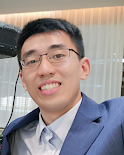
Luowei Zhou
Luowei Zhou is a research scientist at Google Deepmind. He received his Ph.D. degree from the University of Michigan and Bachelor’s degree from Nanjing University. His research lies at the intersection of computer vision and natural language processing, in particular, multimodal foundation models. He organizes the CVPR 2020 & 2021 ActivityNet-Entities Object Localization challenge, co-organizes the CVPR 2020 & 2021 Tutorial on Recent Advances in Vision and Language, and co-organized the CVPR 2018 workshop on Fine-grained Instructional Video Understanding. He has published over 20 papers in top-tier conferences and journals including CVPR, NeurIPS, AAAI, and ECCV and gains media coverages from The Economist, VentureBeat, etc. He won the Best Paper Finalist award and Best Student Paper Honorable Mention award at CVPR 2021.
Research Areas
Authored Publications
Google Publications
Other Publications
Sort By
Preview abstract
To build Video Question Answering (VideoQA) systems capable of assisting humans in daily activities, seeking answers from long-form videos with diverse and complex events is a must. Existing multi-modal VQA models achieve promising performance on images or short video clips, especially with the recent success of large-scale multi-modal pre-training. However, when extending these methods to long-form videos, new challenges arise. On the one hand, using a dense video sampling strategy is computationally prohibitive. On the other hand, methods relying on sparse sampling struggle in scenarios where multi-event and multi-granularity visual reasoning are required. In this work, we introduce a new model named Multi-modal Iterative Spatial-temporal Transformer (MIST) to better adapt pre-trained models for long-form VideoQA. Specifically, MIST decomposes traditional dense spatial-temporal self-attention into cascaded segment and region selection modules that adaptively select frames and image regions that are closely relevant to the question itself. Visual concepts at different granularities are then processed efficiently through an attention module. In addition, MIST iteratively conducts selection and attention over multiple layers to support reasoning over multiple events. The experimental results on four VideoQA datasets, including AGQA, NExT-QA, STAR, and Env-QA, show that MIST achieves state-of-the-art performance and is superior at computation efficiency and interpretability.
View details
MaMMUT: A Simple Vision-Encoder Text-Decoder Architecture for MultiModal Tasks
Wei Li
Abhijit Ogale
Transactions on Machine Learning Research (2023)
Preview abstract
The development of language models have moved from encoder-decoder to decoder-only designs. In addition, the common knowledge has it that the two most popular multimodal tasks, the generative and contrastive tasks, tend to conflict with one another, are hard to accommodate in one architecture, and further need complex adaptations for downstream tasks. We propose a novel paradigm of training with a decoder-only model for multimodal tasks, which is surprisingly effective in jointly learning of these disparate vision-language tasks. This is done with a simple model, called MaMMUT. It consists of a single vision encoder and a text decoder, and is able to accommodate contrastive and generative learning by a novel two-pass approach on the text decoder. We demonstrate that joint learning of these diverse objectives is simple, effective, and maximizes the weight-sharing of the model across these tasks. Furthermore, the same architecture enables straightforward extensions to open-vocabulary object detection and video-language tasks. The model tackles a diverse range of tasks, while being modest in capacity. Our model achieves the state of the art on image-text and text-image retrieval, video question answering and open-vocabulary detection tasks, outperforming much larger and more extensively trained foundational models. It shows very competitive results on VQA and Video Captioning, especially considering its capacity. Ablations confirm the flexibility and advantages of our approach.
View details
No Results Found