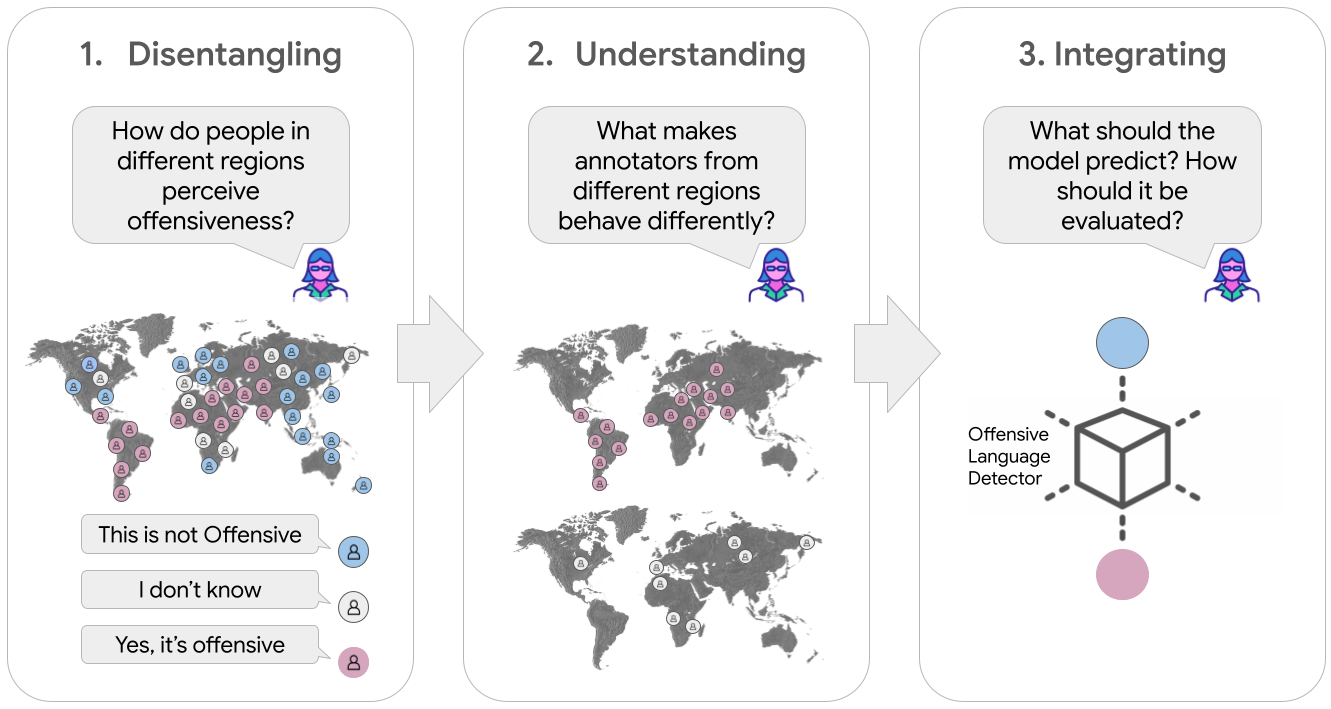
Building AI for the pluralistic society
February 13, 2025
Aida Davani, Research Scientist, and Vinodkumar Prabhakaran, Staff Research Scientist
Building AI for a pluralistic world demands us to move beyond monolithic models of human perspectives, and embrace the tapestry of diverse values and viewpoints, building effective ways to disentangle, understand, and integrate them into different parts of the AI development pipeline.
Modern artificial intelligence (AI) systems rely on input from people. Human feedback helps train models to perform useful tasks, guides them toward safe and responsible behavior, and is used to assess their performance. While hailing the recent AI advancements, we should also ask: which humans are we actually talking about? For AI to be most beneficial, it should reflect and respect the diverse tapestry of values, beliefs, and perspectives present in the pluralistic world in which we live, not just a single "average" or majority viewpoint. Diversity in perspectives is especially relevant when AI systems perform subjective tasks, such as deciding whether a response will be perceived as helpful, offensive, or unsafe. For instance, what one value system deems as offensive may be perfectly acceptable within another set of values.
Since divergence in perspectives often aligns with socio-cultural and demographic lines, preferentially capturing certain groups’ perspectives over others in data may result in disparities in how well AI systems serve different social groups. For instance, we previously demonstrated that simply taking a majority vote from human annotations may obfuscate valid divergence in perspectives across social groups, inadvertently marginalizing minority perspectives, and consequently performing less reliably for groups marginalized in the data. How AI systems should deal with such diversity in perspectives depends on the context in which they are used. However, current models lack a systematic way to recognize and handle such contexts.
With this in mind, here we describe our ongoing efforts in pursuit of capturing diverse perspectives and building AI for the pluralistic society in which we live. We start with understanding the varying perspectives in the world and, ultimately, we develop effective ways to integrate these differences into the modeling pipeline. Each stage of the AI development pipeline — from conceptualization and data collection to training, evaluation, and deployment — offers unique opportunities to embed diverse perspectives, but also presents distinct challenges. A truly pluralistic AI cannot rely on isolated fixes or adjustments; it requires a holistic, layered approach that acknowledges and integrates complexity at every step. Having scalability in mind, we set out to (1) disentangle systematic differences in perspectives across social groups, (2) develop an in-depth understanding of the underlying causes for these differences, and (3) build effective ways to integrate meaningful differences into the machine learning (ML) modeling pipeline.
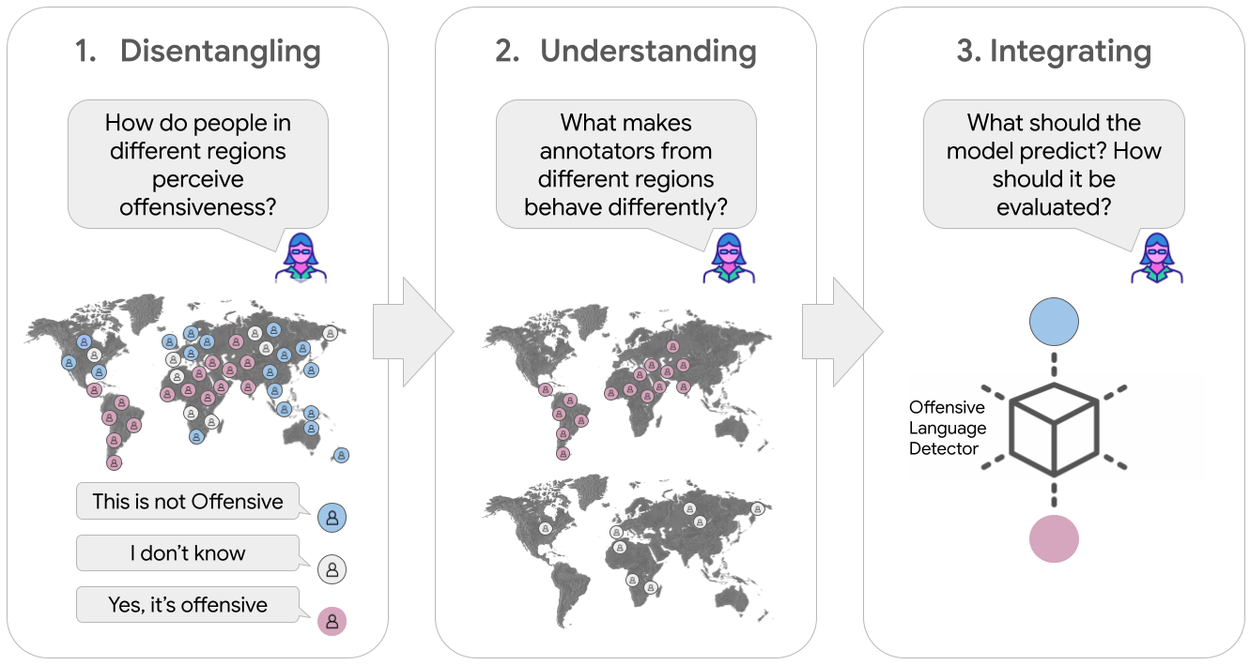
A holistic, layered approach to (1) disentangle systematic differences in perspectives across social groups, (2) develop an in-depth understanding of the underlying causes for these differences, and (3) build effective ways to integrate meaningful differences into the ML modeling pipeline. We use offensive language detection as an example task for demonstration, but the ideas presented here extend to any subjective task with varying human perspectives.
Over the last three years, we have made several advances along all of these three fronts:
Disentangling diverse perspectives
Our efforts began with a call for action paper in 2021 in which we identified an issue in annotated datasets. Previous annotation methods, where only the majority vote of the annotator labels were made public, can lead to data that is inherently biased and unrepresentative of the breadth of users. Our research demonstrated that this reduction may unfairly disregard perspectives of certain annotators, and sometimes certain socio-demographic groups. We concluded that annotators are not interchangeable — that is, they draw from their socially embedded experiences and knowledge when making annotation judgments. As a result, retaining their perspectives separately in the datasets will enable dataset users to account for these differences according to their needs.
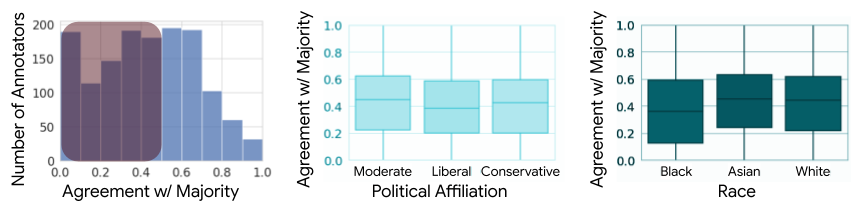
Agreement between annotators and the majority label. The figure on the left illustrates distribution of annotators’ agreement with the majority vore in a sentiment detection (Díaz, et al., 2018) The figures in the center and on the right present two boxplots of annotators’ agreement with the majority label, grouped by race and political orientation. A key finding is that majority label agreement was significantly lower among annotators who identified as Black.
Following these findings, our team took on the challenge of building more inclusive datasets. We collected and published large-scale datasets aimed at capturing diverse perspectives across various socio-demographic subgroups.
Two examples are D3CODE and DICES, both of which centered on detecting offensive and harmful language. The D3CODE dataset is a large-scale cross-cultural dataset that captures parallel annotations for offensive language in over 4.5k sentences annotated by a pool of over 4k annotators, balanced across gender and age, from across 21 countries, representing eight geo-cultural regions. The DICES dataset captures conversational safety ratings on around 1k sentences from a diverse pool of raters along gender, race, and age. The dataset is unique in providing a thorough set of labels for each individual post.
Analyzing D3CODE and DICES revealed that annotator diversity is not just about individual differences, but also about the patterns of agreement and disagreement that emerge within and between groups. To quantify and identify patterns of agreement within groups, we introduced the GRASP metrics, a novel method for reliably measuring statistically significant group level associations in perspectives. When applied to an annotated dataset, the GRASP metric calculates a particular group’s congruency by comparing internal agreements with the level of agreement observed with outgroups.
These datasets and metrics enable new lines of exploratory research to tease apart important distinctions in perspectives: e.g., research on intersectionality in AI safety shows that South Asian women are 53% less likely than White men to rate a conversation safe, and a cross-cultural analysis of offensive language annotations shows that raters in the Global South tend to be more sensitive towards recognizing offensiveness in text.
Understanding the underlying factors
While detecting group-level differences is an important first step, meaningful interventions require us to develop an in-depth understanding of what factors contribute to these differences in perspectives and why these perspectives diverge. This exploration often necessitates a multidisciplinary lens, drawing insights from various fields, such as psychology and sociology.
Consider the example of offensive language detection; research in moral and social psychology reveals that individuals’ judgments of offensive language can be rooted in their personal values and beliefs as well as social norms of their society about what is right or wrong. By understanding these underlying sets of values, we can gain a more nuanced understanding of why different groups and individuals might disagree on what constitutes offensive language.
In our recent research, we employed the social psychology framework of moral foundations theory, which posits moral beliefs across six foundations: Care, Equality, Proportionality, Authority, Loyalty and Purity. We specifically relied on the moral foundations questionnaire (MFQ-2), because it was developed and validated through extensive cross-cultural assessments of moral judgments, making it a reliable tool for integrating a pluralistic definition of values into AI research.
Our experiment demonstrated that cross-cultural differences in perceptions of offensiveness are significantly mediated by individual moral concerns, in particular, Care and Purity. In other words, cultures that place a higher weight on the value of caring for other individuals for other individuals and avoiding impure thoughts are more sensitive to offensive language. These insights provide more meaningful theoretical grounding for data and model developers in their pursuit of aligning AI systems with human values.
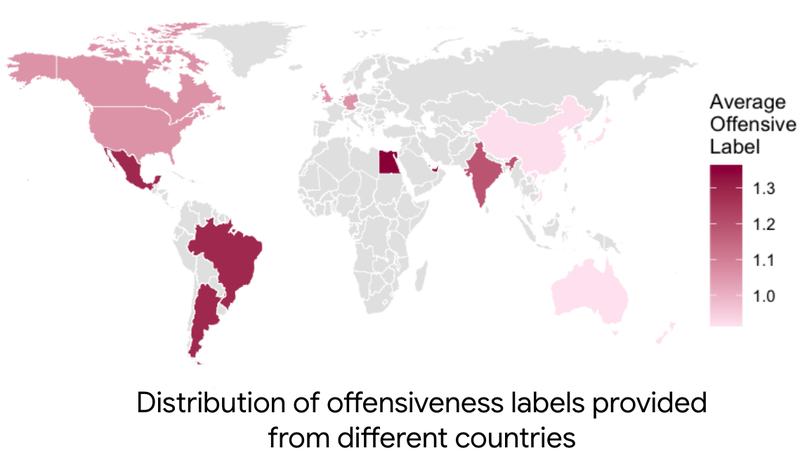
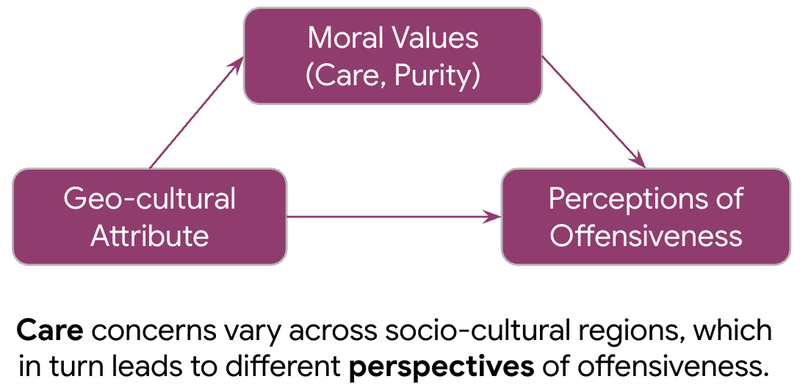
This research underscores the importance of the why behind diverging human perspectives. By identifying moral values as the grounding factor in shaping different attitudes toward offensive language detection, we can move beyond acknowledging the presence of such disagreement to actually anticipating and accounting for them in the design of AI systems.
This brings us to the next crucial step: how can we integrate our understanding of pluralism into our AI models? How can we build models that are not only aware of diverse perspectives but also capable of effectively incorporating them into their decision-making process?
Integrating pluralism in data and models
We have also spearheaded research on how to incorporate such diverse perspectives in ML data and model development and evaluation pipelines. For instance, we recently demonstrated how we can use our metrics to dynamically determine rater groups relevant for any given task. Then we can perform targeted diversification during data annotations by effectively identifying and involving annotators from specific social groups with unique perspectives to ensure representation of various viewpoints. Through simulation experiments, we observed that such an approach can efficiently increase recall of safety issues flagged by minoritized rater groups without hurting overall precision. We have also performed pioneering work on multi-perspective modeling that efficiently learns a shared understanding of the problem at hand, while also catering to specific social groups with distinct perspectives in predictions. This approach also enables models to discern value-laden inputs that can have a variety of correct answers.
Conclusion
Our body of research reveals the importance of accounting for pluralism in AI model development and deployment, and builds tools and resources to further study and innovate on novel ways to incorporate pluralism in AI. It responds to the fact that global society is pluralistic. But the question of whether we want AI to be pluralistic — i.e., always adapting to local values, is a more nuanced question. For instance, in a paper published in 2022, we discussed how certain universal baseline values, such as the principles of universal human rights, may sometimes be desirable. Ultimately, we aspire towards AI that is controllable — i.e., having the ability to control when, where, and how specific values and perspectives are adopted and extensible — is able to adapt to emergent perspectives as we encounter new cultural contexts, or societal change over time, and is transparent about what values guide a particular instantiation.
Acknowledgements
We would like to thank everyone on the team that contributed to the work presented in this blog post (in alphabetical order by last name): Lora Aroyo, Dylan Baker, Mark Díaz, Christopher Homan, Alicia Parrish, Charvi Rastogi, Greg Serapio-Garcia, Alex Taylor, Ding Wang, and Chris Welty.