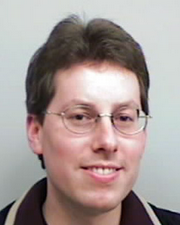
Klaus Macherey
Klaus Macherey joined Google in 2006 as a research scientist, where he works in the machine translation group. He has been working on natural language processing since 1996.
Klaus was a Research Assistant at RWTH Aachen University from 1999 to 2005. His main research interests are in statistical machine translation and automatic speech recognition with the focus on natural language understanding and spoken dialogue systems, natural language processing, statistical pattern recognition, and machine learning.
He received a PhD in Computer Science from RWTH Aachen University, Germany, in 2009 and his Diploma Degree in Computer Science from RWTH Aachen University in 1999 with a major in statistical pattern recognition and a minor in physical chemistry and thermodynamics.
Research Areas
Authored Publications
Sort By
Building Machine Translation Systems for the Next Thousand Languages
Julia Kreutzer
Mengmeng Niu
Pallavi Nikhil Baljekar
Xavier Garcia
Maxim Krikun
Pidong Wang
Apu Shah
Macduff Richard Hughes
Google Research (2022)
Google's Neural Machine Translation System: Bridging the Gap between Human and Machine Translation
Mike Schuster
Mohammad Norouzi
Maxim Krikun
Qin Gao
Apurva Shah
Xiaobing Liu
Łukasz Kaiser
Stephan Gouws
Taku Kudo
Keith Stevens
George Kurian
Nishant Patil
Wei Wang
Jason Smith
Alex Rudnick
Macduff Hughes
CoRR, abs/1609.08144 (2016)
Preview abstract
Neural Machine Translation (NMT) is an end-to-end learning approach for automated translation, with the potential to overcome many of the weaknesses of conventional phrase-based translation systems. Unfortunately, NMT systems are known to be computationally expensive both in training and in translation inference. Also, most NMT systems have difficulty with rare words. These issues have hindered NMT's use in practical deployments and services, where both accuracy and speed are essential. In this work, we present GNMT, Google's Neural Machine Translation system, which attempts to address many of these issues. Our model consists of a deep LSTM network with 8 encoder and 8 decoder layers using attention and residual connections. To improve parallelism and therefore decrease training time, our attention mechanism connects the bottom layer of the decoder to the top layer of the encoder. To accelerate the final translation speed, we employ low-precision arithmetic during inference computations. To improve handling of rare words, we divide words into a limited set of common sub-word units ("wordpieces") for both input and output. This method provides a good balance between the flexibility of "character"-delimited models and the efficiency of "word"-delimited models, naturally handles translation of rare words, and ultimately improves the overall accuracy of the system. Our beam search technique employs a length-normalization procedure and uses a coverage penalty, which encourages generation of an output sentence that is most likely to cover all the words in the source sentence. On the WMT'14 English-to-French and English-to-German benchmarks, GNMT achieves competitive results to state-of-the-art. Using a human side-by-side evaluation on a set of isolated simple sentences, it reduces translation errors by an average of 60% compared to Google's phrase-based production system.
View details
Preview abstract
Unsupervised word alignment is most often modeled as a Markov process that generates a sentence f conditioned on its translation e. A similar model generating e from f will make different alignment predictions. Statistical machine translation systems combine the predictions of two directional models, typically using heuristic combination procedures like grow-diag-final. This paper presents a graphical model that embeds two directional aligners into a single model. Inference can be performed via dual decomposition, which reuses the efficient inference algorithms of the directional models. Our bidirectional model enforces a one-to-one phrase constraint while accounting for the uncertainty in the underlying directional models. The resulting alignments improve upon baseline combination heuristics in word-level and phrase-level evaluations.
View details
Preview abstract
Translating compounds is an important problem in machine translation. Since many compounds have not been observed during training, they pose a challenge for translation systems. Previous decompounding methods have
often been restricted to a small set of languages as they cannot deal with more complex compound forming processes. We present a novel and unsupervised method to learn the
compound parts and morphological operations needed to split compounds into their compound parts. The method uses a bilingual corpus to learn the morphological operations
required to split a compound into its parts. Furthermore, monolingual corpora are used to learn and filter the set of compound part candidates. We evaluate our method within a machine translation task and show significant improvements for various languages to show the versatility of the approach.
View details