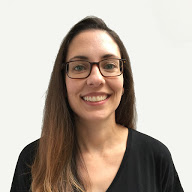
Laura Vardoulakis
Laura Vardoulakis leads User Experience Research for Google Health. Previously, she led Search, Intelligence, and cross-Apps research for G Suite. Prior to Google, her research was at the intersection of HCI, CS, and Medicine: developing conversational, agent-based interactions designed to improve health outcomes. She received her Ph.D. in Computer Science from Northeastern University and her research has been published in CHI, Intelligent Virtual Agents, AAAI, and featured in The Wall Street Journal and MIT Technology Review.
Authored Publications
Sort By
User-centred design for machine learning in health care: a case study from care management
Birju Patel
Daniel Lopez-martinez
Doris Wong
Eric Loreaux
Janjri Desai
Jonathan Chen
Lance Downing
Lutz Thomas Finger
Martin Gamunu Seneviratne
Ming-Jun Chen
Nigam Shah
Ron Li
BMJ Health & Care Informatics (2022)
Preview abstract
Objectives: Few machine learning (ML) models are successfully deployed in clinical practice. One of the common pitfalls across the field is inappropriate problem formulation: designing ML to fit the data rather than to address a real-world clinical pain point.
Methods: We introduce a practical toolkit for user-centred design consisting of four questions covering: (1) solvable pain points, (2) the unique value of ML (eg, automation and augmentation), (3) the actionability pathway and (4) the model’s reward function. This toolkit was implemented in a series of six participatory design workshops with care managers in an academic medical centre.
Results: Pain points amenable to ML solutions included outpatient risk stratification and risk factor identification. The endpoint definitions, triggering frequency and evaluation metrics of the proposed risk scoring model were directly influenced by care manager workflows and real-world constraints.
Conclusions: Integrating user-centred design early in the ML life cycle is key for configuring models in a clinically actionable way. This toolkit can guide problem selection and influence choices about the technical setup of the ML problem.
View details
Isolation in Coordination: Challenges of Caregivers in the USA
Mark Schlager
Laura Pina
Lauren Wilcox
Proceedings of the ACM CHI 2021 Conference on Human Factors in Computing Systems, ACM (2021)
Preview abstract
As the global population ages and the prevalence of chronic conditions and acute infections rise, it is becoming imperative to understand the many forms of caregiving labor and create sociotechnical systems that support them. In this paper, we report results of a large survey study with 2000 informal caregivers in the USA, highlighting the fundamental challenges that different types of caregivers face when coordinating care with others. Our findings support previous findings on caregivers' coordination challenges, while also offering insights into the situational, mediating factors that influence the extent to which care coordination challenges are felt. These mediating factors include caregivers' relationships, access to a variety of resources including physical, social, and financial support, and physical and mental barriers. We discuss these challenges and mediating factors, and conclude with a discussion on how they can be considered in the design of future sociotechnical systems.
View details
A Human-Centered Evaluation of a Deep Learning System Deployed in Clinics for the Detection of Diabetic Retinopathy
Emma Beede
Elizabeth Baylor
Fred Hersch
Lauren Wilcox
Dr. Paisan Raumviboonsuk
Proceedings of the 2020 CHI Conference on Human Factors in Computing Systems (2020)
Preview abstract
Deep learning algorithms promise to improve clinician workflows and patient outcomes. However, these gains have yet to be fully demonstrated in real world clinical settings. In this paper, we describe a human-centered study of a deep learning system used in clinics for the detection of diabetic eye disease. Through observation and interviews with nurses across eleven clinics across Thailand, we characterize several socio-environmental factors that impact model performance, nursing workflows, and patient experience. We find tensions between the model’s thresholds for data quality, and the quality of data that arise from an imperfect, resource-constrained environment. We discuss several advantages to conducting human-centered evaluative research alongside prospective evaluations of model accuracy, including: understanding contextual practices of clinicians and patients in order to inform system design, being able to utilize authentic clinical data in system evaluations, and understanding how the system operates within the context of clinical care prior to widespread deployment.
View details
Predicting inpatient medication orders from electronic health record data
Kathryn Rough
Kun Zhang
Atul J. Butte
Alvin Rajkomar
Clinical Pharmacology and Therapeutics (2020)
Preview abstract
In a general inpatient population, we predicted patient‐specific medication orders based on structured information in the electronic health record (EHR). Data on over three million medication orders from an academic medical center were used to train two machine‐learning models: A deep learning sequence model and a logistic regression model. Both were compared with a baseline that ranked the most frequently ordered medications based on a patient’s discharge hospital service and amount of time since admission. Models were trained to predict from 990 possible medications at the time of order entry. Fifty‐five percent of medications ordered by physicians were ranked in the sequence model’s top‐10 predictions (logistic model: 49%) and 75% ranked in the top‐25 (logistic model: 69%). Ninety‐three percent of the sequence model’s top‐10 prediction sets contained at least one medication that physicians ordered within the next day. These findings demonstrate that medication orders can be predicted from information present in the EHR.
View details
Automatically Charting Symptoms From Patient-Physician Conversations Using Machine Learning
Alvin Rishi Rajkomar
Kat Chou
Journal of the American Medical Association (2019)
Preview abstract
Introduction: Auto-charting -- creation structured sections of clinical notes generated directly from a patient-doctor encounter -- holds promise to lift documentation burden from physicians. However, clinicians exercise professional judgement in what and how to document, and it is unknown if a machine learning (ML) model could assist with these tasks.
Objective: Build a ML model to extract symptoms and status (i.e. experienced, not-experienced, not relevant for note) from transcripts of patient-doctor encounters and assess performance on common symptoms and conversations in which a human interpreterscribe is not used.
Methods: We generated a ML model to auto-generate a review of systems (ROS) from transcripts of 90,000 de-identified medical encounters. 2950 transcripts were labeled by medical scribes to identify 171 common symptoms. Model accuracy was stratified by how clearly a symptom was mentioned in conversation for 800 snippets, which was assessed by a formal rating system termed conversational clarity. The model was also qualitatively assessed in a variety of conversational motifs.
Results: Overall, the model had a sensitivity of 0.71 of matching the exact symptom labeled by a human with a positive predictive value of 0.69. Model sensitivity was associated with the clarity of a conversational (p<0.0001). 39.5% (316/800) snippets of common symptoms contained symptoms mentioned with high clarity, and in this group, the sensitivity of the model was 0.91. The model was robust to a variety of conversational motifs (e.g. detecting symptoms mentioned in colloquial ways).
Conclusions: Auto-generating a review of systems is feasible across a wide-range symptoms that are commonly discussed in doctor-patient encounter
View details
Personal Tasks at Work: An Exploration
Sarah McRoberts
Proceedings of the 20th ACM Conference on Computer Supported Cooperative Work and Social Computing Companion, ACM, New York, NY, USA (2017) (to appear)
Preview abstract
Technology has changed the way that people work, socialize, and generally live their lives. Especially, technology has changed the way that those distinct parts of a person’s life intermingle. Previous work has largely focused on different styles of work-life balance, and most of the industry contributions to this problem focus on preventing work tasks from leaking into one’s personal life. However, little work has been done to investigate the ways that personal tasks impact the work day. We present the results of a survey of 93 employed people, as they relate to personal tasks that people do at work. We consider the context, consequences, and persistency of these tasks, and propose some design implications that could better reinforce the individual boundaries that people have between their work and personal lives.
View details