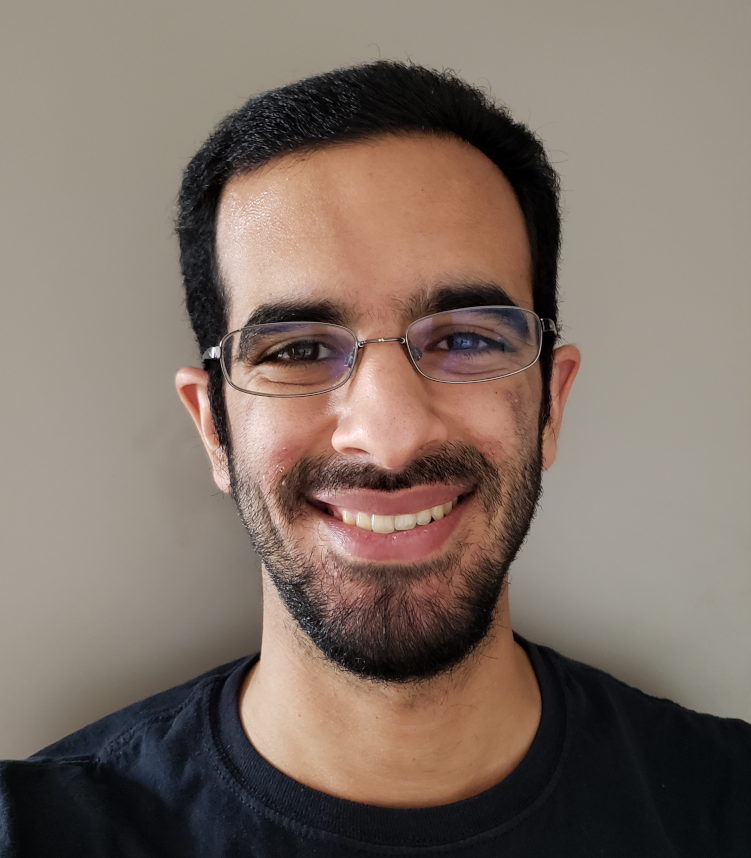
Ahmed Omran
I'm an AI Resident at Google Zurich, working on neural-network based audio models for sound compression and processing. In a previous life, I graduated with a degree in Engineering Physics from Technical University of Munich in 2011 and did my PhD work at the Max-Planck-Institute of Quantum Optics in Garching, building a quantum simulator for electronic lattice models using ultracold gases of atoms. After graduating in 2016. I then spent a four-year postdoc period at Harvard University, developing a platform for experimental quantum simulations using cold atoms trapped in optical tweezers.
Research Areas
Authored Publications
Sort By
Google
Disentangling speech from surroundings with neural embeddings
Malcolm Slaney
Marco Tagliasacchi
Neil Zeghidour
Zalán Borsos
ICASSP 2023 (2023)
SoundStream: An End-to-End Neural Audio Codec
Neil Zeghidour
Alejandro Luebs
Marco Tagliasacchi
Transactions on Audio, Speech and Language Processing (2021)