Publications
Our teams aspire to make discoveries that impact everyone, and core to our approach is sharing our research and tools to fuel progress in the field.
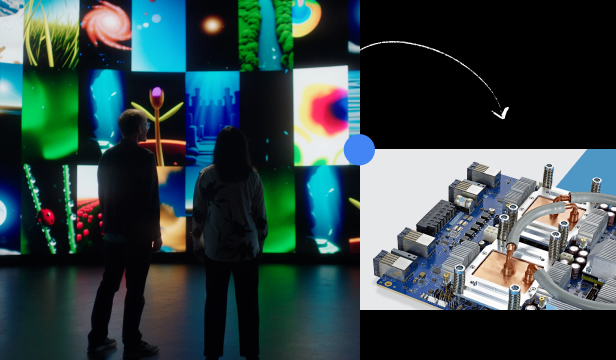
Publications
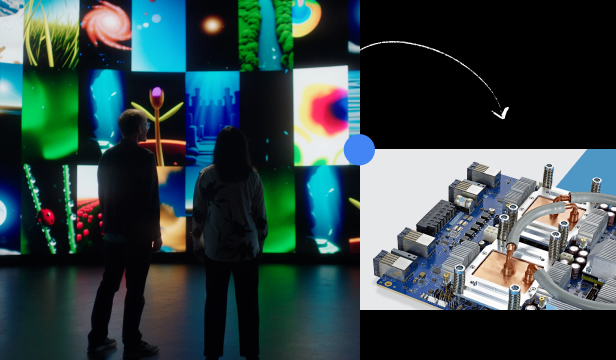
Sort By
1 - 15 of 340 publications
Media Mix Model Calibration With Bayesian Priors
Mike Wurm
Brenda Price
Ying Liu
research.google (2024)
Preview abstract
Effective model calibration is a critical and indispensable component in developing Media Mix Models (MMMs). One advantage of Bayesian-based MMMs lies in their capacity to accommodate the information from experiment results and the modelers' domain knowledge about the ad effectiveness by setting priors for the model parameters. However, it remains ambiguous about how and which Bayesian priors should be tuned for calibration purpose. In this paper, we propose a new calibration method through model reparameterization. The reparameterized model includes Return on Ads Spend (ROAS) as a model parameter, enabling straightforward adjustment of its prior distribution to align with either experiment results or the modeler's prior knowledge. The proposed method also helps address several key challenges regarding combining MMMs and incrementality experiments. We use simulations to demonstrate that our approach can significantly reduce the bias and uncertainty in the resultant posterior ROAS estimates.
View details
Modeling Recommender Ecosystems: Research Challenges at the Intersection of Mechanism Design, Reinforcement Learning and Generative Models
Martin Mladenov
Proceedings of the 38th Annual AAAI Conference on Artificial Intelligence (AAAI-24), Vancouver (2024) (to appear)
Preview abstract
Modern recommender systems lie at the heart of complex ecosystems that couple the behavior of users, content providers, advertisers, and other actors. Despite this, the focus of the majority of recommender research---and most practical recommenders of any import---is on the \emph{local, myopic} optimization of the recommendations made to individual users. This comes at a significant cost to the \emph{long-term utility} that recommenders could generate for its users. We argue that explicitly modeling the incentives and behaviors of all actors in the system---and the interactions among them induced by the recommender's policy---is strictly necessary if one is to maximize the value the system brings to these actors and improve overall ecosystem ``health.'' Doing so requires: optimization over long horizons using techniques such as \emph{reinforcement learning}; making inevitable tradeoffs among the utility that can be generated for different actors using the methods of \emph{social choice}; reducing information asymmetry, while accounting for incentives and strategic behavior, using the tools of \emph{mechanism design}; better modeling of both user and item-provider behaviors by incorporating notions from \emph{behavioral economics and psychology}; and exploiting recent advances in \emph{generative and foundation models} to make these mechanisms interpretable and actionable. We propose a conceptual framework that encompasses these elements, and articulate a number of research challenges that emerge at the intersection of these different disciplines.
View details
Data Exchange Markets via Utility Balancing
Aditya Bhaskara
Sungjin Im
Kamesh Munagala
Govind S. Sankar
WebConf (2024)
Preview abstract
This paper explores the design of a balanced data-sharing marketplace for entities with heterogeneous datasets and machine learning models that they seek to refine using data from other agents. The goal of the marketplace is to encourage participation for data sharing in the presence of such heterogeneity. Our market design approach for data sharing focuses on interim utility balance, where participants contribute and receive equitable utility from refinement of their models. We present such a market model for which we study computational complexity, solution existence, and approximation algorithms for welfare maximization and core stability. We finally support our theoretical insights with simulations on a mean estimation task inspired by road traffic delay estimation.
View details
Nash Equilibria of The Multiplayer Colonel Blotto Game on the Interval and Arbitrary Measure Spaces
Web And Internet Economics (WINE 2023) (2023) (to appear)
Preview abstract
The Colonel Blotto Problem proposed by Borel in 1921 has served as a widely applicable model of budget-constrained simultaneous winner-take-all competitions in the social sciences. Applications include elections, advertising, R&D and more. However, the classic Blotto problem and variants limit the study to competitions over a finite set of discrete battlefields. In this paper, we extend the classical theory to study multiplayer Blotto games over arbitrary measurable battlegrounds, provide an algorithm to efficiently sample equilibria of symmetric "equipartionable" Generalized Blotto games, and characterize the symmetric fair equilibria of the Blotto game over the unit interval.
View details
Preview abstract
We study the design of loss functions for click-through rates (CTR) to optimize (social) welfare in ad auctions. Existing works either only focus on CTR predictions without consideration of business objectives (e.g. welfare) in auctions or assume that the distribution over the participants' expected cost-per-impression (eCPM) is known a priori and uses various additional assumptions on the parametric form of the distribution to derive loss functions for predicting CTRs. In this work, we bring back the welfare objectives of ad auctions into CTR predictions and propose a novel weighted rankloss to train CTR model. Compared with the previous literature, our approach provides a provable guarantee on welfare but without any assumptions on the eCPMs' distribution, while also avoiding the intractability of naively applying existing learning-to-rank methods. We further propose a theoretically justifiable technique for calibrating the losses using labels generated from a teacher network, only assuming that the teacher network has bounded $\ell_2$ generalization error. Finally, we demonstrate the practical advantages of the proposed loss on both synthetic and real-world data.
View details
Preview abstract
Reach and frequency (R&F) is a core lever in the execution of ad campaigns, but it is not widely captured in the marketing mix models (MMMs) being fitted today due to the unavailability of accurate R&F metrics for some traditional media channels. Current practice usually uses impressions aggregated at regional level as inputs for MMMs, which does not take into account the fact that individuals can be exposed to an advertisement multiple times, and that the impact of an advertisement on an individual can change based on the number of times they are exposed. To address this limitation, we propose a R&F MMM which is an extension to Geo-level Bayesian Hierarchical Media Mix Modeling (GBHMMM) and is applicable when R&F data is available for at least one media channel. By incorporating R&F into MMM models, the new methodology is shown to produce more accurate estimates of the impact of marketing on business outcomes, and helps users optimize their campaign execution based on optimal frequency recommendations.
View details
Preview abstract
Advertisers in online ad auctions are increasingly using auto-bidding mechanisms to bid into auctions instead of directly bidding their value manually. One of the prominent auto-bidding formats is that of target cost-per-acquisition (tCPA) which maximizes the volume of conversions subject to a return-of-investment constraint. From an auction theoretic perspective however, this trend seems to go against foundational results that postulate that for profit-maximizing (aka quasi-linear) bidders, it is optimal to use a classic bidding system like marginal CPA (mCPA) bidding rather than using strategies like tCPA.
In this paper we rationalize the adoption of such seemingly sub-optimal bidding within the canonical quasi-linear framework. The crux of the argument lies in the notion of commitment. We consider a multi-stage game where first the auctioneer declares the auction rules; then bidders select either the tCPA or mCPA bidding format (and submit bids accordingly); and then, if the auctioneer lacks commitment, it can revisit the rules of the auction (e.g., may readjust reserve prices depending on the bids submitted by the bidders). Our main result is that so long as a bidder believes that the auctioneer lacks commitment to follow the rule of the declared auction then the bidder will make a higher profit by choosing the tCPA format compared to the classic mCPA format.
We then explore the commitment consequences for the auctioneer. In a simplified version of the model where there is only one bidder, we show that the tCPA subgame admits a credible equilibrium while the mCPA format does not. That is, when the bidder chooses the tCPA format the auctioneer can credibly implement the auction rules announced at the beginning of the game. We also show that, under some mild conditions, the auctioneer’s revenue is larger when the bidder uses the tCPA format rather than mCPA. We further quantify the value for the auctioneer to be able to commit to the declared auction rules.
View details
Preview abstract
Auto-bidding is now widely adopted as an interface between advertisers and internet advertising as it allows advertisers to specify high-level goals, such as maximizing value subject to a value-per-spend constraint. Prior research has mainly focused on auctions that are truthful (such as a second-price auction) because these auctions admit simple (uniform) bidding strategies and are thus simpler to analyze. The main contribution of this paper is to characterize the efficiency across the spectrum of all auctions, including non-truthful auctions for which optimal bidding may be complex.
For deterministic auctions, we show a dominance result: any uniform bidding equilibrium of a second-price auction (SPA) can be mapped to an equilibrium of any other auction – for example, first price auction (FPA) – with identical outcomes. In this sense, SPA with uniform bidding is an instance-wise optimal deterministic auction. Consequently, the price of anarchy (PoA) of any deterministic auction is at least the PoA of SPA with uniform bidding, which is known to be 2. We complement this by showing that the PoA of FPA without uniform bidding is 2.
Next, we show, surprisingly, that truthful pricing is not dominant in the randomized setting. There is a randomized version of FPA that achieves a strictly smaller price of anarchy than its truthful counterpart when there are two bidders per query. Furthermore, this randomized FPA achieves the best-known PoA for two bidders, thus showing the power of non-truthfulness when combined with randomization. Finally, we show that no prior-free auction (even randomized, non-truthful) can improve on a PoA bound of 2 when there are a large number of advertisers per auction. These results should be interpreted qualitatively as follows. When the auction pressure is low, randomization and non-truthfulness is beneficial. On the other hand, if the auction pressure is intense, the benefits diminishes and it is optimal to implement a second-price auction.
View details
Learning to Bid in Contextual First Price Auctions
Guru Prashanth Guruganesh
The Proceedings of the ACM Web Conference 2023
Preview abstract
In this paper, we investigate the problem about how to bid in repeated contextual first price
auctions. We consider a single bidder (learner) who repeatedly bids in the first price auctions:
at each time t, the learner observes a context xt ∈ R
d and decides the bid based on historical
information and xt. We assume a structured linear model of the maximum bid of all the others
mt = α0 · xt + zt, where α0 ∈ R
d
is unknown to the learner and zt is randomly sampled from a
noise distribution F with log-concave density function f. We consider both binary feedback (the
learner can only observe whether she wins or not) and full information feedback (the learner can
observe mt) at the end of each time t. For binary feedback, when the noise distribution F is
known, we propose a bidding algorithm, by using maximum likelihood estimation (MLE) method
to achieve at most Oe(
p
log(d)T ) regret. Moreover, we generalize this algorithm to the setting
with binary feedback and the noise distribution is unknown but belongs to a parametrized family
of distributions. For the full information feedback with unknown noise distribution, we provide
an algorithm that achieves regret at most Oe(
√
dT). Our approach combines an estimator for
log-concave density functions and then MLE method to learn the noise distribution F and linear
weight α0 simultaneously. We also provide a lower bound result such that any bidding policy
in a broad class must achieve regret at least Ω(√
T), even when the learner receives the full
information feedback and F is known.
View details
Preview abstract
We propose two novel approaches to address a critical problem of reach measurement across multiple media -- how to estimate the reach of an unobserved subset of buying groups (BGs) based on the observed reach of other subsets of BGs. Specifically, we propose a model-free approach and a model-based approach. The former provides a coarse estimate for the reach of any subset by leveraging the consistency among the reach of different subsets. Linear programming is used to capture the constraints of the reach consistency. This produces an upper and a lower bound for the reach of any subset. The latter provides a point estimate for the reach of any subset. The key idea behind the latter is to exploit the conditional independence model. In particular, the groups of the model are created by assuming each BG has either high or low reach probability in a group, and the weights of each group are determined through solving a non-negative least squares (NNLS) problem. In addition, we also provide a framework to give both confidence interval and point estimates by integrating these two approaches with training points selection and parameter fine-tuning through cross-validation. Finally, we evaluate the two approaches through experiments on synthetic data.
View details
Common survey issues in UX research Hands on workshop
QuantUXCon 2023
Preview abstract
After having reviewed hundreds of surveys in my career I would like to share what I learned from it. In this hands-on workshop I will discuss how you can improve the survey you are planning to field for your UX project.
We will start with a decision tree to decide if a survey is actually the best method for your research process. Then we will move to some feasibility issues such as sample size and response rates.
Before starting the second part of the workshop I will present the top 10 issues I found in my career reviewing surveys.
In the second part of the workshop I will answer survey questions the audience has using the chat feature in Hopin (or join on screen to pose live questions).
View details
Autobidding Auctions in the Presence of User Costs
Hanrui Zhang
Proceedings of the ACM Web Conference 2023, pp. 3428-3435
Preview abstract
Although costs are prevalent in ad auctions, not many auction theory works study auction design in the presence of cost in the classic settings. One reason is that most auctions design in the setting without cost directly generalize to the setting with cost when the bidders maximizing quasi-linear utility.
However, as auto-bidding becomes a major choice of advertisers in online advertising, the distinction from the underlying behavior model often leads to different solutions of many well-studied auctions. In the context of ad auctions with cost, VCG achieves optimal welfare with quasi-linear utility maximizing bidders, while has 0 welfare approximation guarantee with value maximizing bidders who follow the optimization behind common auto-bidding algorithms.
In this paper, we prove that the approximation welfare guarantee of VCG auction can be significantly improved by a minimal change --- introducing cost multipliers. We note that one can use either one multiplier per auction or one multiplier per bidder, but one global multiplier across all auctions and bidders does not work. Finally, to echo with our theoretical results, we conduct empirical evaluations using semi-synthetic data derived from real auction data of a major advertising platform.
View details
Incentive Compatibility in the Auto-bidding World
Yeganeh Alimohammadi
Andres Perlroth
24th ACM Conference on Economics and Computation, EC 2023
Preview abstract
Auto-bidding has recently become a popular feature in ad auctions. This feature enables advertisers to simply provide high-level constraints and goals to an automated agent, which optimizes their auction bids on their behalf. These auto-bidding intermediaries interact in a decentralized manner in the underlying auctions, leading to new interesting practical and theoretical questions on auction design, for example, in understanding the bidding equilibrium properties between auto-bidder intermediaries for different auctions. In this paper, we examine the effect of different auctions on the incentives of advertisers to report their constraints to the auto-bidder intermediaries. More precisely, we study whether canonical auctions such as first price auction (FPA) and second price auction (SPA) are auto-bidding incentive compatible (AIC): whether an advertiser can gain by misreporting their constraints to the autobidder.
View details
Recent Books and Journals Articles in Public Opinion, Survey Methods, Survey Statistics, Big Data, Data Science, and User Experience Research. 2022 Update
Survey Practice, vol. 16 (2023)
Preview abstract
Welcome to the 15th edition of this column on recent books and journal articles in the field of public opinion, survey methods, survey statistics, Big Data, data science and user experience research.
Special issues of journals have a space in this article because, in our view, they are like edited books. We also added review papers from the journal series of Annual Reviews because these papers are seminal state of the art write ups, a mini book, if you wish on a specific subject.
This article is an update of the books and journals published in the 2021 article. Like the previous year, the books are organized by topic; this should help the readers to focus on their interests.
You will note that we use very broad definitions of public opinion, survey methods, survey statistics, Big Data, data science, and user experience research. This is because there are many books published in different outlets that can be very useful to the readers of Survey Practice, even if they do not come from traditional sources of survey content.
It is unlikely we have exhaustively listed all new books in each subcategory; we did our best scouting different resources and websites, but we take full responsibility for any omissions. The list is also focused only on books published in the English language and available for purchase (as an ebook or in print) at the time of this review (October 2023) and with the printed copyright year of 2022. Books are listed based on the relevance to the topic, and no judgment is made in terms of quality of the content. We let the readers do so.
If you want to send information for the next issue, please send it to surveypractice.new.books@gmail.com.
View details
Preview abstract
Welcome to the 14th edition of this column on recent books and journal articles in the field of public opinion, survey methods, survey statistics, Big Data, data science, and user experience research.
Special issues of journals have a space in this article because, in our view, they are like edited books. We also added review papers from the journal series of Annual Reviews because these papers are seminal state of the art write ups, a short book, if you wish on a specific subject.
This article is an update of the books and journals published in the 2020 article. Like the previous year, the books are organized by topic; this should help the readers to focus on their interests.
You will note that we use very broad definitions of public opinion, survey methods, survey statistics, Big Data, data science, and user experience research. This is because there are many books published in different outlets that can be very useful to the readers of Survey Practice, even if they do not come from traditional sources of survey content.
It is unlikely we have exhaustively listed all new books in each subcategory; we did our best scouting different resources and websites, but we take full responsibility for any omissions. The list is also focused only on books published in the English language and available for purchase (as an ebook or in print) at the time of this review (October 2023) and with the printed copyright year of 2021. Books are listed based on the relevance to the topic, and no judgment is made in terms of quality of the content. We let the readers do so.
If you want to send information for the next issue, please send it to surveypractice.new.books@gmail.com.
View details