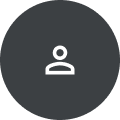
Denys Shabalin
Denys has been at google since 2019. His research interests include interaction of programming languages, compilers and high-performance computing.
Authored Publications
Sort By
Mutable Value Semantics
Dimitri Racordon
Dave Abrahams
Brennan Saeta
Journal of Object Technology, 21 (2022)
Preview abstract
Mutable value semantics is a programming discipline that upholds the independence of values to support local reasoning. In the discipline’s strictest form, references become second-class citizens: they are only created implicitly, at function boundaries, and cannot be stored in variables or object fields. Hence, variables can never share mutable state. Unlike pure functional programming, however, mutable value semantics allows part-wise in-place mutation, thereby eliminating the memory traffic usually associated with functional updates of immutable data.
This paper presents implementation strategies for compiling programs with mutable value semantics into efficient native code. We study Swift, a programming language based on that discipline, through the lens of a core language that strips some of Swift’s features to focus on the semantics of its value types. The strategies that we introduce leverage the inherent properties of mutable value semantics to unlock aggressive optimizations. Fixed-size values are allocated on the stack, thereby enabling numerous off-the-shelf compiler optimizations, while dynamically sized containers use copy-on-write to mitigate copying costs.
View details
LazyTensor: combining eager execution with domain-specific compilers
Alex Suhan
Davide Libenzi
Ailing Zhang
Parker Schuh
Brennan Saeta
Jie Young Sohn
Google (2021)
Preview abstract
Domain-specific optimizing compilers have demonstrated significant performance and portability benefits, but require programs to be represented in their specialized IRs. Existing frontends to these compilers suffer from the "language subset problem" where some host language features are unsupported in the subset of the user's program that interacts with the domain-specific compiler. By contrast, define-by-run ML frameworks-colloquially called "eager" mode-are popular due to their ease of use and expressivity, where the full power of the host programming language can be used. LazyTensor is a technique to target domain specific compilers without sacrificing define-by-run ergonomics. Initially developed to support PyTorch on Cloud TPUs, the technique, along with a substantially shared implementation, has been used by Swift for TensorFlow across CPUs, GPUs, and TPUs, demonstrating the generality of the approach across (1) Tensor implementations, (2) hardware accelerators, and (3) programming languages.
View details
Swift for TensorFlow: A portable, flexible platform for deep learning
Brennan Saeta
Marc Rasi
Brad Larson
Xihui Wu
Parker Schuh
Saleem Abdulrasool
Aleksandr Efremov
Dave Abrahams
Chris Lattner
Richard Wei
MLSys (2021)
Preview abstract
Swift for TensorFlow is a deep learning platform that scales from mobile devices to clusters of hardware accelerators in data centers. It combines a language-integrated automatic differentiation system and multiple Tensor implementations within a modern ahead-of-time compiled language oriented around mutable value semantics. The resulting platform has been validated through use in over 30 deep learning models and has been employed across data center and mobile applications.
View details