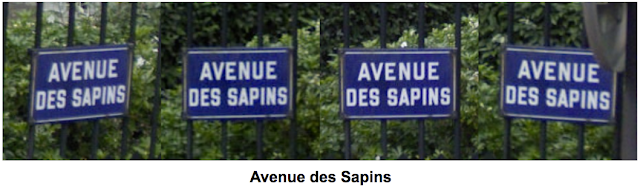
Updating Google Maps with Deep Learning and Street View
May 3, 2017
Posted by Julian Ibarz, Staff Software Engineer, Google Brain Team and Sujoy Banerjee, Product Manager, Ground Truth Team
Quick links
Every day, Google Maps provides useful directions, real-time traffic information and information on businesses to millions of people. In order to provide the best experience for our users, this information has to constantly mirror an ever-changing world. While Street View cars collect millions of images daily, it is impossible to manually analyze more than 80 billion high resolution images collected to date in order to find new, or updated, information for Google Maps. One of the goals of the Google’s Ground Truth team is to enable the automatic extraction of information from our geo-located imagery to improve Google Maps.
In “Attention-based Extraction of Structured Information from Street View Imagery”, we describe our approach to accurately read street names out of very challenging Street View images in many countries, automatically, using a deep neural network. Our algorithm achieves 84.2% accuracy on the challenging French Street Name Signs (FSNS) dataset, significantly outperforming the previous state-of-the-art systems. Importantly, our system is easily extensible to extract other types of information out of Street View images as well, and now helps us automatically extract business names from store fronts. We are excited to announce that this model is now publicly available!
![]() |
Example of street name from the FSNS dataset correctly transcribed by our system. Up to four views of the same sign are provided. |
In 2014, Google’s Ground Truth team published a state-of-the-art method for reading street numbers on the Street View House Numbers (SVHN) dataset, implemented by then summer intern (now Googler) Ian Goodfellow. This work was not only of academic interest but was critical in making Google Maps more accurate. Today, over one-third of addresses globally have had their location improved thanks to this system. In some countries, such as Brazil, this algorithm has improved more than 90% of the addresses in Google Maps today, greatly improving the usability of our maps.
The next logical step was to extend these techniques to street names. To solve this problem, we created and released French Street Name Signs (FSNS), a large training dataset of more than 1 million street names. The FSNS dataset was a multi-year effort designed to allow anyone to improve their OCR models on a challenging and real use case. FSNS dataset is much larger and more challenging than SVHN in that accurate recognition of street signs may require combining information from many different images.
![]() |
Example of text normalization learned from data in Brazil. Here it changes “AV.” into “Avenida” and “Pres.” into “Presidente” which is what we desire. |
![]() |
In this example, the model is not confused from the fact that there is two street names, properly normalizes “Av” into “Avenue” as well as correctly ignores the number “1600”. |
This new system, combined with the one extracting street numbers, allows us to create new addresses directly from imagery, where we previously didn’t know the name of the street, or the location of the addresses. Now, whenever a Street View car drives on a newly built road, our system can analyze the tens of thousands of images that would be captured, extract the street names and numbers, and properly create and locate the new addresses, automatically, on Google Maps.
But automatically creating addresses for Google Maps is not enough -- additionally we want to be able to provide navigation to businesses by name. In 2015, we published “Large Scale Business Discovery from Street View Imagery”, which proposed an approach to accurately detect business store-front signs in Street View images. However, once a store front is detected, one still needs to accurately extract its name for it to be useful -- the model must figure out which text is the business name, and which text is not relevant. We call this extracting “structured text” information out of imagery. It is not just text, it is text with semantic meaning attached to it.
Using different training data, the same model architecture that we used to read street names can also be used to accurately extract business names out of business facades. In this particular case, we are able to only extract the business name which enables us to verify if we already know about this business in Google Maps, allowing us to have more accurate and up-to-date business listings.
publicly announced earlier this year, to drastically reduce the computational cost of the inferences of our pipeline.
People rely on the accuracy of Google Maps in order to assist them. While keeping Google Maps up-to-date with the ever-changing landscape of cities, roads and businesses presents a technical challenge that is far from solved, it is the goal of the Ground Truth team to drive cutting-edge innovation in machine learning to create a better experience for over one billion Google Maps users.