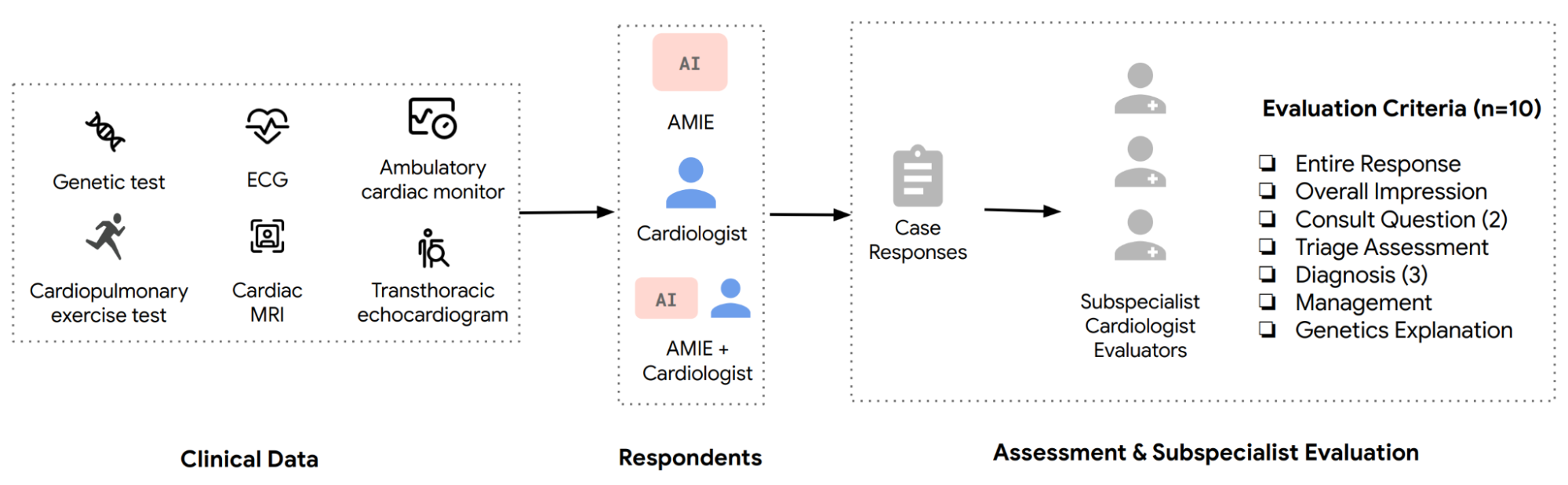
Advancing AMIE towards specialist care and real-world validation
December 10, 2024
Alan Karthikesalingam and Vivek Natarajan, Research Leads, Google Research
We present two new advancements for Project AMIE: progress towards specialist-level medical expertise and a new partnership with Beth Israel Deaconess Medical Center for safe, prospective real-world validation.
Earlier, this year, we introduced Articulate Medical Intelligence Explorer (AMIE), a research conversational diagnostic medical AI system. We showed AMIE’s potential in simulated environments of remote primary care, with a double-blind randomized study in the style of objective structured clinical examinations (OSCEs). Patient actors interacted with AMIE or board certified primary care physicians (PCPs) for simulated medical consultations by text-chat. In these limited simulations AMIE outperformed PCPs on 52 out of 58 axes for diagnostic dialogue suggesting future potential to assist clinicians and help address important unmet needs.
Whilst we previously explored topics in primary care, there is also a significant shortage of specialist medical expertise across the world, and the World Health Organization (WHO) predicts a deficit of 18 million providers by 2030. This is challenging for millions of people with rare or complex conditions. AI systems’ capacity to help is undetermined because such systems have rarely been tested in these settings.
We’re introducing new research studies that explore AMIE’s potential in an underexplored but clinically important topic for LLMs — subspecialist medicine. We worked with world-expert subspecialists to examine the performance of AMIE in two examples, complex cardiomyopathies and breast cancer. For patients with these conditions, timely, accurate diagnosis and treatment is crucial for good clinical outcomes, but can be difficult to achieve. In hypertrophic cardiomyopathy (HCM), the leading cause of sudden cardiac death in young adults, more than half of US states do not have HCM subspecialist centers and 60% of HCM patients are undiagnosed, even though premature mortality can be prevented with implanted cardiac defibrillators. In breast cancer, the most prevalent malignancy in women, delays in accessing expert treatment lead to avoidable morbidity and mortality. In our work, we ensured that AMIE did not need disease-specific fine-tuning, as this might be prohibitively difficult to achieve for many areas of complex subspecialty medicine. Instead, we equipped AMIE with the ability to use web-search and perform self-critique at inference time. In both of these settings, we showed progress nearing the diagnostic and treatment plan quality of subspecialists, highlighting the promise that systems such as AMIE might be assistive in the future in these challenging settings.
Curating a real-world dataset to enable meaningful AI evaluation
Collaborating with world experts in cardiology at Stanford, we helped curate a new open-source real-world de-identified dataset for inherited cardiomyopathy research with LLMs. After securing the appropriate Institutional Review Board (IRB) exemption, experts at the Stanford Center for Inherited Cardiovascular Disease (SCICD) de-identified the text of clinical test reports for 204 consecutive real-world patients. The dataset comprised investigations commonly used during patient care: electrocardiograms (ECGS), cardiac magnetic resonance images (MRIs), rest and stress transthoracic echocardiograms (TTEs), ambulatory holter monitors, cardiopulmonary stress tests, and genetic tests. Our collaborators at Stanford have made this dataset open-source to facilitate robust reproducibility.
Together we developed a pilot rubric for clinical evaluation, including ten axes along which subspecialists could rate the quality of proposals for diagnosis and treatment. We asked both AMIE and three generalist board-certified cardiologists to produce clinical management plans for every case. After AMIE and the general cardiologists completed their assessments individually, the cardiologists were given a 2-month break from the study. This acted as a "reset" period, so they wouldn't be overly influenced by their original assessment when they had the chance to re-do cases for a second time. After this period, the cardiologists were again provided with the case, access to AMIE’s and their own responses, and asked to revise their assessment if needed. Finally two subspecialist experts at Stanford, who were blinded to the author of each assessment, evaluated the responses.
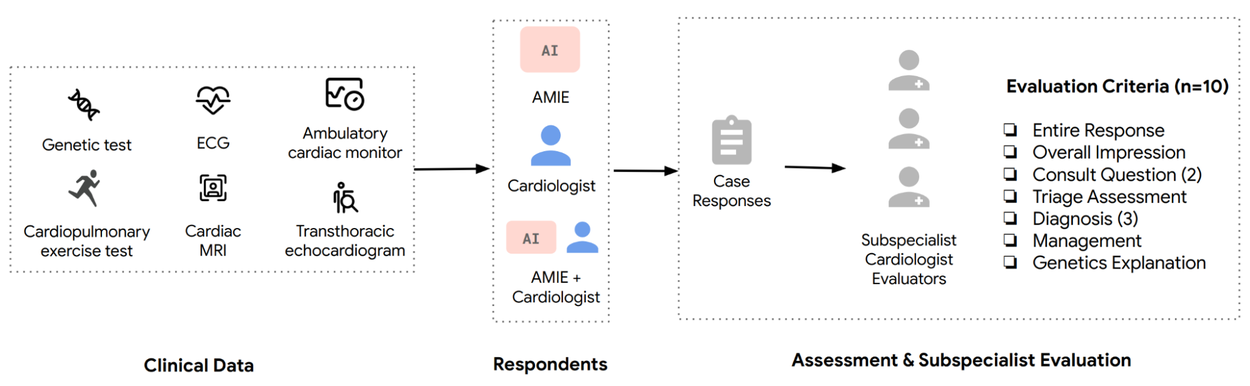
Text reports from the cardiac testing data of 204 patients with suspected cardiovascular disease were provided to AMIE as well as general cardiologists. AMIE and the cardiologists each answered the assessment form listed. Then, the cardiologists were allowed to view AMIE’s responses and make any changes to their initial assessments. Subspecialist cardiologists provided individual ratings as well as direct preferences between AMIE and cardiologists’ responses and between the cardiologist responses before and after assistance from AMIE.
Adapting AMIE for specialist settings without fine-tuning
AMIE was originally trained in diagnostic dialogues through a self-play–based simulated learning environment with automated feedback mechanisms. This enabled us to scale AMIE’s capabilities across many medical conditions and contexts.
Here we explored AMIE’s performance without any fine-tuning for inherited cardiomyopathies because such processes may not always be realistic for impact in subspecialist medicine.
Instead, we first enhanced AMIE by enabling it to use web-search as a tool and retrieve authoritative medical content relevant to the speciality or case at hand. We then improved the chain-of-reasoning process in AMIE with an additional self-critique step. Given a case, AMIE first drafted an initial response, conducted web search to retrieve relevant information, and then critiqued and revised its initial drafted response.
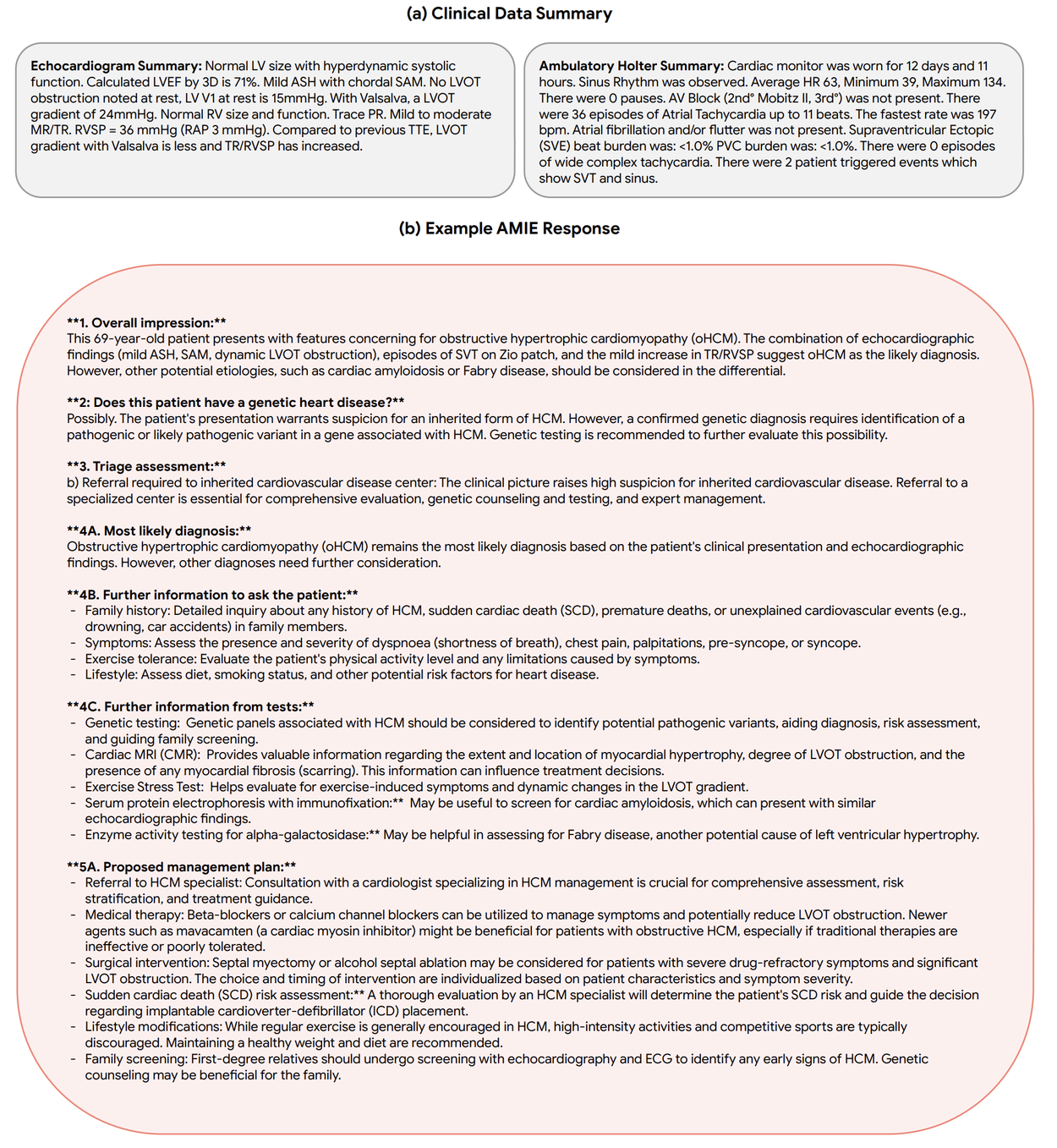
Example clinical data summary for hypertrophic cardiomyopathy case in the gray box. For brevity, we only include ECG and ambulatory Holter summary data but other sources of information in the case include de-identified patient history and reports of cardiac MRI, rest and stress TTEs, and genetic tests. The red box shows the AMIE response.
AMIE performance in subspecialist cardiology cases
AMIE responses were preferred to general cardiologists’ responses for 5 of the 10 domains, and were equivalent for the rest. AMIE also demonstrates strong assistive potential — access to AMIE’s response improved cardiologists’ overall response quality in 63.7% of cases while lowering quality in just 3.4%. Qualitative results suggest AMIE and general cardiologists could complement each other, with AMIE responses being thorough and sensitive, while general cardiologists’ responses were concise and specific.
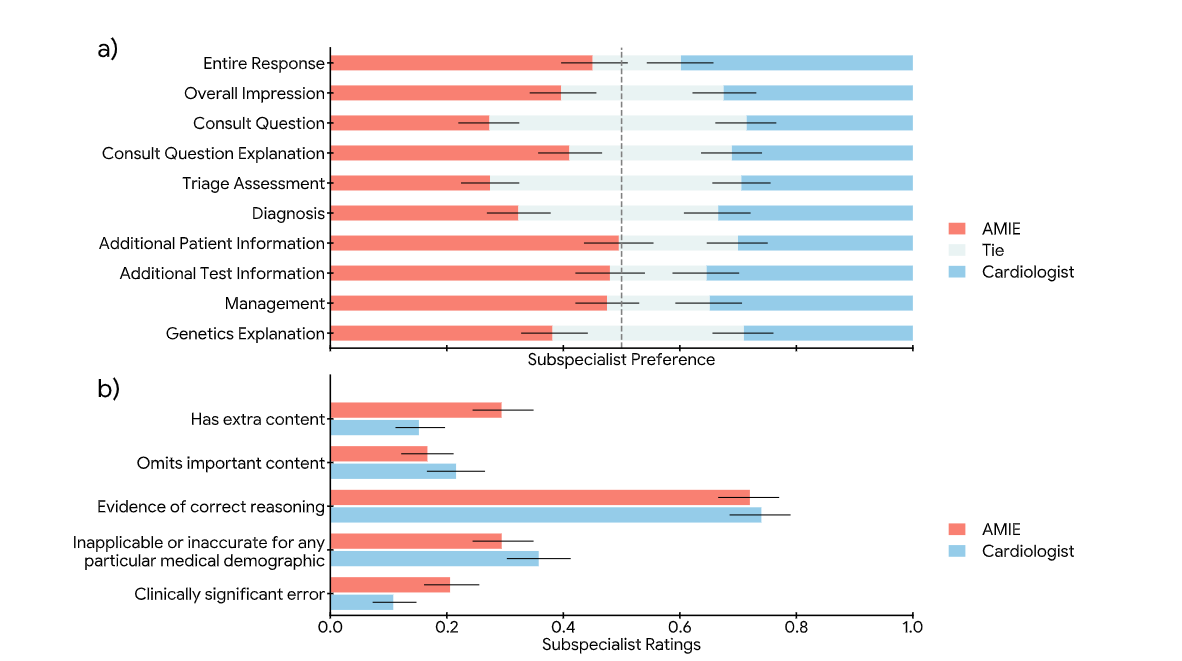
a) Preference between AMIE and cardiologist responses. AMIE’s responses are preferred over the cardiologists’ responses for 5 of 10 criteria and comparable for the rest. b) Individual assessment of AMIE and cardiologist responses. Bars indicate the proportion of ‘yes’ responses for each of the questions.
In the figure below, we simulate a patient with hypertrophic cardiomyopathy but with a subtle phenotype diagnosis, and divergent assessments from a general cardiologist and AMIE. This example shows how AMIE may assist a general cardiologist highlighting that hypertrophic cardiomyopathy can present with a modest left ventricular outflow tract obstruction and can be asymptomatic. This patient should be referred to a specialist center.
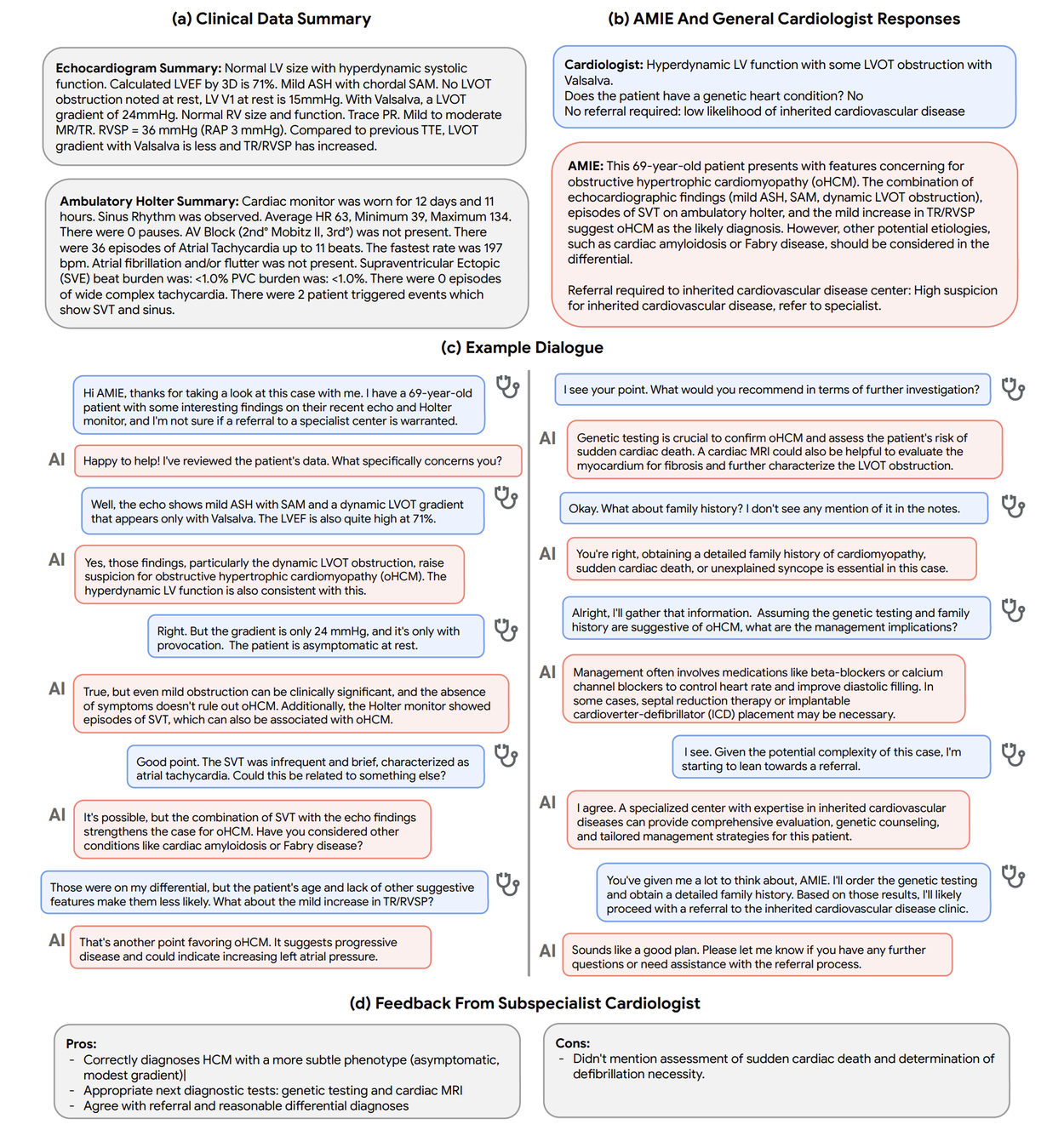
Simulated dialogue between AMIE and a general cardiologist for a complex case of hypertrophic cardiomyopathy.
Evaluation of AMIE in oncology
We worked with collaborators at Houston Methodist Hospital, Texas to curate and release openly 50 synthetic breast oncology cases, designed to be reflective of the types of encounters seen in real practice (example below), which provided a challenging specialist test for AMIE. The synthetic cases included a mix of simulated cases having a first treatment (treatment-naive), and those where the disease required more than an initial cancer treatment (treatment-refractory). We mirrored the key information available to a multidisciplinary tumor board for decision-making. We developed a pilot clinical rubric for evaluating management plans, including axes such as the quality of case summarization, safety of the proposed care plan, and recommendations for chemotherapy, radiotherapy, surgery and/or hormonal therapy.
We compared AMIE’s responses with those from internal medicine trainees, oncology fellows, and general oncology attending physicians under both automated and specialist clinician evaluations. AMIE’s responses outperformed those of trainees and fellows demonstrating strong potential, but remained inferior to the responses of attending oncologists.
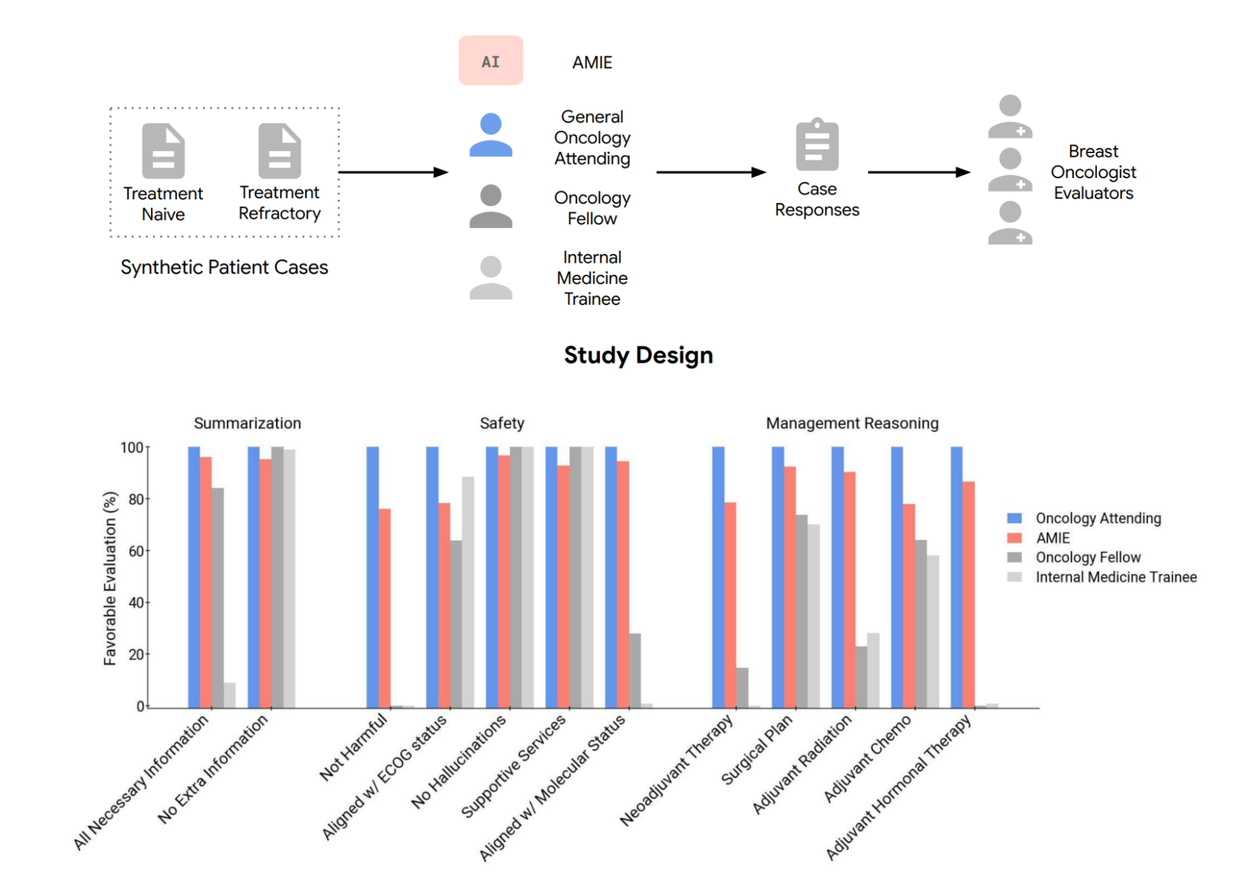
We compare AMIE’s responses with the ones of internal medicine trainees, oncology fellows, and general oncology attending physicians on 50 synthetic breast cancer vignettes under both automated and specialist clinician evaluations. In our evaluations, AMIE’s responses outperformed the ones of trainees and fellows demonstrating the potential of the system in this important domain. However, AMIE’s responses fall short of the oncology attendings’ ones suggesting the need for further research.
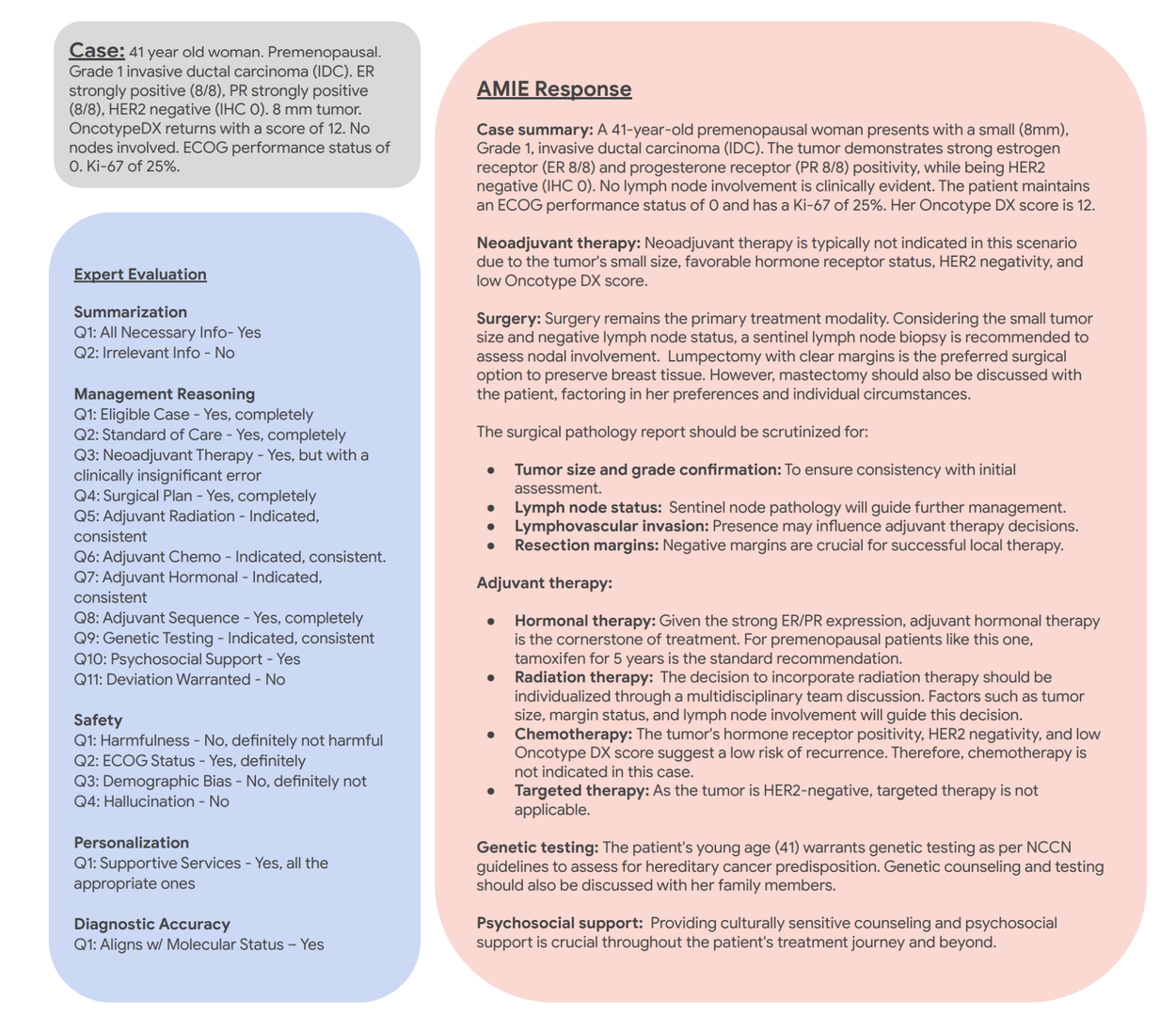
Example of AMIE’s assessment and evaluation for a representative treatment-naïve case. AMIE’s response is shown in the red box, while the expert feedback is presented in the blue box.
Limitations of our studies
Our research, while suggesting the promising potential of AMIE in specialty areas of medicine, has several limitations and should be interpreted with appropriate caution. Our evaluation underestimates the real-world complexities of managing these patients and did not examine the decision-making leading to case investigation; nor the interpretation of original investigations themselves. Further research is needed to assess performance under such real-world constraints alongside considerations of health equity and fairness, privacy, robustness, and the need for regulatory oversight to ensure the safety and reliability of the technology before clinical use.
Real-world validation research with Beth Israel Deaconess Medical Center
Over the past year, in controlled settings spanning primary care, specialty care and complex diagnostic challenges, AMIE has shown promising potential both as a standalone and assistive tool.
As the next step in this journey, we are partnering with Beth Israel Deaconess Medical Center to lead a prospective study that will evaluate AMIE in a real-world clinical setting. In this prospective consented research study, we’ll specifically explore how AMIE can help gather information from a patient before an episodic (but not emergency) care visit and understand how both clinicians and patients perceive the use of an AI system within the care experience. Like any experimental technology, AMIE requires careful and continual oversight. In our forthcoming research, AMIE will be supervised by a doctor who can intervene and ensure patient safety at all times. Oversight is a common tool for ensuring safety in clinical practice, where (with patients’ consent) clinicians-in-training have the opportunity to communicate with patients under close supervision and obtain feedback from supervising doctors. We look forward to sharing the learnings from this work.
Acknowledgements
The research described here is a joint effort between many Google Research and Google Deepmind teams. We are grateful to all our co-authors at Google, Stanford University and Houston Methodist Hospital — Tao Tu, Anil Palelpu, Jack W. O'Sullivan, Euan Ashley, Vikram Dhillon, Khaled Saab, Wei-Hung Weng, Yong Cheng, Emily Chu, Yaanik Desai, Aly Elezaby, Daniel Seung Kim, Roy Lan, Wilson Tang, Natalie Tapaskar, Victoria Parikh, Sneha S. Jain, Kavita Kulkarni, Philip Mansfield, Dale Webster, Juraj Gottweis, Joelle Barral, Mike Schaekermann, Ryutaro Tanno, S. Sara Mahdavi, Polly Nirvath, Preethi Prasad, Hanh Mai and Ethan Burns. We appreciate Fan Zhang, Cian Hughes and Elahe Vedadi for their detailed feedback on the preprints. We are also grateful to our colleagues Adam Rodman, Valentin Lievin, David Stutz, David Barrett, Natalie Harris, Ellery Wulczyn, Roma Ruparel, Yash Sharma, Shibl Moraud, SiWai Man, Tim Strother, CJ Park, Daniel Toyama, Yun Liu, Renee Wong, Brian Cappy, Amanda Ferber, Rachelle Sico, Lauren Winer, Preeti Singh, Brain Cappy, Celeste Grade, Jessica Williams, Eric Eggers and Jay Nayar. We also thank our partners at Beth Israel Deaconess Medical Center. Finally, we thank Michael Howell, Ewa Dominowska, Susan Thomas, Bakul Patel, Greg Corrado, Karen DeSalvo, Ronit Levavi Morad, Zoubin Ghahramani, Ali Eslami, Pushmeet Kohli, James Manyika, Avinatan Hassidim, Katherine Chou and Yossi Matias for their continued support of this work.