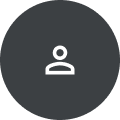
Richard Nock
Research Areas
Authored Publications
Sort By
Google
Fair Wrapping for Black-box Predictions
Alexander Soen
Sanmi Koyejo
Nyalleng Moorosi
Ke Sun
Lexing Xie
NeurIPS (2022)