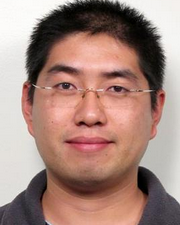
Xiance Si
Authored Publications
Sort By
Preview abstract
We present a large-scale dataset for the task of rewriting an ill-formed natural language question to a well-formed one. Our multi-domain question rewriting (MQR) dataset is constructed from human contributed Stack Exchange question edit histories. The dataset contains 427,719 question pairs which come from 303 domains. We provide human annotations for a subset of the dataset as a quality estimate. When moving from ill-formed to well-formed questions, the question quality improves by an average of 45 points across three aspects. We train sequence-to-sequence neural models on the constructed dataset and obtain an improvement of 13.2% in BLEU-4 over baseline methods built from other data resources.
View details
Deceptive Answer Prediction with User Preference Graph
Preview
Yang Gao
Shuchang Zhou
Decheng Dai
The 51st Annual Meeting of the Association for Computational Linguistics (ACL 2013) (to appear)
Entity Disambiguation with Freebase
Preview
Zhicheng Zheng
Edward Y. Chang
Xiaoyan Zhu
The 2012 IEEE/WIC/ACM International Conference on Web Intelligence (WI'2012) (to appear)
A Data-Driven Approach to Question Subjectivity Identification in Community Question Answering
Preview
Tom Chao Zhou
Edward Y.
Irwin King
Michael R. Lyu
Proceedings of the 26th AAAI Conference on Artificial Intelligence (AAAI-12) (2012)
K2Q: Generating Natural Language Questions from Keywords with User Refinements
Zhicheng Zheng
Edward Y. Chang
Xiaoyan Zhu
Proceedings of the 5th International Joint Conference on Natural Language Processing, ACL (2011), 947–955
Preview abstract
Garbage in and garbage out. A Q&A system must receive a well formulated question that matches the user’s intent or she
has no chance to receive satisfactory answers. In this paper, we propose a keywords to questions (K2Q) system to assist a user to articulate and refine questions.
K2Q generates candidate questions and refinement words from a set of input keywords. After specifying some initial keywords, a user receives a list of candidate questions as well as a list of refinement words. The user can then select a satisfactory question, or select a refinement word
to generate a new list of candidate questions and refinement words. We propose a User Inquiry Intent (UII) model to de-
scribe the joint generation process of keywords and questions for ranking questions, suggesting refinement words, and generating questions that may not have previously
appeared. Empirical study shows UII to be useful and effective for the K2Q task.
View details
Question Identification on Twitter, Accepted by CIKM 2011
Baichuan Li
Michael R. Lyu
Irwin King
Edward Y. Chang
Proceedings of the 20th ACM international conference on Information and knowledge management, ACM, New York, NY, USA (2011)
Preview abstract
In this paper, we investigate the novel problem of auto-
matic question identification in the microblog environment.
It contains two steps: detecting tweets that contain ques-
tions (we call them “interrogative tweets”) and extracting
the tweets which really seek information or ask for help (so
called “qweets”) from interrogative tweets. To detect inter-
rogative tweets, both traditional rule-based approach and
state-of-the-art learning-based method are employed. To
extract qweets, context features like short urls and Tweet-
specific features like Retweets are elaborately selected for
classification. We conduct an empirical study with sampled
one hour’s English tweets and report our experimental re-
sults for question identification on Twitter.
View details
Confucius and Its Intelligent Disciples: Integrating Social with Search
Edward Y. Chang
Zoltan Gyongyi
Maosong Sun
Proceedings of VLDB 2010, 36th International Conference on Very Large Data Bases, VLDB Endowment, pp. 1505-1516
Preview abstract
Q&A sites continue to flourish as a large number of users rely on them as useful substitutes for incomplete or missing search results. In this paper, we present our experience with developing Confucius, a Google Q&A service launched in 21 countries and four languages by the end of 2009. Confucius employs six data mining subroutines to harness synergy between web search and social networks. We present these subroutines’ design goals, algorithms, and their effects on service quality. We also describe techniques for and experience with scaling the subroutines to mine massive data sets.
View details