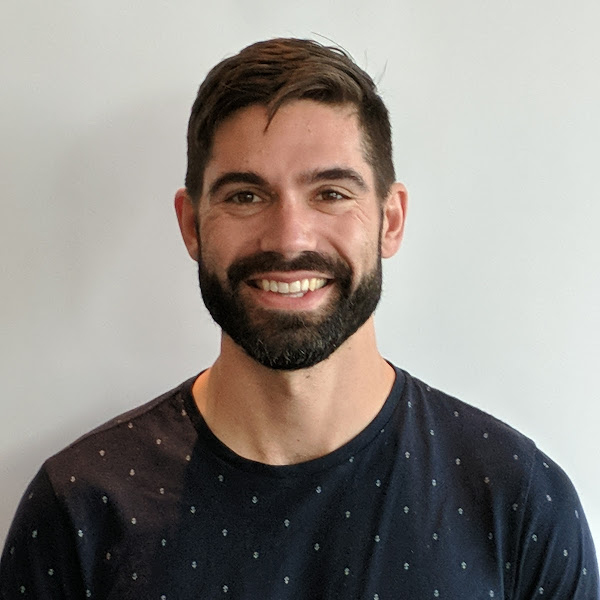
Ben Mathes
Product Manager in Google AI Research. I design and build new products in messy environments. Healthcare, ML/Analytics/Data, macro finance, startups/VC.
Authored Publications
Sort By
Towards ML Engineering: A Brief History Of TensorFlow Extended (TFX)
Abhijit Karmarkar
Ahmet Altay
Aleksandr Zaks
Anusha Ramesh
Jarek Wilkiewicz
Jiri Simsa
Justin Hong
Mitch Trott
Neoklis Polyzotis
Noé Lutz
Robert Crowe
Sarah Sirajuddin
Zhitao Li
(2020)
Preview abstract
Software Engineering, as a discipline, has matured over the past 5+ decades. The modern world heavily depends on it, so the increased maturity of Software Engineering is a necessary blessing. Practices like testing and reliable technologies help make Software Engineering reliable enough to build industries upon. Meanwhile, Machine Learning (ML) has also grown over the past 2+ decades. ML is used more and more for research, experimentation and production workloads. ML now commonly powers widely-used products integral to our lives.
But ML Engineering, as a discipline, has not widely matured as much as its Software Engineering ancestor. Can we take what we have learned and help the nascent field of applied ML evolve into ML Engineering the way Programming evolved into Software Engineering [book]?
In this article we will give a whirlwind tour of Sibyl [article] and TensorFlow Extended (TFX) [website], two successive end-to-end (E2E) ML platforms at Alphabet. We will share the lessons learned from over a decade of applied ML built on these platforms, explain both their similarities and their differences, and expand on the shifts (both mental and technical) that helped us on our journey. In addition, we will highlight some of the capabilities of TFX that help realize several aspects of ML Engineering. We argue that in order to unlock the gains ML can bring, organizations should advance the maturity of their ML teams by investing in robust ML infrastructure and promoting ML Engineering education. We also recommend that before focusing on cutting-edge ML modeling techniques, product leaders should invest more time in adopting interoperable ML platforms for their organizations. In closing, we will also share a glimpse into the future of TFX.
View details