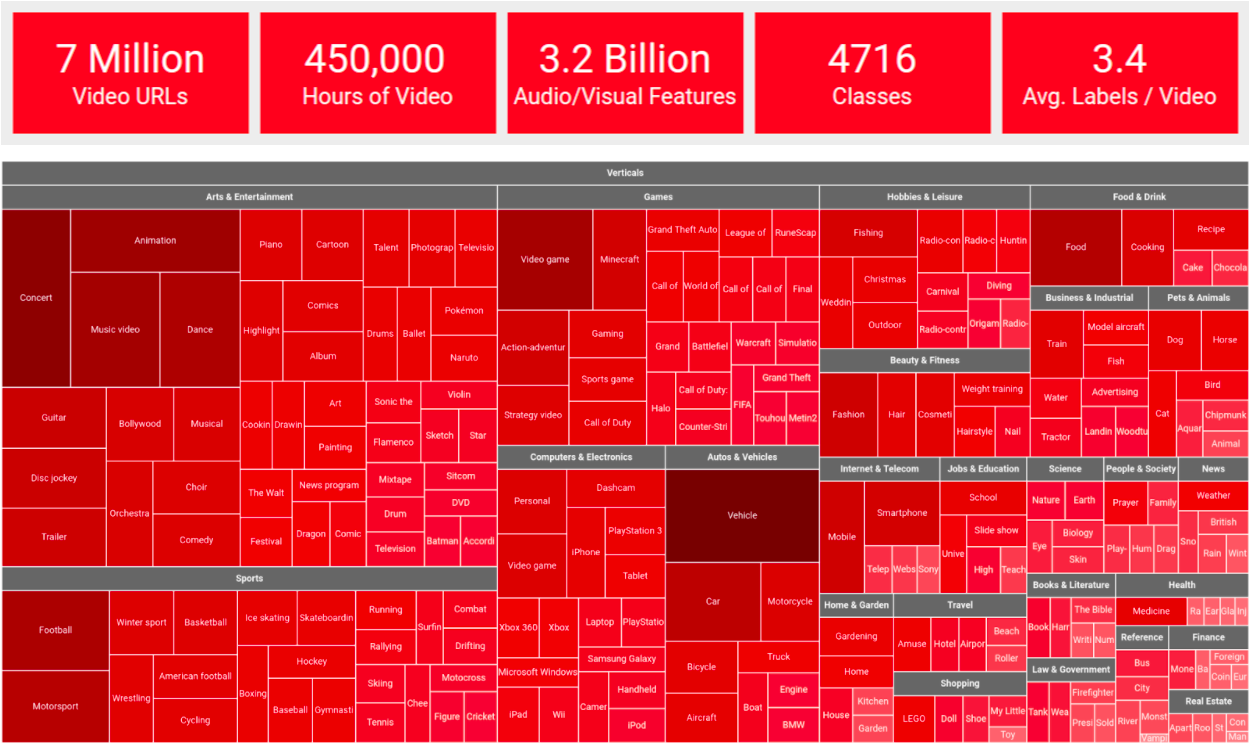
An updated YouTube-8M, a video understanding challenge, and a CVPR workshop. Oh my!
February 15, 2017
Posted by Paul Natsev, Software Engineer
Quick links
Last September, we released the YouTube-8M dataset, which spans millions of videos labeled with thousands of classes, in order to spur innovation and advancement in large-scale video understanding. More recently, other teams at Google have released datasets such as Open Images and YouTube-BoundingBoxes that, along with YouTube-8M, can be used to accelerate image and video understanding. To further these goals, today we are releasing an update to the YouTube-8M dataset, and in collaboration with Google Cloud Machine Learning and kaggle.com, we are also organizing a video understanding competition and an affiliated CVPR’17 Workshop.
An Updated YouTube-8M
The new and improved YouTube-8M includes cleaner and more verbose labels (twice as many labels per video, on average), a cleaned-up set of videos, and for the first time, the dataset includes pre-computed audio features, based on a state-of-the-art audio modeling architecture, in addition to the previously released visual features. The audio and visual features are synchronized in time, at 1-second temporal granularity, which makes YouTube-8M a large-scale multi-modal dataset, and opens up opportunities for exciting new research on joint audio-visual (temporal) modeling. Key statistics on the new version are illustrated below (more details here).
![]() |
A tree-map visualization of the updated YouTube-8M dataset, organized into 24 high-level verticals, including the top-200 most frequent entities, plus the top-5 entities for each vertical. |
![]() |
Sample videos from the top-18 high-level verticals in the YouTube-8M dataset. |
We are also excited to announce the Google Cloud & YouTube-8M Video Understanding Challenge, in partnership with Google Cloud and kaggle.com. The challenge invites participants to build audio-visual content classification models using YouTube-8M as training data, and to then label ~700K unseen test videos. It will be hosted as a Kaggle competition, sponsored by Google Cloud, and will feature a $100,000 prize pool for the top performers (details here). In order to enable wider participation in the competition, Google Cloud is also offering credits so participants can optionally do model training and exploration using Google Cloud Machine Learning. Open-source TensorFlow code, implementing a few baseline classification models for YouTube-8M, along with training and evaluation scripts, is available at Github. For details on getting started with local or cloud-based training, please see our README and the getting started guide on Kaggle.
The CVPR 2017 Workshop on YouTube-8M Large-Scale Video Understanding
We will announce the results of the challenge and host invited talks by distinguished researchers at the 1st YouTube-8M Workshop, to be held July 26, 2017, at the 30th IEEE Conference on Computer Vision and Pattern Recognition (CVPR 2017) in Honolulu, Hawaii. The workshop will also feature presentations by top-performing challenge participants and a selected set of paper submissions. We invite researchers to submit papers describing novel research, experiments, or applications based on YouTube-8M dataset, including papers summarizing their participation in the above challenge.
We designed this dataset with scale and diversity in mind, and hope lessons learned here will generalize to many video domains (YouTube-8M captures over 20 diverse video domains). We believe the challenge can also accelerate research by enabling researchers without access to big data or compute clusters to explore and innovate at previously unprecedented scale. Please join us in advancing video understanding!
Acknowledgements
This post reflects the work of many others within Machine Perception at Google Research, including Sami Abu-El-Haija, Anja Hauth, Nisarg Kothari, Joonseok Lee, Hanhan Li, Sobhan Naderi Parizi, Rahul Sukthankar, George Toderici, Balakrishnan Varadarajan, Sudheendra Vijayanarasimhan, Jiang Wang, as well as Philippe Poutonnet and Mike Styer from Google Cloud, and our partners at Kaggle. We are grateful for the support and advice from many others at Google Research, Google Cloud, and YouTube, and especially thank Aren Jansen, Jort Gemmeke, Dan Ellis, and the Google Research Sound Understanding team for providing the audio features in the updated dataset.