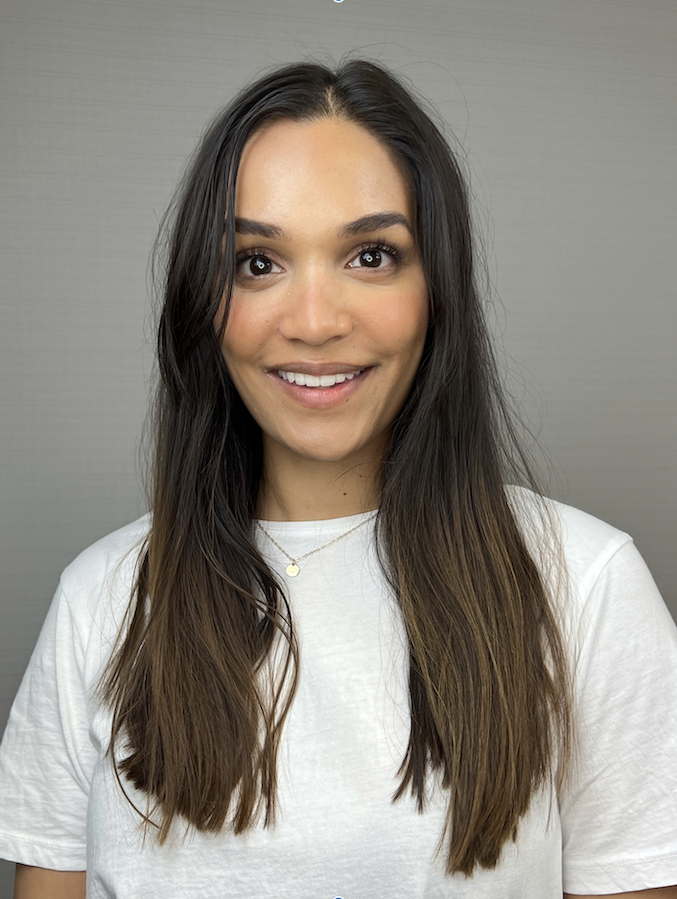
Meera Hahn
I am a research scientist in Google Research, working predominantly at the intersection of computer vision and natural language processing. I joined Google in 2022 after completing my PhD at Georgia Tech. My research interests include embodied AI, text based navigation and localization, text to image and video generation, and general multimodal AI tasks. Check out my homepage for more about me and my research.
Research Areas
Authored Publications
Sort By
Google
Photorealistic Video Generation with Diffusion Models
Agrim Gupta
Kihyuk Sohn
Xiuye Gu
Fei-Fei Li
Lu Jiang
ECCV (2024)
Transformer-based Localization from Embodied Dialog with Large-scale Pre-training
James M. Rehg
The Conference of the Asia-Pacific Chapter of the Association for Computational Linguistics, Association for Computational Linguistics (2022)