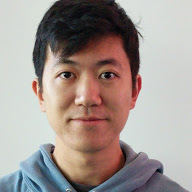
Bo Chang
Research Areas
Authored Publications
Sort By
Preview abstract
Users who come to recommendation platforms are heterogeneous in activity levels. There usually exists a group of core users who visit the platform regularly and consume a large body of contents upon each visit, while others are casual users who tend to visit the platform occasionally and consume less each time.
As a result, consumption activities from core users often dominate the training data used for learning. As core users can exhibit different activity patterns from casual users, recommender systems trained on historical user activity data usually achieve much worse performance on casual users than core users.
To bridge the gap, we propose a model-agnostic framework \textit{L2Aug} to improve recommendations for casual users through data augmentation, without sacrificing core user experience. \textit{L2Aug} is powered by a data augmentor that learns to generate augmented interaction sequences, in order to fine-tune and optimize the performance of the recommendation system for casual users. On four real-world public datasets, the proposed \textit{L2Aug} outperforms other treatment methods and achieves the best sequential recommendation performance for both casual and core users. We also test \textit{L2Aug} in an online simulation environment with real-time feedback to further validate its efficacy, and showcase its flexibility in supporting different augmentation actions.
View details
Preview abstract
Recurrent neural networks have gained widespread use in modeling sequential
data. Learning long-term dependencies using these models remains difficult
though, due to exploding or vanishing gradients. In this paper, we draw connections between recurrent networks and ordinary differential equations. A special
form of recurrent networks called the AntisymmetricRNN is proposed under this
theoretical framework, which is able to capture long-term dependencies thanks to
the stability property of its underlying differential equation. Existing approaches
to improving RNN trainability often incur significant computation overhead. In
comparison, AntisymmetricRNN achieves the same goal by design. We showcase
the advantage of this new architecture through extensive simulations and experiments. AntisymmetricRNN exhibits much more predictable dynamics. It outperforms regular LSTM models on tasks requiring long-term memory and matches
the performance on tasks where short-term dependencies dominate despite being
much simpler.
View details