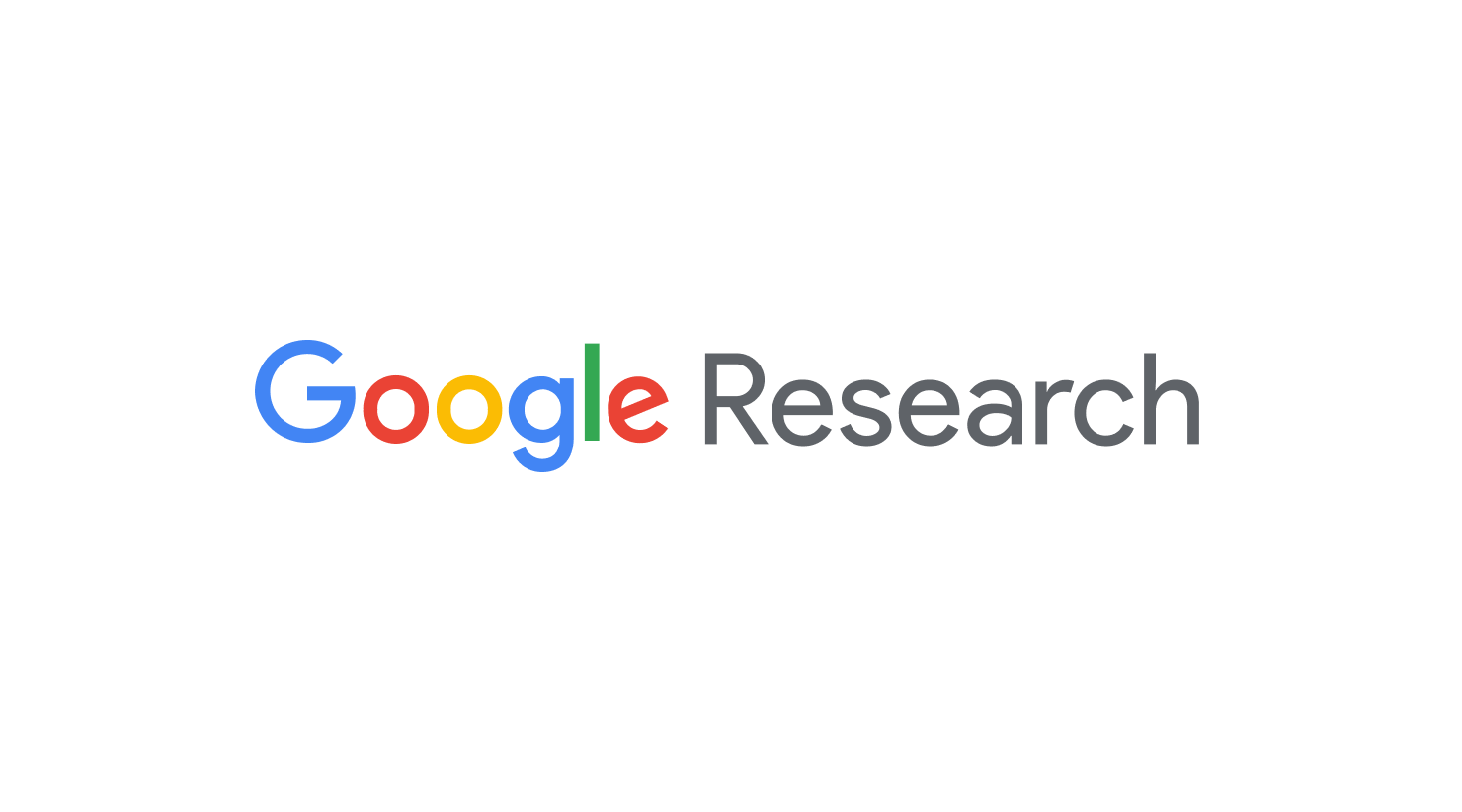
Overlapping Experiment Infrastructure: More, Better, Faster Experimentation
April 5, 2011
Posted by Deirdre O'Brien and Diane Tang, Adwords Team
At Google, experimentation is practically a mantra; we evaluate almost every change that potentially affects what our users experience. Such changes include not only obvious user-visible changes such as modifications to a user interface, but also more subtle changes such as different machine learning algorithms that might affect ranking or content selection. Our insatiable appetite for experimentation has led us to tackle the problems of how to run more experiments, how to run experiments that produce better decisions, and how to run them faster.
Google's infrastructure supports this vast experimentation by using orthogonal diversion criteria for experiments in different "layers" so that each event (e.g. a web search) can be assigned to multiple experiments. The treatment and population sample are easily specified in data files allowing for fast and accurate experiment set up. We have also developed analytical tools to do experiment sizing and a metrics dashboard which provides summarized data within hours of experiment set up. Decision making is improved by the consistency and accuracy in metrics assured by these tools. We believe that Google's experimental system and processes described in this paper can be generalized and applied by any entity interested in using experimentation to improve search engines and other web applications.