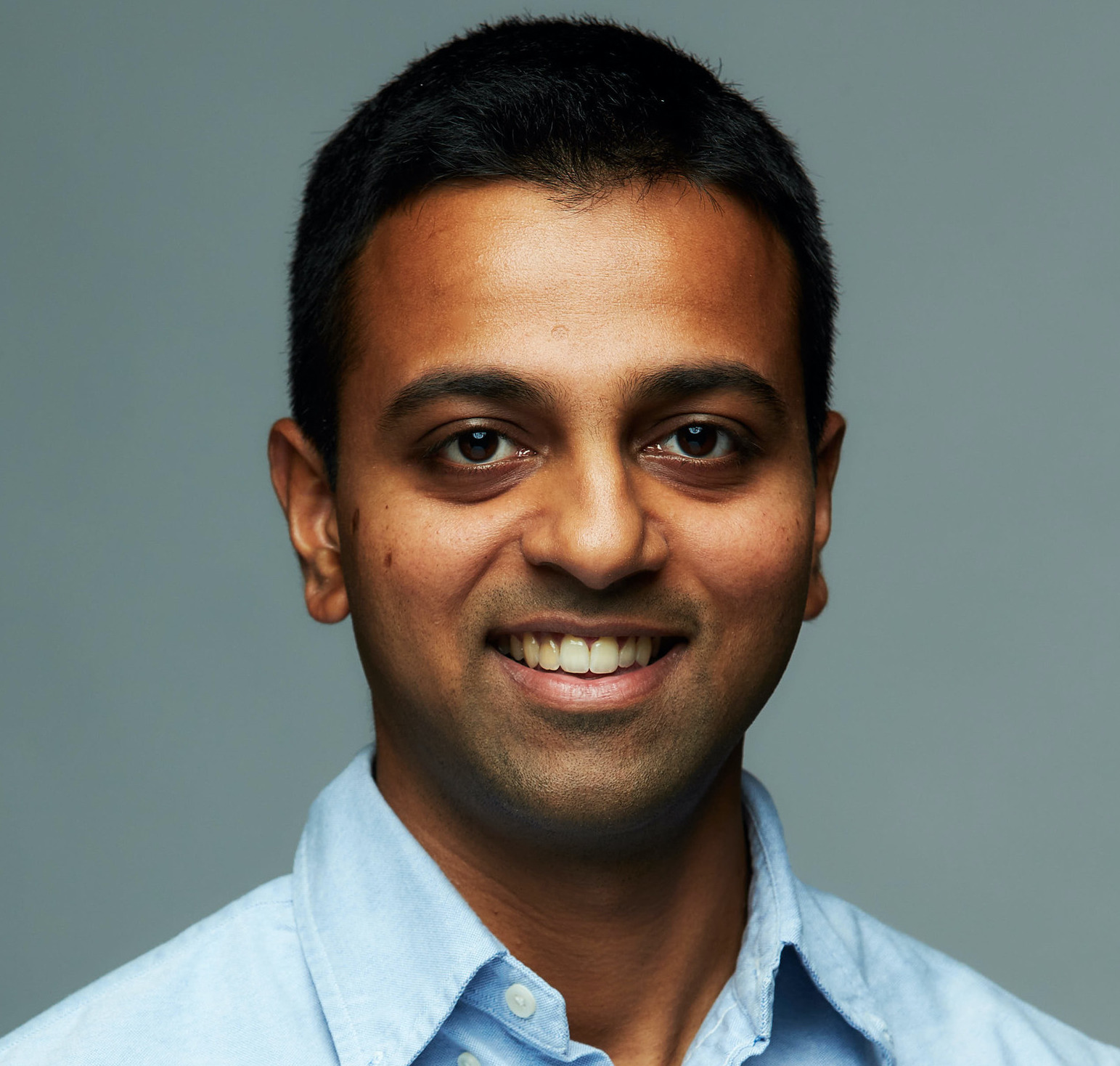
Arjun Gopalan
I am a software engineer at Google Research. My areas of interest include graph-based machine learning, label propagation, and data mining. I currently work on Google's large scale semi-supervised machine learning platform and on Neural Structured Learning in TensorFlow.
Prior to Google, I worked on developing enterprise storage technologies at Tintri for close to 4 years. While at Tintri, I was one of the principal contributors to the design and implementation of logical synchronous replication with automatic transparent failover. A paper on Logical Synchronous Replication appeared in FAST’18.
I completed my Masters in Computer Science with a distinction in research at Stanford University in 2014. At Stanford, I was part of the Platform Lab working with Dr. John Ousterhout on RAMCloud, a low latency DRAM-based distributed data center storage system. A paper on RAMCloud appeared in TOCS’15. My Master’s thesis was on managing objects and secondary indexes in RAMCloud, which was part of a larger effort to design and implement scalable low-latency secondary indices (SLIK) in RAMCloud. A paper on SLIK appeared in ATC'16.