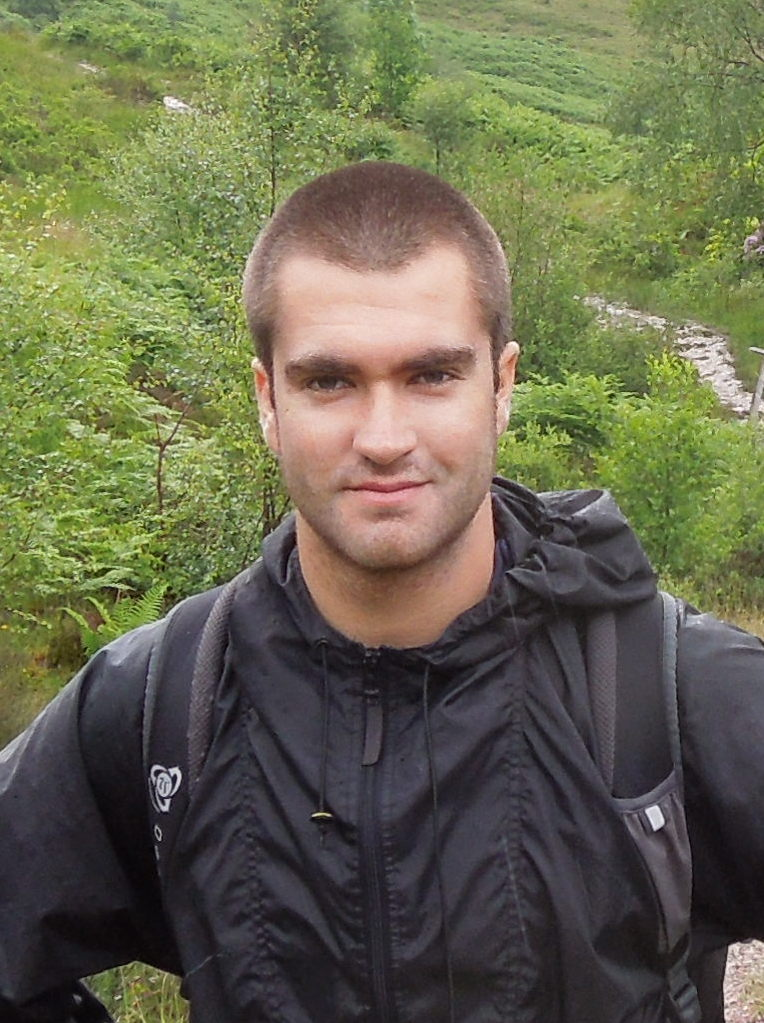
David Belanger
I am a research scientist in the Cambridge, MA branch of the Google Brain Team. I recently received a PhD from UMass Amherst, where I was advised by Andrew McCallum. Before grad school, I worked on optical character recognition at BBN Technologies and before that I attended Harvard, where I researched numerical methods for simulating earthquake ruptures on rough faults. During grad school, I also interned with Sham Kakade and Dilip Krishnan. You can find links to all of my pre-Google papers david-belanger.net.
My grad school research spanned graphical models, structured prediction, and deep learning. I have applied these methods to both natural language processing and computer vision tasks. Broadly speaking, I’m interested in developing accurate machine learning methods that leverage practitioners’ expertise about the problem domain, can be fit reliably using limited data, provide fair and un-biased behavior, appropriately quantify their uncertainty, offer interpretable predictions, and can be run using limited power on widely-accessible hardware. This requires both fundamental progress in machine learning methods and also close collaboration with a variety of domain experts. Fortunately, Google provides great opportunities for both.
In my free time, I enjoy running, rock climbing, cycling, grilling, traveling, and spending time with my family.
Research Areas
Authored Publications
Sort By
Rethinking Attention with Performers
Valerii Likhosherstov
David Martin Dohan
Peter Hawkins
Jared Quincy Davis
Afroz Mohiuddin
Lukasz Kaiser
Adrian Weller
accepted to ICLR 2021 (oral presentation) (to appear)
Preview abstract
We introduce Performers, Transformer architectures which can estimate regular (softmax) full-rank-attention Transformers with provable accuracy, but using only linear (as opposed to quadratic) space and time complexity, without relying on any priors such as sparsity or low-rankness. To approximate softmax attention-kernels, Performers use a novel Fast Attention Via positive Orthogonal Random features approach (FAVOR+), which may be of independent interest for scalable kernel methods. FAVOR+ can be also used to efficiently model kernelizable attention mechanisms beyond softmax. This representational power is crucial to accurately compare softmax with other kernels for the first time on large-scale tasks, beyond the reach of regular Transformers, and investigate optimal attention-kernels. Performers are linear architectures fully compatible with regular Transformers and with strong theoretical guarantees: unbiased or nearly-unbiased estimation of the attention matrix, uniform convergence and low estimation variance. We tested Performers on a rich set of tasks stretching from pixel-prediction through text models to protein sequence modeling. We demonstrate competitive results with other examined efficient sparse and dense attention methods, showcasing effectiveness of the novel attention-learning paradigm leveraged by Performers.
View details
Population Based Optimization for Biological Sequence Design
Zelda Mariet
David Martin Dohan
D. Sculley
ICML 2020 (2020)
Preview abstract
The use of black-box optimization for the design of new biological sequences is an emerging research area with potentially revolutionary impact. The cost and latency of wet-lab experiments requires methods that find good sequences in few experimental rounds of large batches of sequences --- a setting that off-the-shelf black-box optimization methods are ill-equipped to handle. We find that the performance of existing methods varies drastically across optimization tasks, posing a significant obstacle to real-world applications. To improve robustness, we propose population-based optimization (PBO), which generates batches of sequences by sampling from an ensemble of methods. The number of sequences sampled from any method is proportional to the quality of sequences it previously proposed, allowing PBO to combine the strengths of individual methods while hedging against their innate brittleness. Adapting the population of methods online using evolutionary optimization further improves performance. Through extensive experiments on in-silico optimization tasks, we show that PBO outperforms any single method in its population, proposing both higher quality single sequences as well as more diverse batches. By its robustness and ability to design diverse, high-quality sequences, PBO is shown to be a new state-of-the art approach to the batched black-box optimization of biological sequences.
View details
Model-Based Reinforcement Learning for Biological Sequence Design
David Dohan
Ramya Deshpande
ICLR 2020 (2020)
Preview abstract
Being able to design biological sequences like DNA or proteins to have desired properties would have considerable impact in medical and industrial applications. However, doing so presents a challenging black-box optimization problem that requires multiple rounds of expensive, time-consuming experiments. In response, we propose using reinforcement learning (RL) for biological sequence design. RL is a flexible framework that allows us to optimize generative sequence policies to achieve a variety of criteria, including diversity among high-quality sequences discovered. We use model-based RL to improve sample efficiency, where at each round the policy is trained offline using a simulator fit on functional measurements from prior rounds. To accommodate the growing number of observations across rounds, the simulator model is automatically selected at each round from a pool of diverse models of varying capacity. On the tasks of designing DNA transcription factor binding sites, designing antimicrobial proteins, and optimizing the energy of Ising models based on protein structures, we find that model-based RL is an attractive alternative to existing methods.
View details
Biological Sequences Design using Batched Bayesian Optimization
Zelda Mariet
Ramya Deshpande
David Dohan
Olivier Chapelle
NeurIPS workshop on Bayesian Deep Learning (2019)
Preview abstract
Being able to effectively design biological sequences like DNA and proteins would have transformative impact on medicine. Currently, the most popular method in the life sciences for performing design is directed evolution,which explores sequence space by making small mutations to existing sequences.Alternatively, Bayesian optimization (BO) provides an attractive framework for model-based black-box optimization, and has achieved many recent successes in life sciences applications. However, within the ML community, most large-scale BO efforts have focused on hyper-parameter tuning. These methods often do not translate to biological sequence design, where the search space is over a discrete alphabet, wet-lab experiments are run with considerable parallelism (1K-100K sequences per batch), and experiments are sufficiently slow and expensive that only few rounds of experiments are feasible. This paper discusses the particularities of batched BO on a large discrete space, and investigates the design choices that must be made in order to obtain robust, scalable, and experimentally successful models within this unique context.
View details
Preview abstract
When confronted with a substance of unknown identity, researchers often perform mass spectrometry on the sample and compare the observed spectrum to a library of previously collected spectra to identify the molecule. While popular, this approach will fail to identify molecules that are not in the existing library. In response, we propose to improve the library’s coverage by augmenting it with synthetic spectra that are predicted from candidate molecules using machine learning. We contribute a lightweight neural network model that quickly predicts mass spectra for small molecules, averaging 5 ms per molecule with a recall-at-10 accuracy of 91.8%. Achieving high-accuracy predictions requires a novel neural network architecture that is designed to capture typical fragmentation patterns from electron ionization. We analyze the effects of our modeling innovations on library matching performance and compare our models to prior machine-learning-based work on spectrum prediction.
View details
A Comparison of Generative Models for Sequence Design
David Dohan
Ramya Deshpande
Olivier Chapelle
Babak Alipanahi
Machine Learning in Computational Biology Workshop (2019)
Preview abstract
In this paper, we compare generative models of different complexity for designing DNA and protein sequences using the Cross Entropy Method.
View details
Critiquing Protein Family Classification Models Using Sufficient Input Subsets
Brandon Michael Carter
Jamie Alexander Smith
Theo Sanderson
ACM SIGKDD Conference on Knowledge Discovery and Data Mining (2019) (to appear)
Preview abstract
In many application domains, neural networks are highly accurate and have been deployed at large scale. However, users often do not have good tools for understanding how these models arrive at their predictions. This has hindered adoption in fields such as the life and medical sciences, where researchers require that models base their decisions on underlying biological phenomena rather than peculiarities of the dataset introduced, e.g., as a function of when and how the data were collected. In response, we propose a set of methods for critiquing deep learning models, and demonstrate their application for protein family classification, a task for which high- accuracy models have considerable potential impact. Our methods extend the recently-introduced sufficient input subsets technique (SIS), which we use to identify the subset of locations (SIS) in each protein sequence that is sufficient for classification. Our suite of tools analyzes these SIS to shed light on the decision making criteria employed by models trained on this task. These tools expose that while these deep models may perform classification for biologically-relevant reasons, their behavior varies considerably across choice of network architecture and parameter initialization. While the techniques that we develop are specific to the protein sequence classification task, the approach taken generalizes to a broad set of scientific contexts in which model interpretability is essential. We encourage further application of our techniques for interrogating machine learning models trained on other scientifically relevant tasks.
View details
Deep Learning Classifies the Protein Universe
Theo Sanderson
Brandon Carter
D. Sculley
Mark DePristo
Nature Biotechnology (2019)
Preview abstract
Understanding the relationship between amino acid sequence and protein function is a long-standing problem in molecular biology with far-reaching scientific implications. Despite six decades of progress, state-of-the-art techniques cannot annotate $\sim1/3$ of microbial protein sequences, hampering our ability to exploit sequences collected from diverse organisms. To address this, we report a deep learning model that learns the relationship between unaligned amino acid sequences and their functional classification across all 17929 families of the PFam database. Using the Pfam seed sequences we establish a rigorous benchmark assessment and find that a dilated convolutional model reduces the error of state of the art BLASTp and pHMM models by a factor of nine. With 80\% of the full Pfam database we train a protein family predictor that is more accurate and over 200 times faster than BLASTp, while learning sequence features such as structural disorder and transmembrane helices. Our model co-locates sequences from unseen families in embedding space far from existing families, allowing sequences from novel families to be classified. We anticipate that deep learning models will be a core component of future general-purpose protein function prediction tools.
View details
Sequential regulatory activity prediction across chromosomes with convolutional neural networks
David Kelley
Yakir Reshef
Genome Research (2018)
Preview abstract
Functional genomics approaches to better model genotype-phenotype relationships have important
applications toward understanding genomic function and improving human health. In particular,
thousands of noncoding loci associated with diseases and physical traits lack mechanistic
explanation. Here, we develop the first machine-learning system to predict cell type-specific
epigenetic and transcriptional profiles in large mammalian genomes from DNA sequence alone.
Using convolutional neural networks, this system identifies promoters and distal regulatory elements
and synthesizes their content to make effective gene expression predictions. We show that model
predictions for the influence of genomic variants on gene expression align well to causal variants
underlying eQTLs in human populations and can be useful for generating mechanistic hypotheses
to enable GWAS loci fine mapping.
View details
Preview abstract
Gradient descent methods have greatly facilitated the practice of machine learning, as the learning problem can be usually represented as the minimization of a differentiable function over some parameters. However, in cases where some dependencies between parameters and variables are discrete, gradient descent cannot be applied, unless those discrete nodes are relaxed to continued values ones, where derivatives can be defined. Nonetheless, no clear solution exists in cases of structured discrete objects defined by a certain combinatorial structure; for example, in permutations, which underlie the notions of ordering, ranking and matching of objects. Here we show how to extend the relaxation method to enable gradient descent in computational graphs containing permutations as deterministic or stochastic nodes. To this end, we first show that permutations can be approximated by the differentiable Sinkhorn operator. With this, we are able to define Sinkhorn networks for the supervised learning of permutations. Finally, for stochastic nodes (corresponding to latent distributions over permutations) we introduce two implicit distributions: Gumbel-Matching and its relaxation, the Gumbel-Sinkhorn, and we prescribe how to perform inferences. We demonstrate the effectiveness of our method by showing we achieve state-of-the-art results on several tasks involving both standard datasets and a scientific application.
View details