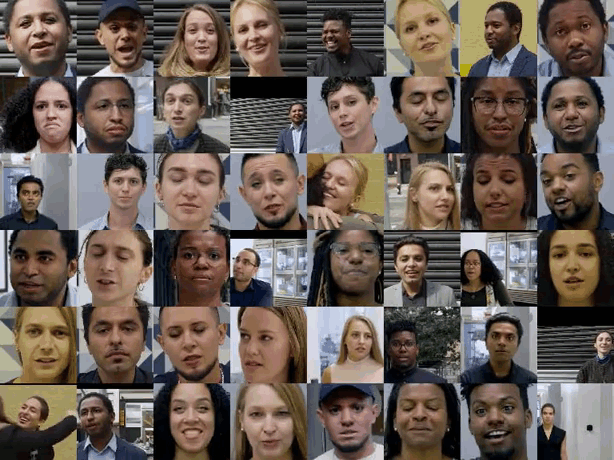
Contributing Data to Deepfake Detection Research
September 24, 2019
Posted by Nick Dufour, Google Research and Andrew Gully, Jigsaw
Quick links
Deep learning has given rise to technologies that would have been thought impossible only a handful of years ago. Modern generative models are one example of these, capable of synthesizing hyperrealistic images, speech, music, and even video. These models have found use in a wide variety of applications, including making the world more accessible through text-to-speech, and helping generate training data for medical imaging.
Like any transformative technology, this has created new challenges. So-called "deepfakes"—produced by deep generative models that can manipulate video and audio clips—are one of these. Since their first appearance in late 2017, many open-source deepfake generation methods have emerged, leading to a growing number of synthesized media clips. While many are likely intended to be humorous, others could be harmful to individuals and society.
Google considers these issues seriously. As we published in our AI Principles last year, we are committed to developing AI best practices to mitigate the potential for harm and abuse. Last January, we announced our release of a dataset of synthetic speech in support of an international challenge to develop high-performance fake audio detectors. The dataset was downloaded by more than 150 research and industry organizations as part of the challenge, and is now freely available to the public.
Today, in collaboration with Jigsaw, we're announcing the release of a large dataset of visual deepfakes we've produced that has been incorporated into the Technical University of Munich and the University Federico II of Naples’ new FaceForensics benchmark, an effort that Google co-sponsors. The incorporation of these data into the FaceForensics video benchmark is in partnership with leading researchers, including Prof. Matthias Niessner, Prof. Luisa Verdoliva and the FaceForensics team. You can download the data on the FaceForensics github page.
Acknowledgements
Special thanks to all our team members and collaborators who work on this project with us: Daisy Stanton, Per Karlsson, Alexey Vorobyov, Thomas Leung, Jeremiah "Spudde" Childs, Avneesh Sud, Christoph Bregler, Andreas Roessler, Davide Cozzolino, Justus Thies, Luisa Verdoliva, Matthias Niessner, and the hard-working actors and film crew who helped make this dataset possible.