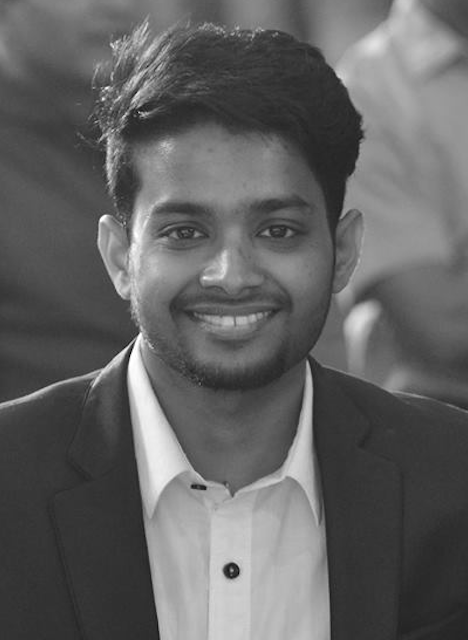
Mohit Agarwal
Mohit Agarwal is a Research Engineer at Google, where he focuses on advancing geospatial inference and large language models (LLMs). He joined Google in 2023, bringing with him a strong foundation in data-driven research and machine learning applications. Prior to Google, Mohit worked as a Quantitative Strategist at Goldman Sachs, where he specialized in leveraging data science and machine learning techniques to drive equity research insights. Mohit completed his Ph.D. in Electrical and Computer Engineering at the Georgia Institute of Technology, where he worked under the guidance of Dr. Raghupathy Sivakumar. His doctoral research explored the intersection of Brain-Computer Interfaces (BCIs) and Human-Assisted Machine Learning, focusing on novel approaches to human-centered AI.
Mohit holds a Bachelor's degree from the Indian Institute of Technology Kanpur, which he completed in 2014.
Authored Publications
Sort By
Google
General Geospatial Inference with a Population Dynamics Foundation Model
Chaitanya Kamath
Prithul Sarker
Joydeep Paul
Yael Mayer
Sheila de Guia
Jamie McPike
Adam Boulanger
David Schottlander
Yao Xiao
Manjit Chakravarthy Manukonda
Monica Bharel
Von Nguyen
Luke Barrington
Niv Efron
Krish Eswaran
Shravya Shetty
(2024) (to appear)