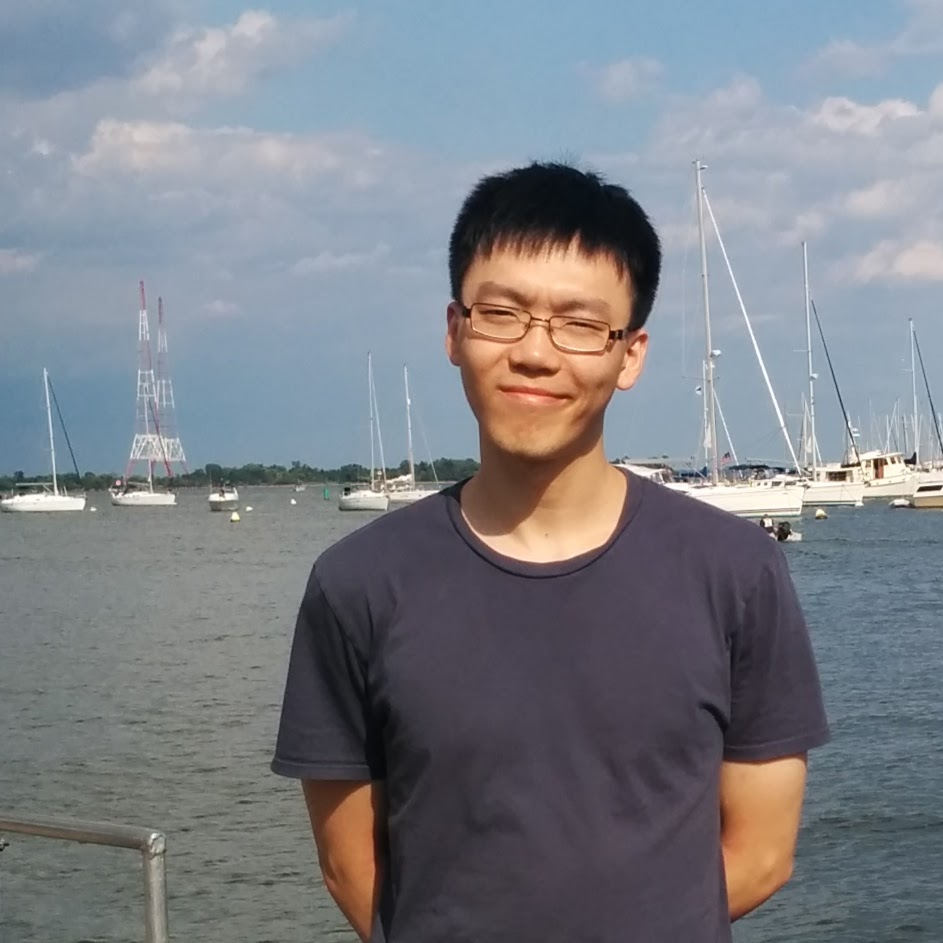
Di Wang
Di Wang is in the Market Algorithm team, which is part of the broader Algorithms, Theory, Neural networks, and AI (Athena) team under Google Research. Di finished his PhD in Computer Science at UC Berkeley where he was advised by Satish Rao. Before joining Google, Di worked as a postdoc researcher at the Simons Institute (UC Berkeley) and the Algorithms & Randomness Center at Georgia Tech. Di's research interests are in the design of efficient algorithms, especially for large-scale optimization problems and graph problems that arise broadly in applications from machine learning, data analysis and operations research. Di's work draws on a broad range of numerical and discrete tools from combinatorics, optimization and graph theory, which leads to not only stronger theoretical guarantees, but also practical improvements for computational challenges arising in practice.
Authored Publications
Sort By
Google
Online Bidding under RoS Constraints without Knowing the Value
Sushant Vijayan
Swati Padmanabhan
The Web Conference (2025)
Auto-bidding and Auctions in Online Advertising: A Survey
Ashwinkumar Badanidiyuru Varadaraja
Christopher Liaw
Haihao (Sean) Lu
Andres Perlroth
Georgios Piliouras
Ariel Schvartzman
Kelly Spendlove
Hanrui Zhang
Mingfei Zhao
ACM SIGecom Exchanges, 22 (2024)
A Field Guide for Pacing Budget and ROS Constraints
Haihao (Sean) Lu
ICML (2024)
Minimum Cost Flows, MDPs, and $\ell_1$-Regression in Nearly Linear Time for Dense Instances
Jan van den Brand
Yin Tat Lee
Yang P. Liu
Thatchaphol Saranurak
Aaron Sidford
Zhao Song
The 53rd ACM Symposium on Theory of Computing (STOC) (2021) (to appear)
Packing LPs are Hard to Solve Accurately, Assuming Linear Equations are Hard
Preview
Rasmus Kyng
Peng Zhang
Proceedings of the 2020 ACM-SIAM Symposium on Discrete Algorithms (SODA) (2020)