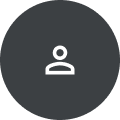
Yuxue Jin
Research Areas
Authored Publications
Sort By
Google
Bias Correction For Paid Search In Media Mix Modeling
Jim Koehler
Mike Perry
research.google.com, Google Inc. (2018)
Bayesian Methods for Media Mix Modeling with Carryover and Shape Effects
Jim Koehler
research.google.com, Google Inc., 76 Ninth Avenue
Google New York
NY 10011 (2017)
How Many People Visit YouTube? Imputing Missing Events in Panels With Excess Zeros
Georg M. Goerg
Nicolas Remy
Jim Koehler
; SAGE Publications - edited by Herwig Friedl and Helga Wagner, Linz, Austria (2015), pp. 1-6
The Optimal Mix of TV and Online Ads to Maximize Reach
Jim Koehler
Georg M. Goerg
Nicolas Remy
research.google.com, 76 Ninth Avenue (2013), pp. 1-16