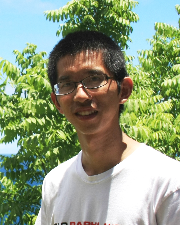
Nan Ding
Research Areas
Authored Publications
Sort By
Google
PaLI: A Jointly-Scaled Multilingual Language-Image Model
Piotr Padlewski
Daniel Salz
Sebastian Alexander Goodman
Basil Mustafa
Lucas Beyer
Alexander Kolesnikov
Keran Rong
Hassan Akbari
Linting Xue
James Bradbury
Chao Jia
Carlos Riquelme
Xiaohua Zhai
Neil Houlsby
International Conference on Learning Representations (ICLR) (2023)
Bridging the Gap Between Practice and PAC-Bayes Theory in Few-shot Meta-learning
Sebastian Alexander Goodman
Advances in Neural Information Processing Systems 2021
Characterizing Quantum Supremacy in Near-Term Devices
Sergei Isakov
Vadim Smelyanskiy
Michael J. Bremner
John Martinis
Nature Physics, 14 (2018), 595–600