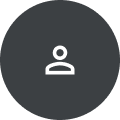
Cristian Sminchisescu
Cristian Sminchisescu is a Research Scientist and Engineering Manager at Google DeepMind, and a Professor at Lund University. He has obtained a doctorate in computer science and applied mathematics with focus on imaging, vision and robotics at INRIA, under an Eiffel excellence fellowship of the French Ministry of Foreign Affairs, and has done postdoctoral research in the Artificial intelligence Laboratory at the University of Toronto. He has held a Professor equivalent title at the Romanian Academy and a Professor rank, status appointment at Toronto, and has advised research at both institutions. During 2004-07, he was a faculty member at the Toyota Technological Institute at the University of Chicago, and later on the Faculty of the Institute for Numerical Simulation in the Mathematics Department at Bonn University. Over time, his work has been funded by the US National Science Foundation, the Romanian Science Foundation, the German Science Foundation, the Swedish Science Foundation, the European Commission under a Marie Curie Excellence Grant, and the European Research Council under an ERC Consolidator Grant. Cristian Sminchisescu's research interests are in the area of computer vision (3d human sensing, reconstruction and recognition), machine learning (optimization and sampling algorithms, kernel methods and deep learning), and multi-modal foundation agents. The visual recognition methodology developed in his group was a winner of the PASCAL VOC object segmentation and labeling challenge during 2009-12, as well as the Reconstruction Meets Recognition Challenge (RMRC) 2013-14. His work on deep learning of graph matching has received the best paper award honorable mention at CVPR 2018. Cristian Sminchisescu regularly serves as an Area Chair for computer vision and machine learning conferences (CVPR, ECCV, ICCV, AAAI, neurIPS). He has been an Associate Editor of IEEE Transactions for Pattern Analysis and Machine Intelligence (PAMI) and the International Journal of Computer Vision (IJCV), was a Program Chair for ECCV 2018, and is General Chair for CVPR 2025 and ECCV 2028.
Authored Publications
Sort By
Preview abstract
We present PhoMoH, a neural network methodology to construct generative models of photo-realistic 3D geometry and appearance of human heads including hair, beards, an oral cavity, and clothing. In contrast to prior work, PhoMoH models the human head using neural fields, thus supporting complex topology. Instead of learning a head model from scratch, we propose to augment an existing expressive head model with new features. Concretely, we learn a highly detailed geometry network layered on top of a mid-resolution head model together with a detailed, local geometry-aware, and disentangled color field. Our proposed architecture allows us to learn photo-realistic human head models from relatively little data. The learned generative geometry and appearance networks can be sampled individually and enable the creation of diverse and realistic human heads. Extensive experiments validate our method qualitatively and across different metrics.
View details
SPHEAR: Spherical Head Registration for Complete Statistical 3D Modeling
Andrei Zanfir
Teodor Szente
Mihai Zanfir
International Conference on 3D Vision (2024)
Preview abstract
We present SPHEAR, an accurate, differentiable parametric statistical 3D human head model, enabled by a novel 3D registration method based on spherical embeddings. We shift the paradigm away from the classical Non-Rigid Registration methods, which operate under various surface priors, increasing reconstruction fidelity and minimizing required human intervention. Additionally, SPHEAR is a complete model that allows not only to sample diverse synthetic head shapes and facial expressions, but also gaze directions, high-resolution color textures, surface normal maps, and hair cuts represented in detail, as strands. SPHEAR can be used for automatic realistic visual data generation, semantic annotation, and general reconstruction tasks. Compared to state-of-the-art approaches, our components are fast and memory efficient, and experiments support the validity of our design choices and the accuracy of registration, reconstruction and generation techniques.
View details
Score Distillation Sampling with Learned Manifold Corrective
European Conference on Computer Vision (ECCV) (2024)
Preview abstract
Score Distillation Sampling (SDS) is a recent but already widely popular method that relies on an image diffusion model to control optimization problems using text prompts. In this paper, we conduct an in-depth analysis of the SDS loss function, identify an inherent problem with its formulation, and propose a surprisingly easy but effective fix. Specifically, we decompose the loss into different factors and isolate the component responsible for noisy gradients. In the original formulation, high text guidance is used to account for the noise, leading to unwanted side effects such as oversaturation or repeated detail. Instead, we train a shallow network mimicking the timestep-dependent frequency bias of the image diffusion model in order to effectively factor it out. We demonstrate the versatility and the effectiveness of our novel loss formulation through qualitative and quantitative experiments, including optimization-based image synthesis and editing, zero-shot image translation network training, and text-to-3D synthesis.
View details
Instant 3D Human Avatar Generation using Image Diffusion Models
Enric Corona
European Conference on Computer Vision (ECCV) (2024)
Preview abstract
We present AvatarPopUp, a method for fast, high quality 3D human avatar generation from different input modalities, such as images and text prompts and with control over the generated pose and shape. The common theme is the use of diffusion-based image generation networks that are specialized for each particular task, followed by a 3D lifting network. We purposefully decouple the generation from the 3D modeling which allow us to leverage powerful image synthesis priors, trained on billions of text-image pairs. We fine-tune latent diffusion networks with additional image conditioning for image generation and back-view prediction, and to support qualitatively different multiple 3D hypotheses. Our partial fine-tuning approach allows to adapt the networks for each task without inducing catastrophic forgetting. In our experiments, we demonstrate that our method produces accurate, high-quality 3D avatars with diverse appearance that respect the multimodal text, image, and body control signals. Our approach can produce a 3D model in as few as 2 seconds, a four orders of magnitude speedup w.r.t. the vast majority of existing methods, most of which solve only a subset of our tasks, and with fewer controls. AvatarPopUp enables applications that require the controlled 3D generation of human avatars at scale.
View details
DiffHuman: Probabilistic Photorealistic 3D Reconstruction of Humans
Akash Sengupta
Enric Corona
Andrei Zanfir
Proceedings of the IEEE/CVF Conference on Computer Vision and Pattern Recognition (CVPR) (2024)
Preview abstract
We present DiffHuman, a probabilistic method for photorealistic 3D human reconstruction from a single RGB image. Despite the ill-posed nature of this problem, most methods are deterministic and output a single solution, often resulting in a lack of geometric detail and blurriness in unseen or uncertain regions. In contrast, DiffHuman predicts a distribution over 3D reconstructions conditioned on an image, which allows us to sample multiple detailed 3D avatars that are consistent with the input image. DiffHuman is implemented as a conditional diffusion model that denoises partial observations of an underlying pixel-aligned 3D representation. In testing, we can sample a 3D shape by iteratively denoising renderings of the predicted intermediate representation. Further, we introduce an additional generator neural network that approximates rendering with considerably reduced runtime (55x speed up), resulting in a novel dual-branch diffusion framework. We evaluate the effectiveness of our approach through various experiments. Our method can produce diverse, more detailed reconstructions for the parts of the person not observed in the image, and has competitive performance for the surface reconstruction of visible parts.
View details
DreamHuman: Animatable 3D Avatars from Text
Andrei Zanfir
Mihai Fieraru
Advances in Neural Information Processing Systems (2023)
Preview abstract
We present DreamHuman, a method to generate realistic animatable 3D human avatar models solely from textual descriptions. Recent text-to-3D methods have made considerable strides in generation, but are still lacking in important aspects. Control and often spatial resolution remain limited, existing methods produce fixed rather than animated 3D human models, and anthropometric consistency for complex structures like people remains a challenge. DreamHuman connects large text-to-image synthesis models, neural radiance fields, and statistical human body models in a novel modeling and optimization framework. This makes it possible to generate dynamic 3D human avatars with high-quality textures and learned, instance-specific, surface deformations. We demonstrate that our method is capable to generate a wide variety of animatable, realistic 3D human models from text. Our 3D models have diverse appearance, clothing, skin tones and body shapes, and significantly outperform both generic text-to-3D approaches and previous text-based 3D avatar generators in visual fidelity.
View details
Structured 3D Features for Reconstructing Controllable Avatars
Enric Corona
Mihai Zanfir
Andrei Zanfir
Proceedings of the IEEE/CVF Conference on Computer Vision and Pattern Recognition (CVPR) (2023)
Preview abstract
We introduce Structured 3D Features, a model based on a novel implicit 3D representation that pools pixel-aligned image features onto dense 3D points sampled from a parametric, statistical human mesh surface. The 3D points have associated semantics and can move freely in 3D space. This allows for optimal coverage of the person of interest, beyond just the body shape, which in turn, additionally helps modeling accessories, hair, and loose clothing. Owing to this, we present a complete 3D transformer-based attention framework which, given a single image of a person in an unconstrained pose, generates an animatable 3D reconstruction with albedo and illumination decomposition, as a result of a single end-to-end model, trained semi-supervised, and with no additional postprocessing. We show that our S3F
model surpasses the previous state-of-the-art on various tasks, including monocular 3D reconstruction, as well as albedo & shading estimation. Moreover, we show that the proposed methodology allows novel view synthesis, relighting, and re-posing the reconstruction, and can naturally be extended to handle multiple input images (e.g. different views of a person, or the same view, in different poses, in video). Finally, we demonstrate the editing capabilities of our model for 3D virtual try-on applications.
View details
Photorealistic Monocular 3D Reconstruction of Humans Wearing Clothing
Mihai Zanfir
Proceedings of the IEEE/CVF Conference on Computer Vision and Pattern Recognition (CVPR), IEEE (2022)
Preview abstract
We present PHORHUM, a novel, end-to-end trainable, deep neural network methodology for photorealistic 3D human reconstruction given just a monocular RGB image. Our pixel-aligned method estimates detailed 3D geometry and, for the first time, the unshaded surface color together with the scene illumination. Observing that 3D supervision alone is not sufficient for high fidelity color reconstruction, we introduce patch-based rendering losses that enable reliable color reconstruction on visible parts of the human, and detailed and plausible color estimation for the non-visible parts. Moreover, our method specifically addresses methodological and practical limitations of prior work in terms of representing geometry, albedo, and illumination effects, in an end-to-end model where factors can be effectively disentangled. In extensive experiments, we demonstrate the versatility and robustness of our approach. Our state-of-the-art results validate the method qualitatively and for different metrics, for both geometric and color reconstruction.
View details
THUNDR: Transformer-based 3D HUmaN Reconstruction with Markers
Mihai Zanfir
Andrei Zanfir
Proceedings of the IEEE/CVF International Conference on Computer Vision (2021)
Preview abstract
We present THUNDR, a transformer-based deep neural network methodology to reconstruct the 3D pose and shape of people, given monocular RGB images. Key to our methodology is an intermediate 3D marker representation, where we aim to combine the predictive power of model-free output architectures and the regularizing, anthropometrically-preserving properties of a statistical human surface models like GHUM—a recently introduced, expressive full body statistical 3d human model, trained end-to-end. Our novel transformer-based prediction pipeline can focus on image regions relevant to the task, supports self-supervised regimes, and ensures that solutions are consistent with human anthropometry. We show state-of-the-art results on Human3.6M and 3DPW, for both the fully-supervised and the self-supervised models, for the task of inferring 3D human shape, joint positions, and global translation. Moreover, we observe very solid 3d reconstruction performance for difficult human poses collected in the wild. Models will be made available for research.
View details
Calibration of Neural Networks using Splines
Kartik Gupta
Amir Rahimi
Thalaiyasingam Ajanthan
Richard Ian Hartley
International Conference on Learning Representations (ICLR) (2021)
Preview abstract
Calibrating neural networks is of utmost importance when employing them in safety-critical applications where the downstream decision making depends on the predicted probabilities. Measuring calibration error amounts to comparing two empirical distributions. In this work, we introduce a binning-free calibration measure inspired by the classical Kolmogorov-Smirnov (KS) statistical test in which the main idea is to compare the respective cumulative probability distributions. From this, by approximating the empirical cumulative distribution using a differentiable function via splines, we obtain a recalibration function, which maps the network outputs to actual (calibrated) class assignment probabilities. The spline-fitting is performed using a held-out calibration set and the obtained recalibration function is evaluated on an unseen test set. We tested our method against existing calibration approaches on various image classification datasets and our spline-based recalibration approach consistently outperforms existing methods on KS error as well as other commonly used calibration measures.
View details