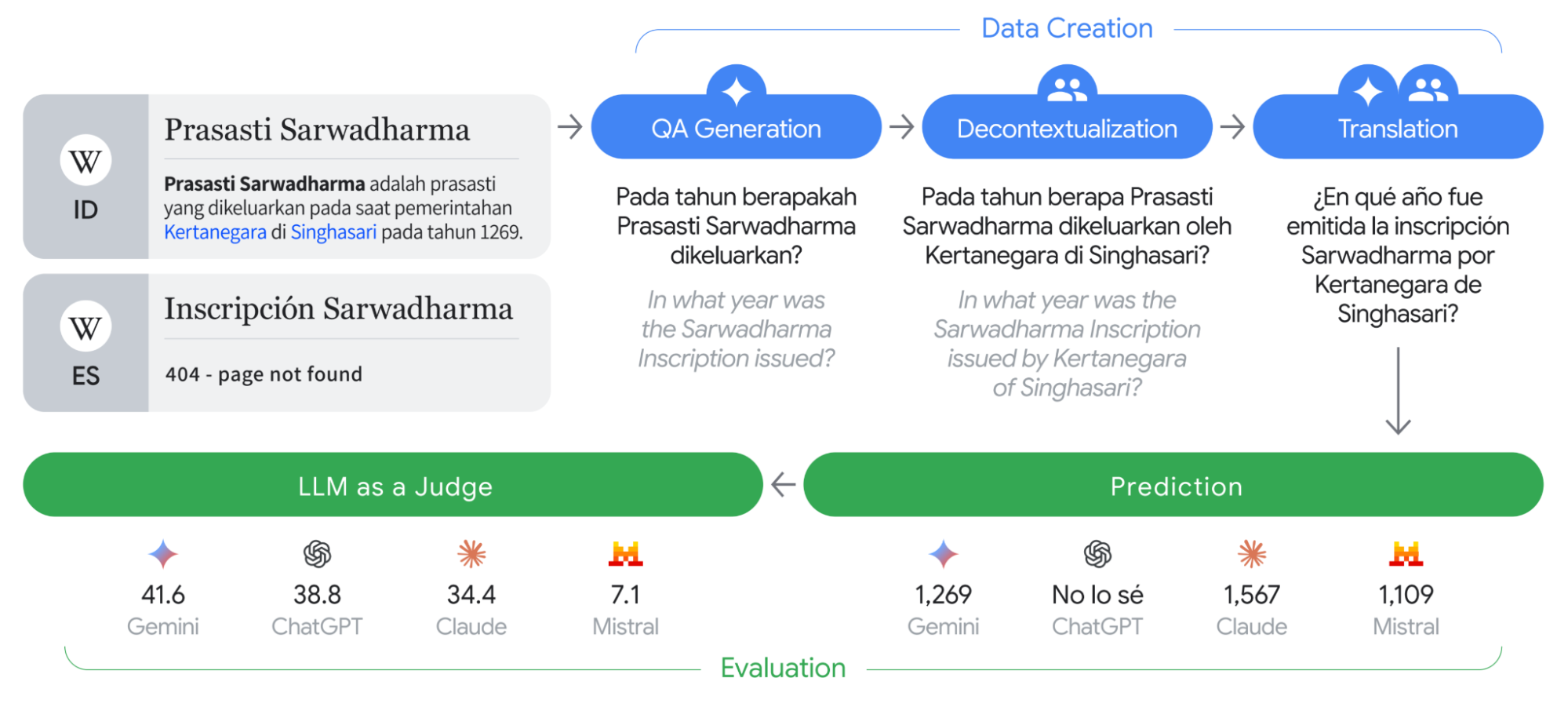
ECLeKTic: A novel benchmark for evaluating cross-lingual knowledge transfer in LLMs
April 2, 2025
Omer Goldman, Student Researcher, and Uri Shaham, Research Scientist, Google Research
ECLeKTic is a new benchmark designed to evaluate the ability of large language models (LLMs) to transfer knowledge across different languages. It uses a closed-book question answering task, where models must rely on their internal knowledge to answer questions based on information relevant to a specific language
Quick links
Imagine you are traveling around eastern Java, Indonesia, and your tour guide mentions the Sarwadharma inscription, an ancient artifact from the time of King Kertanagara that was found nearby. You want to know more, so you go online and ask a large language model (LLM), like Gemini, for some details. You soon find that if you ask the model in Indonesian, the model provides detailed information about the Sarwadharma inscription — for example, it knows that the artifact was written in 1269. But when asked in other languages, for example Chinese or German, the model does not have the same level of knowledge. In other words, the knowledge is inaccessible simply because of the language in which you asked. This is a common limitation for all leading LLMs today [1, 2].
This behavior is more than a quirk, it highlights a difference between human linguistic capabilities and those of LLMs, as humans generally possess the ability to access and express the same knowledge in all languages they speak. A model with such capabilities would enable speakers of languages with smaller resource footprints online to have equal access to the world's knowledge, a disproportionate amount of which is encoded in only a few languages. It would also enable speakers of those higher resource languages to access a wider range of information. In order to improve LLMs’ cross-lingual knowledge transfer capability, we first need to measure it.
In our recent work, we present ECLeKTic, a dataset for Evaluating Cross-Lingual Knowledge Transfer, to help discover the prevalence of knowledge accessibility discrepancies in LLMs. ECLeKTic is a question answering (QA) dataset that evaluates transfer in a simple black-box manner (i.e., using only the model’s input and output). So, unlike previous measures, it is easily applicable to proprietary models. ECLeKTic is based on knowledge that models have likely observed in only a single language during training by targeting Wikipedia articles that exist only in one language, like the article about the Sarwadharma inscription that exists only on the Indonesian Wikipedia. ECLeKTic, which is released as an open source benchmark on Kaggle, includes questions in 12 languages. We used ECLeKTic to benchmark various LLMs. In the paper we evaluated 8 of the leading LLMs, both open and proprietary. Among the models we tested Gemini 2.0 Pro performed the best, achieving 41.6% in terms of overall success. Since the publication of the paper a new version of Gemini was released, Gemini 2.5 Pro, that managed to top that result with 52.6% overall success. All in all, these results indicate that there is still room to improve state-of-the-art LLMs on the task of cross-lingual knowledge transfer.
Data creation and verification
To construct ECLeKTic, we started by selecting articles that only exist in a single language on Wikipedia for 12 languages — English, French, German, Hebrew, Hindi, Indonesian, Italian, Japanese, Korean, Mandarin Chinese, Portuguese, and Spanish. These pages are often based on topics most salient to speakers of that language, but they may very well include information that is of interest to others around the world. Of course, models may learn about these topics from other sources, but since it is not possible to analyze the training data of every LLM, we use presence in Wikipedia as a proxy for whether the model has seen information in a particular language. With this assumption, focusing on this kind of content suggests that models would need to internally transfer the knowledge from the source language to the other 11 target languages in order to solve ECLeKTic’s QA task.
Specifically, we analyzed the July 2023 download of Wikipedia. For each language, we selected 100 random articles that contained at least 200 characters, had at least 100 views during 2023, and most importantly, did not have equivalent articles in any of the other 11 languages. From each selected article we extracted the first ten sentences. Based on one fact mentioned in these sentences, human annotators filtered and corrected question and answer pairs that were generated by Gemini. The annotators, each native in the relevant language, first made sure that the question is answerable in a closed book setting, i.e., it does not refer explicitly to the surrounding context in the Wikipedia article, nor does it mention the answer. Second, they validated that the question is related to information that is particularly salient for the speakers of the language in question, and less related to general knowledge, like science or current events. Questions and answers that did not meet these criteria were discarded. Third, in a process called decontextualization, the annotators confirmed that the question contains all the information needed to be answerable when translated. For example, a question in Hebrew relating to the "supreme court" was disambiguated by the annotators to explicitly mention "the Israeli supreme court". Named entities were also clarified similarly, so a question referring to "Ambev" was modified to refer to "the Brazilian brewing company, Ambev".
Finally, each retained question and answer were automatically translated into the other 11 languages. The translations were verified by another set of human annotators and modified when needed. At this stage, some examples were also discarded if they proved to be untranslatable — for example, when a question explicitly refers to the meaning of a word in the source language.
Based on this approach, the final ECLeKTic dataset consists of 384 unique questions and 4224 translated examples.
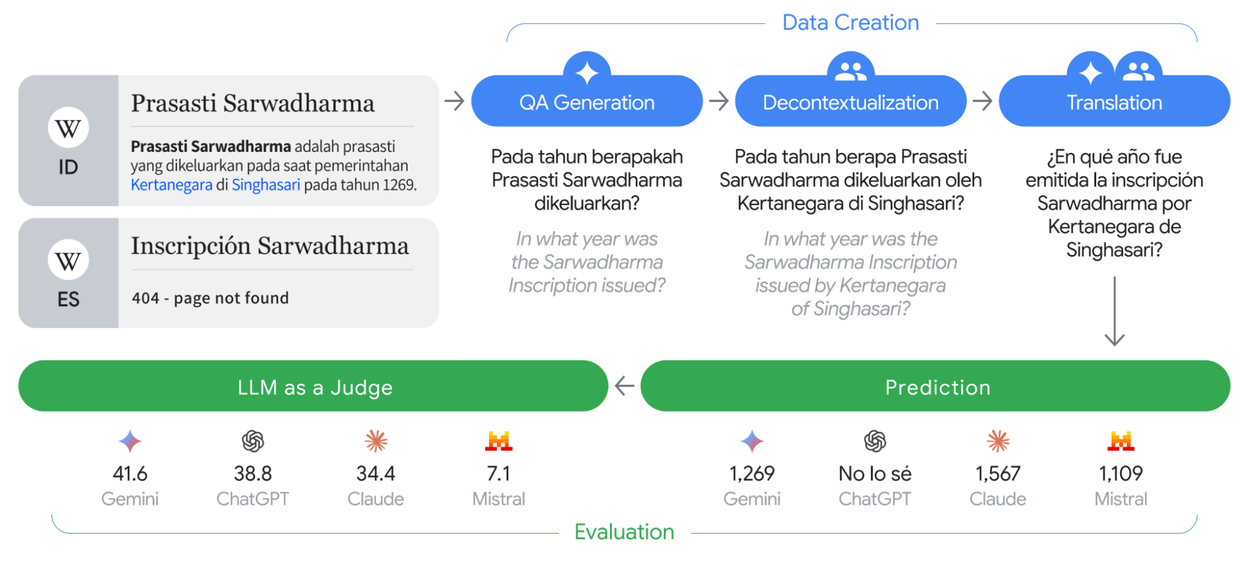
Illustration of the data creation for ECLeKTic and the evaluation of various models.
We also defined two metrics to measure the models’ success on ECLeKTic. The first and main one is the overall success; it is the share of questions that the model managed to answer correctly both in their original source language and in a target language. In addition, we report performance in terms of the transfer score, which is the share of questions that the model answered correctly in a target language only out of those that were answered correctly in their source language. This metric is more generous to models that answer fewer questions correctly in their source language.
Evaluation
The evaluation of models with ECLeKTic is done in a zero-shot setting, providing the model only with the question and no examples or surrounding context. When applied to state-of-the-art models, Gemini 2.0 Pro and Flash, GPT-4o, Claude 3.5 Sonnet, Gemma 2 9B, Mistral Nemo, Qwen 2.5 7B, and Olmo 2 7B, the evaluation showed that proprietary models can transfer knowledge far better than open ones, and that Gemini 2.0 is the best of the bunch, with 41.6% overall success and 65.0% transfer score.
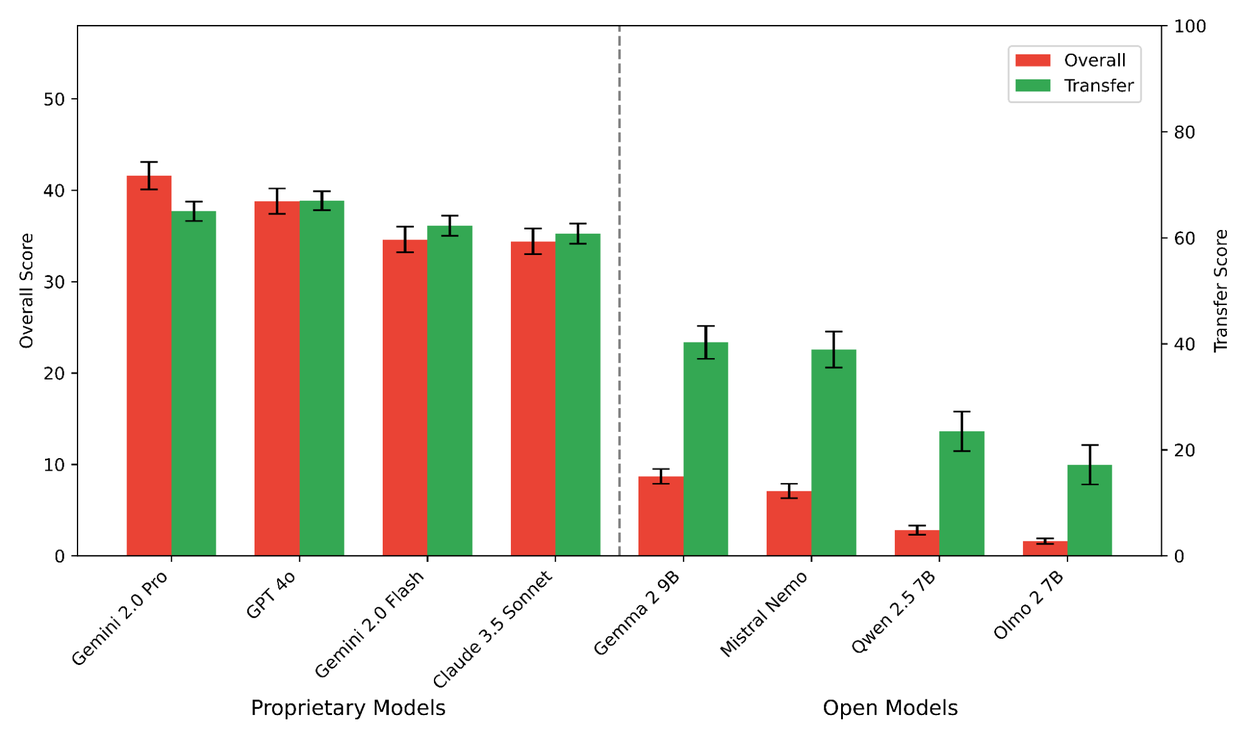
Performance for all proprietary and open models over all examples in ECLEKTIC in both metrics.
With the recent release of Gemini 2.5 Pro the performance improved even further. This latest model achieves an impressive 52.6% score in terms of overall success and 77.0% transfer score. It continues the trend of better multilingual capabilities with every new Gemini release.
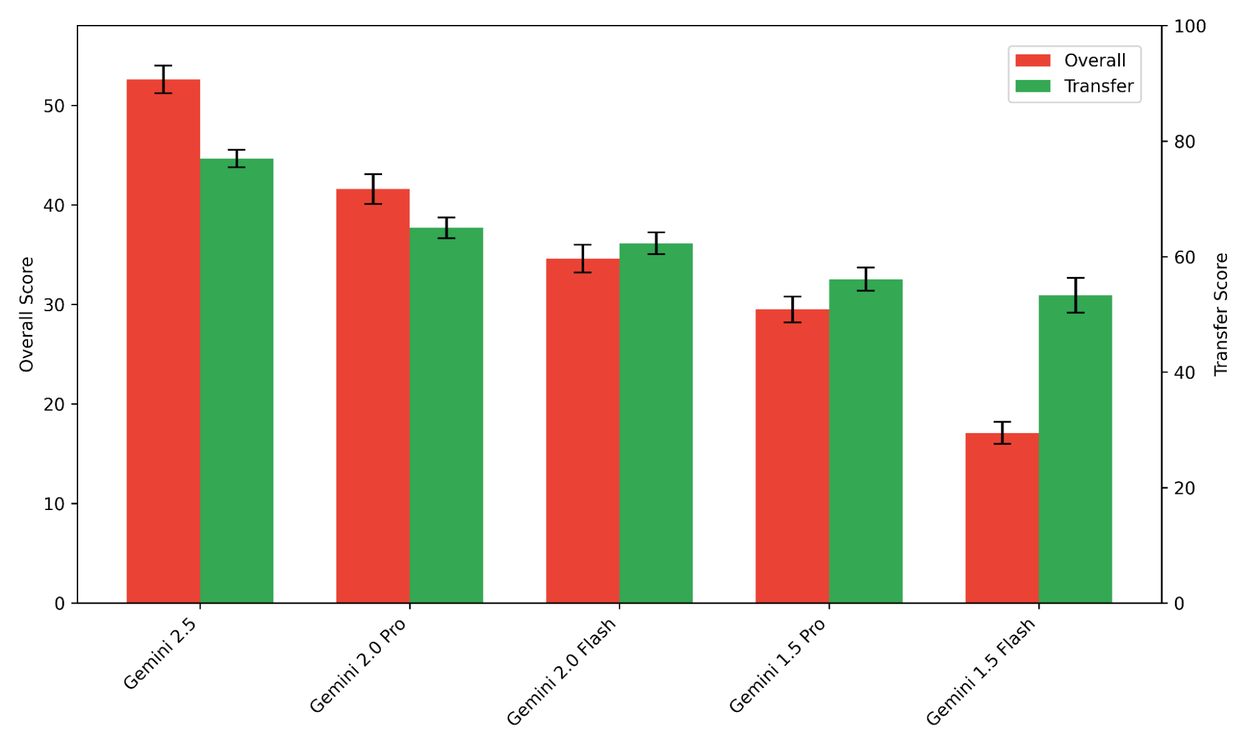
Performance for all available Gemini models over ECLEKTIC in both metrics. The upward trend is apparent.
We also broke down the results by source and target language to show how well models share knowledge between specific language pairs. For that we used the transfer score, which ignores the success in the source language itself. As shown in the figure below, the ability to transfer knowledge is not evenly distributed. Presumably, the main factor in enabling transfer is a shared writing system. Transfer between Latin script languages, such as German (de) and Italian (it), is better than transfer between Latin- and non-Latin script languages, such as Portuguese (pt) and Japanese (ja). The relatively good transfer scores for Indonesian (id), which is not a European language but is written in Latin script, suggests that script is more important than language family. This finding is in line with similar indications from previous studies by other researchers [1, 2, 3].
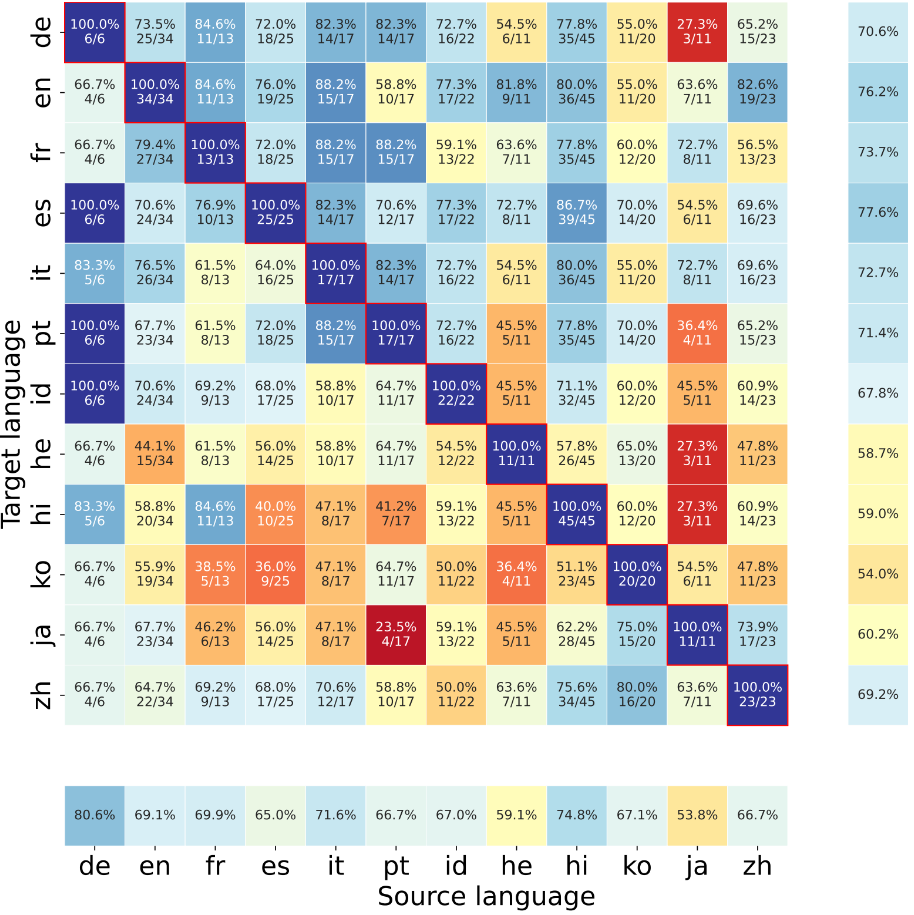
Transfer score results of Gemini 2.0 Pro broken down per source and target language. Blue is better, red is worse. Note the diagonal is perfect by the definition of the transfer metric.
Parting thoughts
By releasing ECLeKTic, we hope to provide a tool that allows LLM developers to assess and improve their models’ cross-lingual knowledge transfer capabilities. We place a high value on equitable, inclusive, and accurate language technology. The headroom remaining on ECLeKTic demonstrates that there is much work to be done to ensure that knowledge, cultural insights, and historical information encoded in any language is accessible to all LLM users.
Acknowledgements
The research presented here was conducted in cooperation with multiple researchers in Google Research and Google DeepMind: Dan Malkin, Sivan Eiger, Avinatan Hassidim, Yossi Matias, Joshua Maynez, Adi Mayrav Gilady, Jason Riesa, Shruti Rijhwani, Laura Rimell, Idan Szpektor, Reut Tsarfaty and Matan Eyal.