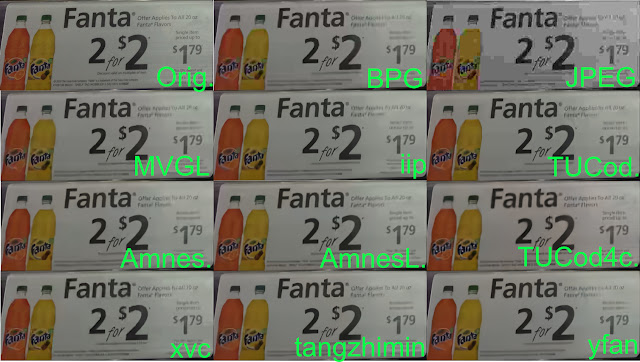
Announcing the Second Workshop and Challenge on Learned Image Compression
February 6, 2019
Posted by Nick Johnston, Software Engineer, Machine Perception
Last year, we announced the Workshop and Challenge on Learned Image Compression (CLIC), an event that aimed to advance the field of image compression with and without neural networks. Held during the 2018 Computer Vision and Pattern Recognition conference (CVPR 2018), CLIC was quite a success, with 23 accepted workshop papers, 95 authors and 41 entries into the competition. This spawned many new algorithms for image compression, domain specific applications to medical image compression and augmentations to existing methods based, with the winner Tucodec (abbreviated TUCod4c in the image below) achieving 13% better mean opinion score (MOS) than Better Portable Graphics (BPG) compression.
Workshop and Challenge on Learned Image Compression at CVPR 2019 in Long Beach, California.The half day workshop will feature talks from invited guests Anne Aaron (Netflix), Aaron Van Den Oord (DeepMind) and Jyrki Alakuijala (Google), along with presentations from five top performing teams in the 2019 competition, which is currently open for submissions.
This year's competition features two tracks for participants to compete in. The first track remains the same as last year, in what we're calling the "low-rate compression" track. The goal for low-rate compression is to compress an image dataset to 0.15 bits per pixel and maintaining the highest quality metrics as measured by PSNR, MS-SSIM and a human evaluated rating task.
The second track incorporates feedback from last year's workshop, in which participants expressed interest in the inverse challenge of determining the amount an image could be compressed and still look good. In this "transparent compression" challenge, we set a relatively high quality threshold for the test dataset (in both PSNR and MS-SSIM) with the goal of compressing the dataset to the smallest file sizes.
If you're doing research in the field of learned image compression, we encourage you to participate in CLIC during CVPR 2019. For more details on the competition and dates, please refer to compression.cc.
Acknowledgements
This workshop is being jointly hosted by researchers at Google, Twitter and ETH Zürich. We'd like to thank: George Toderici (Google), Michele Covell (Google), Johannes Ballé (Google), Nick Johnston (Google), Eirikur Agustsson (Google), Wenzhe Shi (Twitter), Lucas Theis (Twitter), Radu Timofte (ETH Zürich), Fabian Mentzer (ETH Zürich) for their contributions.