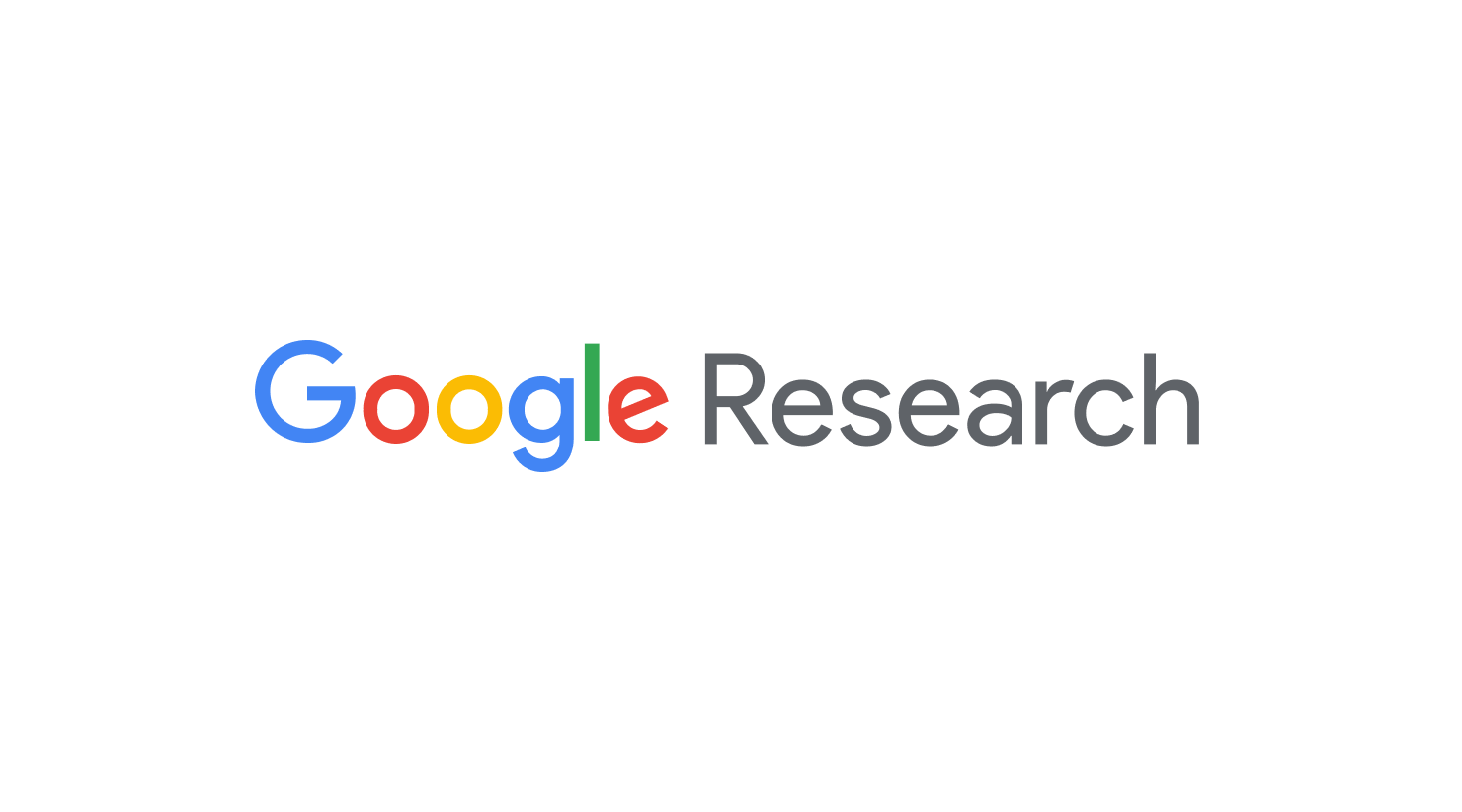
Advances in Variational Inference: Working Towards Large-scale Probabilistic Machine Learning at NIPS 2014
December 1, 2014
Posted by Shakir Mohamed and Charles Blundell, Google DeepMind, London
At Google, we continually explore and develop large-scale machine learning systems to improve our user’s experience, such as providing better video recommendations, deciding on the best language translation in a given context, or improving the accuracy of image search results. The data used to train these systems often contains many inconsistencies and missing elements, making progress towards large-scale probabilistic models designed to address these problems an important and ongoing part of our research. One principled and efficient approach for developing such models relies on an approach known as Variational Inference.
A renewed interest and several recent advances in variational inference1,2,3,4,5,6 has motivated us to support and co-organise this year’s workshop on Advances in Variational Inference as part of the Neural Information Processing Systems (NIPS) conference in Montreal. These advances include new methods for scalability using stochastic gradient methods, the ability to handle data that arrives continuously as a stream, inference in non-linear time-series models, principled regularisation in deep neural networks, and inference-based decision making in reinforcement learning, amongst others.
Whilst variational methods have clearly emerged as a leading approach for tractable, large-scale probabilistic inference, there remain important trade-offs in speed, accuracy, simplicity and applicability between variational and other approximative schemes. The goal of the workshop will be to contextualise these developments and address some of the many unanswered questions through:
- Contributed talks from 6 speakers who are leading the resurgence of variational inference, and shaping the debate on topics of stochastic optimisation, deep learning, Bayesian non-parametrics, and theory.
- 34 contributed papers covering significant advances in methodology, theory and applications including efficient optimisation, streaming data analysis, submodularity, non-parametric modelling and message passing.
- A panel discussion with leading researchers in the field that will further interrogate these ideas. Our panelists are David Blei, Neil Lawrence, Shinichi Nakajima and Matthias Seeger.
The workshop presents a fantastic opportunity to discuss the opportunities and obstacles facing the wider adoption of variational methods. The workshop will be held on the 13th December 2014 at the Montreal Convention and Exhibition Centre. For more details see: www.variationalinference.org.
References:
1. Rezende, Danilo J., Shakir Mohamed, and Daan Wierstra, Stochastic Backpropagation and Approximate Inference in Deep Generative Models, Proceedings of the 31st International Conference on Machine Learning (ICML-14), 2014.
2. Gregor, Karol, Ivo Danihelka, Andriy Mnih, Charles Blundell and Daan Wierstra, Deep AutoRegressive Networks, Proceedings of the 31st International Conference on Machine Learning (ICML-14), 2014.
3. Mnih, Andriy, and Karol Gregor, Neural Variational Inference and Learning in Belief Networks, Proceedings of the 31st International Conference on Machine Learning (ICML-14), 2014.
4. Kingma, D. P. and Welling, M., Auto-Encoding Variational Bayes, Proceedings of the International Conference on Learning Representations (ICLR), 2014.
5. Broderick, T., Boyd, N., Wibisono, A., Wilson, A. C., & Jordan, M., Streaming Variational Bayes, Advances in Neural Information Processing Systems (pp. 1727-1735), 2013.
6. Hoffman, M., Blei, D. M., Wang, C., and Paisley, J., Stochastic Variational Inference, Journal of Machine Learning Research, 14:1303–1347, 2013.