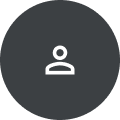
Zoya Svitkina
Research Areas
Authored Publications
Sort By
Google
Optimal Coordination Mechanisms for Unrelated Machine Scheduling
Preview
Yossi Azar
Lisa Fleischer
Kamal Jain
Operations Research, 63 (2015), pp. 489-500
On Distributing Symmetric Streaming Computations
Preview
S. Muthukrishnan
Anastasios Sidiropoulos
Cliff Stein
Proc. 19th Annual Symposium on Discrete Algorithms (SODA) (2008)
Stochastic Models for Budget Optimization in Search-Based Advertising
Preview
S. Muthukrishnan
Internet and Network Economics (WINE), Springer, San Diego (2007), pp. 131-142