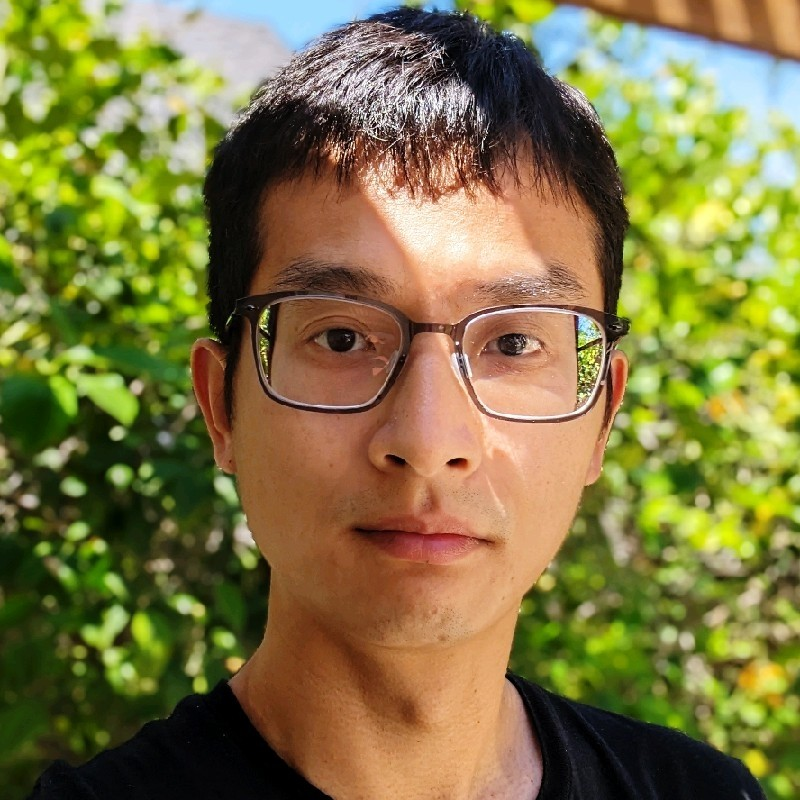
Xiaoran "Van" Fan
Van is a Senior Research Scientist at Google. He is working on building novel health and safety features for the consumer hardware lines at Google (Pixel phone, watch, buds). Van is an experimentalist and system builder. He is enthusiastic about real-world-experiment-driven research.
Authored Publications
Sort By
See Through Vehicles: Fully Occluded Vehicle Detection with Millimeter Wave Radar
Chenming He
Chengzhen Meng
Beibei Wang
Chunwang He
Yubo Yan
Yanyong Zhang
MobiCom 2024: The 30th Annual International Conference On Mobile Computing And Networking
Preview abstract
A crucial task in autonomous driving is to continuously detect nearby vehicles. Problems thus arise when a vehicle is occluded and becomes “unseeable”, which may lead to accidents. In this study, we develop mmOVD, a system that can detect fully occluded vehicles by involving millimeter-wave radars to capture the ground-reflected signals passing beneath the blocking vehicle’s chassis. The foremost challenge here is coping with ghost points caused by frequent multi-path reflections, which highly resemble the true points. We devise a set of features that can efficiently distinguish the ghost points by exploiting the neighbor points’ spatial and velocity distributions. We also design a cumulative clustering algorithm to effectively aggregate the unstable ground reflected radar points over consecutive frames to derive the bounding boxes of the vehicles.
We have evaluated mmOVD in both controlled environments and real-world environments. In an underground garage and two campus roads, we conducted controlled experiments in 56 scenes with 8 vehicles, including a minibus and a motorcycle. Our system accurately detects occluded vehicles for the first time, with a 91.1% F1 score for occluded vehicle detection and a 100% success rate for occlusion event detection. More importantly, we drove 324km on crowded roads at a speed up to 70km per hour and show we could achieve an occlusion detection success rate of 92% and a low false alarm rate of 4% with only 10% of the training data in complex real-world environments.
View details
Leveraging Foundation Models for Zero-Shot IoT Sensing
Dinghao Xue
Tao Chen
Qun Song
Guohao Lan
ECAI 2024: The 27th European Conference on Artificial Intellegence (to appear)
Preview abstract
Zero-shot learning (ZSL) aims to classify data of unseen classes with the help of semantic information. Foundation models (FMs) trained on web-scale data have shown impressive ZSL capability in natural language processing and visual understanding. However, leveraging FMs' generalized knowledge for zero-shot Internet of Things (IoT) sensing using signals such as mmWave, IMU, and Wi-Fi has not been fully investigated. In this work, we align the IoT data embeddings with the semantic embeddings generated by an FM's text encoder for zero-shot IoT sensing. To utilize the physics principles governing the generation of IoT sensor signals to derive more effective prompts for semantic embedding extraction, we propose to use cross-attention to combine a learnable soft prompt that is optimized automatically on training data and an auxiliary hard prompt that encodes domain knowledge of the IoT sensing task. To address the problem of IoT embeddings biasing to seen classes due to the lack of unseen class data during training, we propose a data augmentation method to synthesize unseen class IoT data for fine-tuning IoT feature extractor and embedding projector. We evaluate our approach on multiple IoT sensing tasks. Experiment results show that our approach achieves superior open-set detection and generalized zero-shot learning performance compared with various baselines.
View details
Exploring the Feasibility of Remote Cardiac Auscultation Using Earphones
Jie Xiong
Tao Chen
Yongjie Yang
Xiuzhen Guo
Shangguan Longfei
MobiCom 2024: The 30th Annual International Conference On Mobile Computing And Networking
Preview abstract
The elderly over 65 accounts for 80% of COVID deaths in the United States. In response to the pandemic, the federal, state governments, and commercial insurers are promoting video visits, through which the elderly can access specialists at home over the Internet, without the risk of COVID exposure. However, the current video visit practice barely relies on video observation and talking. The specialist could not assess the patient's health conditions by performing auscultations.
This paper tries to address this key missing component in video visits by proposing Asclepius, a hardware-software solution that turns the patient's earphones into a stethoscope, allowing the specialist to hear the patient's fine-grained heart sound (i.e., PCG signals) in video visits. To achieve this goal, we contribute a low-cost plug-in peripheral that repurposes the earphone's speaker into a microphone and uses it to capture the patient's minute PCG signals from her ear canal. As the PCG signals suffer from strong attenuation and multi-path effects when propagating from the heart to ear canals, we then propose efficient signal processing algorithms coupled with a data-driven approach to de-reverberate and further correct the amplitude and frequency distortion in raw PCG receptions. We implement Asclepius on a 2-layer PCB board and follow the IRB protocol to evaluate its performance with 30 volunteers. Our extensive experiments show that Asclepius can effectively recover Phonocardiogram (PCG) signals with different types of earphones. The feedback from cardiologists also confirms the efficacy and efficiency of our system. PCG signal samples and benchmark results can be found at an anonymous link https://asclepius-system.github.io/
View details
APG: Audioplethysmography for Cardiac Monitoring in Hearables
Longfei Shangguan
Rich Howard
David Pearl
Trausti Thormundsson
MobiCom 2023: The 29th Annual International Conference On Mobile Computing And Networking (MobiCom), Association for Computing Machinery (ACM) (to appear)
Preview abstract
This paper presents Audioplethysmography (APG), a novel cardiac monitoring modality for active noise cancellation (ANC) headphones. APG sends a low intensity ultrasound probing signal using an ANC headphone's speakers and receives the echoes via the on-board feedback microphones. We observed that, as the volume of ear canals slightly changes with blood vessel deformations, the heartbeats will modulate these ultrasound echoes. We built mathematical models to analyze the underlying physics and propose a multi-tone APG signal processing pipeline to derive the heart rate and heart rate variability in both constrained and unconstrained settings. APG enables robust monitoring of cardiac activities using mass-market ANC headphones in the presence of music playback and body motion such as running.
We conducted an eight-month field study with 153 participants to evaluate APG in various conditions. Our studies conform to the (Institutional Review Board) IRB policies from our company. The presented technology, experimental design, and results have been reviewed and further improved by feedback garnered from our internal Health Team, Product Team, User Experience (UX) Team and Legal team. Our results demonstrate that APG achieves consistently high HR (3.21% median error across 153 participants in all scenarios) and HRV (2.70% median error in interbeat interval, IBI) measurement accuracy. Our UX study further shows that APG is resilient to variation in: skin tone, sub-optimal seal conditions, and ear canal size.
View details
Design Earable Sensing Systems: Perspectives and Lessons Learned from Industry
Trausti Thormundsson
Adjunct Proceedings of the 2023 ACM International Joint Conference on Pervasive and Ubiquitous Computing and the 2023 ACM International Symposium on Wearable Computers, ACM (to appear)
Preview abstract
Earables computing is an emerging research community as the industry witnesses the soaring of True Wireless Stereo (TWS) Active Noise Canceling (ANC) earbuds in the past ten years. There is an increasing trend of newly initiated earable research spanning across mobile health, user-interfaces, speech processing, and context-awareness. Head-worn devices are anticipated to be the next generation Mobile Computing and Human-Computer Interaction (HCI) platform. In this paper, we share our design experiences and lessons learned in building hearable sensing systems from the industry perspective. We also give our takes on future directions of the earable research.
View details