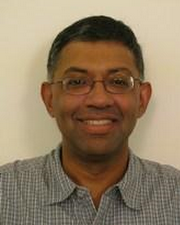
Arif Merchant
Arif Merchant is a Research Scientist with the Storage Analytics group at Google, where he studies interactions between components of the storage stack. Prior to this, he was with HP Labs, where he worked on storage QoS, distributed storage systems, and stochastic models of storage. He holds the B.Tech. degree from IIT Bombay and the Ph.D. in Computer Science from Stanford University. He is an ACM Distinguished Scientist.
Authored Publications
Sort By
Google
Practical Design Considerations for Wide Locally Recoverable Codes (LRCs)
Shashwat Silas
Dave Clausen
File and Storage Technologies (FAST), USENIX (2023)
CacheSack: Admission Optimization for Datacenter Flash Caches
Homer Wolfmeister
Mustafa Uysal
Seth Bradley Pollen
Tzu-Wei Yang
2022 USENIX Annual Technical Conference (2022) (to appear)
Tiger: disk-adaptive redundancy without placement restrictions
Francisco Maturana
Sanjith Athlur
Rashmi KV
Gregory R. Ganger
Tiger: disk-adaptive redundancy without placement restrictions (2022)
Slicer: Auto-Sharding for Datacenter Applications
Atul Adya
Jon Howell
Jeremy Elson
Colin Meek
Vishesh Khemani
Stefan Fulger
Pan Gu
Lakshminath Bhuvanagiri
Jason Hunter
Roberto Peon
Alexander Shraer
Kfir Lev-Ari
OSDI 2016 (2016)
Take me to your leader! Online Optimization of Distributed Storage Configurations
Artyom Sharov
Alexander Shraer
Murray Stokely
Proceedings of the 41st International Conference on Very Large Data Bases, VLDB Endowment (2015), pp. 1490-1501
Poster Paper: Automatic Reconfiguration of Distributed Storage
Artyom Sharov
Alexander Shraer
Murray Stokely
The 12th International Conference on Autonomic Computing, IEEE (2015), pp. 133-134
Janus: Optimal Flash Provisioning for Cloud Storage Workloads
Murray Stokely
Muhammad Waliji
Francois Labelle
Xudong Shi
Eric Schrock
Proceedings of the USENIX Annual Technical Conference, USENIX, Advanced Computing System Association, 2560 Ninth Street, Suite 215, Berkeley, CA 94710, USA (2013), pp. 91-102
Projecting Disk Usage Based on Historical Trends in a Cloud Environment
Murray Stokely
Amaan Mehrabian
Francois Labelle
ScienceCloud 2012 Proceedings of the 3rd International Workshop on Scientific Cloud Computing, ACM, pp. 63-70