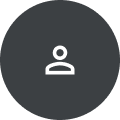
Dustin Zelle
Research Areas
Authored Publications
Sort By
Learning Large Graph Property Prediction via Graph Segment Training
Kaidi Cao
Mangpo Phothilimthana
Charith Mendis
Jure Leskovec
Advances in Neural Information Processing Systems (2023)
Preview abstract
Learning to predict properties of large graphs is challenging because each prediction requires the knowledge of an entire graph, while the amount of memory available during training is bounded. Here we propose Graph Segment Training (GST), a general framework that utilizes a divide-and-conquer approach to allow learning large graph property prediction with a constant memory footprint. GST first divides a large graph into segments and then backpropagates through only a few segments sampled per training iteration. We refine the GST paradigm by introducing a historical embedding table to efficiently obtain embeddings for segments not sampled for backpropagation. To mitigate the staleness of historical embeddings, we design two novel techniques. First, we finetune the prediction head to fix the input distribution shift. Second, we introduce Stale Embedding Dropout to drop some stale embeddings during training to reduce bias. We evaluate our complete method GST-EFD (with all the techniques together) on two large graph property prediction benchmarks: MalNet and TpuGraphs. Our experiments show that GST-EFD is both memory-efficient and fast, while offering a slight boost on test accuracy over a typical full graph training regime.
View details
Zero-shot Transfer Learning within a Heterogeneous Graph via Knowledge Transfer Networks
Minji Yoon
Ziniu Hu
Ruslan Salakhutdinov
Advances in Neural Information Processing Systems (2022)
Preview abstract
Data continuously emitted from industrial ecosystems such as social or e-commerce platforms are commonly represented as heterogeneous graphs (HG) composed of multiple node/edge types. State-of-the-art graph learning methods for HGs known as heterogeneous graph neural networks (HGNNs) are applied to learn deep context-informed node representations. However, many HG datasets from industrial applications suffer from label imbalance between node types. As there is no direct way to learn using labels rooted at different node types, HGNNs have been applied to only a few node types with abundant labels. We propose a zero-shot transfer learning module for HGNNs called a Knowledge Transfer Network (KTN) that transfers knowledge from label-abundant node types to zero-labeled node types through rich relational information given in the HG. KTN is derived from the theoretical relationship, which we introduce in this work, between distinct feature extractors for each node type given in an HGNN model. KTN improves the performance of 6 different types of HGNN models by up to 960% for inference on zero-labeled node types and outperforms state-of-the-art transfer learning baselines by up to 73% across 18 different transfer learning tasks on HGs.
View details
DDGK: Learning Graph Representations for Deep Divergence Graph Kernels
Rami Al-Rfou
Proceedings of the 2019 World Wide Web Conference on World Wide Web
Preview abstract
Can neural networks learn to compare graphs without feature engineering?
In this paper, we show that it is possible to learn representations for graph similarity with neither domain knowledge nor supervision (i.e. feature engineering or labeled graphs).
We propose Deep Divergence Graph Kernels, an unsupervised method for learning representations over graphs that encodes a relaxed notion of graph isomorphism.
Our method consists of three parts.
First, we learn an encoder for each anchor graph to capture its structure.
Second, for each pair of graphs, we train a cross-graph attention network which uses the node representations of an anchor graph to reconstruct another graph.
This approach, which we call isomorphism attention, captures how well the representations of one graph can encode another.
We use the attention-augmented encoder's predictions to define a divergence score for each pair of graphs.
Finally, we construct an embedding space for all graphs using these pair-wise divergence scores.
Unlike previous work, much of which relies on 1) supervision, 2) domain specific knowledge (e.g. a reliance on Weisfeiler-Lehman kernels), and 3) known node alignment, our unsupervised method jointly learns node representations, graph representations, and an attention-based alignment between graphs.
Our experimental results show that Deep Divergence Graph Kernels can learn an unsupervised alignment between graphs, and that the learned representations achieve competitive results when used as features on a number of challenging graph classification tasks.
Furthermore, we illustrate how the learned attention allows insight into the the alignment of sub-structures across graphs.
View details