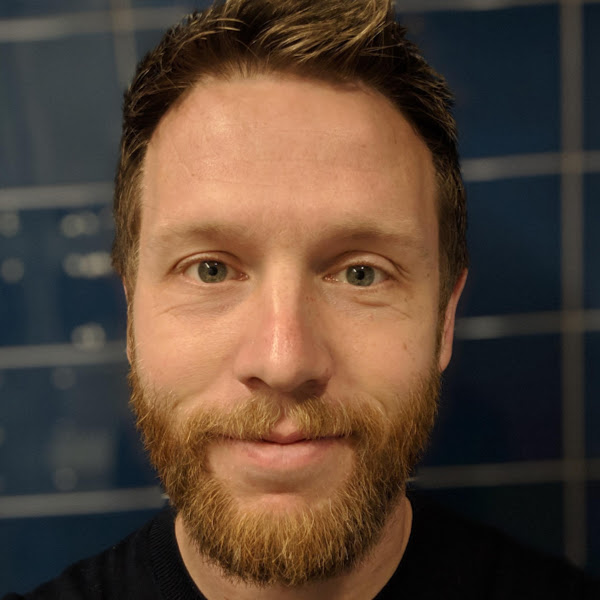
Kevin Regan
Kevin received his PhD from the University of Toronto where he focused on models of decision making and preference elicitation (homepage). Recently he has been working on large-scale machine learning and ad click prediction at Google Pittsburgh.
Research Areas
Authored Publications
Sort By
Preview abstract
We present a formulation of deep learning that aims at producing a large margin classifier. The notion of margin has served as the foundation of several theoretically profound and empirically successful results for both classification and regression tasks. However, most large margin algorithms are applicable only to shallow models with preset feature representation; and existing margin methods for neural networks only enforce margin at the output layer, or are formulated with weak approximations to the true margin. This keeps margin methods inaccessible to models like deep networks. In this paper, we propose a novel loss function to impose a margin on any set of layers of deep network and show promising empirical results that consistently outperform cross-entropy based models across different application scenarios such as adversarial examples and generalization from small training sets. Our formulation allows choosing any norm for the margin. The resulting loss is general and complementary to existing regularization techniques such as weight decay, dropout and batch norm. It is applicable to any classification task where cross-entropy is used.
View details