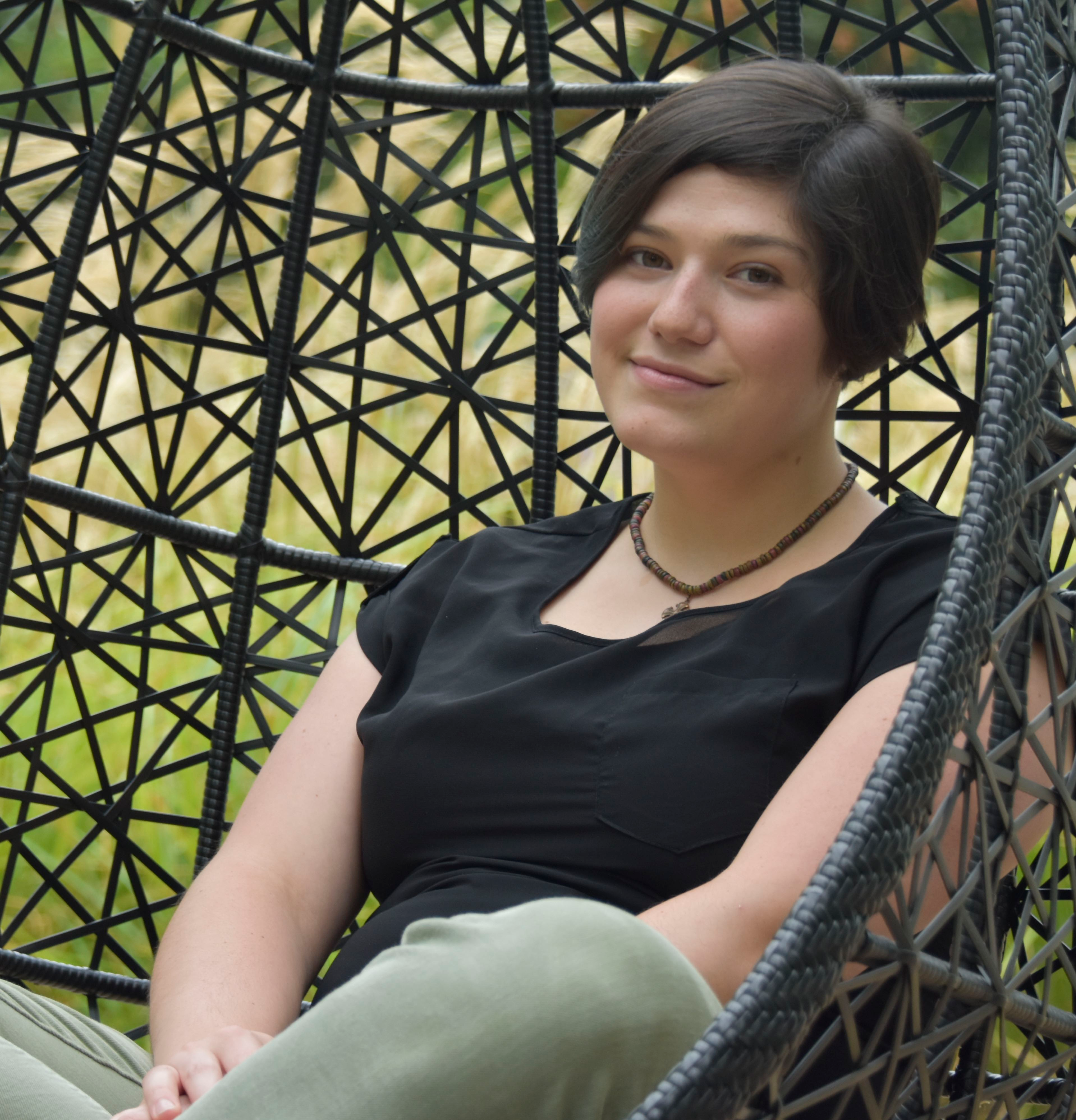
Emily Johnston
I work on static analysis tools for Java. Before coming to Google, I studied Computer Science and researched Evolutionary Computation at Carleton College. My main research interests are in compilers, programming languages, and static analysis, with an emphasis on usability.
Research Areas
Authored Publications
Sort By
Google
DeepDelta: Learning to Repair Compilation Errors
Ali Mesbah
Andrew Rice
Nick Glorioso
Eddie Aftandilian
Proceedings of the 2019 27th ACM Joint European Software Engineering Conference and Symposium on the Foundations of Software Engineering (ESEC/FSE) (2019)
Analyzing and Repairing Compilation Errors
Ali Mesbah
Andrew Rice
Eddie Aftandilian
Nick Glorioso
International Conference on Software Engineering (ICSE), poster track (2019) (to appear)
Detecting argument selection defects
Andrew Rice
Eddie Aftandilian
Michael Pradel
Yulissa Arroyo-Paredes
SPLASH 2017 OOPSLA