Publications
Our teams aspire to make discoveries that impact everyone, and core to our approach is sharing our research and tools to fuel progress in the field.
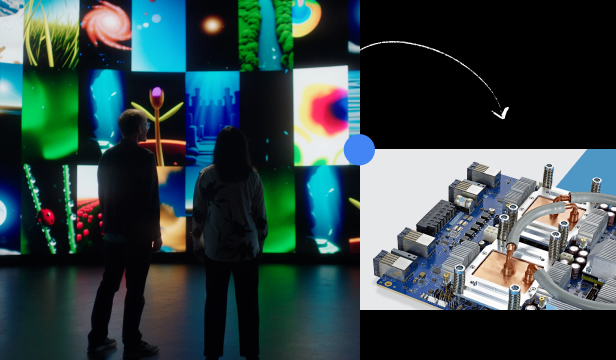
Publications
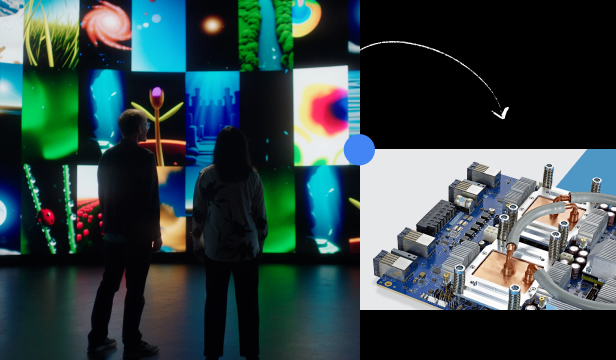
Sort By
1 - 15 of 450 publications
Dynamic Inference of Likely Symbolic Tensor Shapes in Python Machine Learning Programs
Koushik Sen
International Conference on Software Engineering: Software Engineering in Practice (ICSE-SEIP) (2024) (to appear)
Preview abstract
In machine learning programs, it is often tedious to annotate the dimensions of shapes of various tensors that get created during execution. We present a dynamic likely tensor shape inference analysis that annotates the dimensions of shapes of tensor expressions with symbolic dimension values. Such annotations can be used for understanding the machine learning code written in popular frameworks, such as TensorFlow, PyTorch, JAX, and for finding bugs related to tensor shape mismatch.
View details
Preview abstract
2022 marked the 50th anniversary of memory safety vulnerabilities, first reported by Anderson et al. Half a century later, we are still dealing with memory safety bugs despite substantial investments to improve memory unsafe languages.
Like others', Google’s data and internal vulnerability research show that memory safety bugs are widespread and one of the leading causes of vulnerabilities in memory-unsafe codebases. Those vulnerabilities endanger end users, our industry, and the broader society.
At Google, we have decades of experience addressing, at scale, large classes of vulnerabilities that were once similarly prevalent as memory safety issues. Based on this experience we expect that high assurance memory safety can only be achieved via a Secure-by-Design approach centered around comprehensive adoption of languages with rigorous memory safety guarantees.
We see no realistic path for an evolution of C++ into a language with rigorous memory safety guarantees that include temporal safety. As a consequence, we are considering a gradual transition of C++ code at Google towards other languages that are memory safe.
Given the large volume of pre-existing C++, we believe it is nonetheless necessary to improve the safety of C++ to the extent practicable. We are considering transitioning to a safer C++ subset, augmented with hardware security features like MTE.
View details
CodeQueries: A Dataset of Semantic Queries over Code
Surya Prakash Sahu
Madhurima Mandal
Shikhar Bharadwaj
Aditya Kanade
Shirish Shevade
Innovations in Software Engineering (ISEC), ACM, Bangalore, India (2024)
Preview abstract
Developers often have questions about semantic aspects of code
they are working on, e.g., “Is there a class whose parent classes
declare a conflicting attribute?”. Answering them requires understanding code semantics such as attributes and inheritance relation
of classes. An answer to such a question should identify code spans
constituting the answer (e.g., the declaration of the subclass) as well
as supporting facts (e.g., the definitions of the conflicting attributes).
The existing work on question-answering over code has considered
yes/no questions or method-level context. We contribute a labeled
dataset, called CodeQueries, of semantic queries over Python code.
Compared to the existing datasets, in CodeQueries, the queries
are about code semantics, the context is file level and the answers
are code spans. We curate the dataset based on queries supported
by a widely-used static analysis tool, CodeQL, and include both
positive and negative examples, and queries requiring single-hop
and multi-hop reasoning.
To assess the value of our dataset, we evaluate baseline neural
approaches. We study a large language model (GPT3.5-Turbo) in
zero-shot and few-shot settings on a subset of CodeQueries. We
also evaluate a BERT style model (CuBERT) with fine-tuning. We
find that these models achieve limited success on CodeQueries.
CodeQueries is thus a challenging dataset to test the ability of
neural models, to understand code semantics, in the extractive
question-answering setting
View details
Preview abstract
Automatic differentiation (AD) is conventionally understood as a family of distinct algorithms, rooted in two "modes" -- forward and reverse -- which are typically presented (and implemented) separately. Can there be only one? Following up on the AD systems developed in the JAX and Dex projects, we formalize a decomposition of reverse-mode AD into (i) forward-mode AD followed by (ii) unzipping the linear and non-linear parts and then (iii) transposition of the linear part.
To that end, we define a (substructurally) linear type system that can prove a class of functions are (algebraically) linear. Our main results are that forward-mode AD produces such linear functions, and that we can unzip and transpose any such linear function, conserving cost, size, and linearity. Composing these three transformations recovers reverse-mode AD. This decomposition also sheds light on checkpointing, which emerges naturally from a free choice in unzipping `let` expressions. As a corollary, checkpointing techniques are applicable to general-purpose partial evaluation, not just AD.
We hope that our formalization will lead to a deeper understanding of automatic differentiation and that it will simplify implementations, by separating the concerns of differentiation proper from the concerns of gaining efficiency (namely, separating the derivative computation from the act of running it backward).
View details
Preview abstract
Identifying invariants in programs is an important program analysis task with applications towards program understanding, vulnerability analysis, and formal verification. Existing tools for identifying invariants rely on dynamic analysis, requiring traces collected from multiple executions in order to produce reliable invariants. We study the application of large language models to invariant prediction, finding that models training on source code and fine-tuned to invariant prediction can perform invariant prediction as static rather than dynamic analysis. Using a scratchpad approach gives the best performance, finding invariants statically of quality comparable to those obtained by a dynamic analysis tool with access to five program traces.
View details
Grisette: Symbolic Compilation as a Functional Programming Library
Sirui Lu
Grisette: Symbolic Compilation as a Functional Programming Library, ACM (2023) (to appear)
Preview abstract
The development of constraint solvers simplified automated reasoning about programs and shifted the engineering burden to implementing symbolic compilation tools that translate programs into efficiently solvable constraints. We describe Grisette, a reusable symbolic evaluation framework for implementing domain-specific symbolic compilers. Grisette evaluates all execution paths and merges their states into a normal form that avoids making guards mutually exclusive. This ordered-guards representation reduces the constraint size 5-fold and the solving time more than 2-fold. Grisette is designed entirely as a library, which sidesteps the complications of lifting the host language into the symbolic domain. Grisette is purely functional, enabling memoization of symbolic compilation as well as monadic integration with host libraries. Grisette is statically typed, which allows catching programming errors at compile time rather than delaying their detection to the constraint solver. We implemented Grisette in Haskell and evaluated it on benchmarks that stress both the symbolic evaluation and constraint solving.
View details
Predicting Dynamic Properties of Heap Allocations Using Neural Networks Trained on Static Code
Christian Navasca
Guoqing Harry Xu
2023 ACM SIGPLAN International Symposium on Memory Management (ISMM 2023)
Preview abstract
Memory allocators and runtime systems can leverage dynamic properties of heap allocations – such as object lifetimes, hotness or access correlations – to improve performance and resource consumption. A significant amount of work has focused on approaches that collect this information in performance profiles and then use it in new memory allocator or runtime designs, both offline (in ahead-of-time compilers) and online (in JIT compilers). This is a special instance of profile-guided optimization.
This approach has significant disadvantages: 1) The profiling oftentimes introduces substantial overheads, which are prohibitive in many production scenarios, 2) Creating a representative profiling run adds significant engineering complexity and reduces deployment velocity, and 3) Profiles gathered ahead of time or during the warm-up phase of a server are often not representative of all workload behavior and may miss important corner cases.
In this paper, we investigate a fundamentally different approach. Instead of deriving heap allocation properties from profiles, we explore the ability of neural network models to predict them from the statically available code. As an intellectual abstract, we do not offer a conclusive answer but describe the trade-off space of this approach, investigate promising directions, motivate these directions with data analysis and experiments, and highlight challenges that future work needs to overcome.
View details
Snowcat: Efficient Kernel Concurrency Testing using a Learned Coverage Predictor
Sishuai Gong
Dinglan Peng
Pedro Fonseca
Symposium on Operating Systems Principles (SOSP) (2023)
Preview abstract
Random-based approaches and heuristics are commonly
used in kernel concurrency testing due to the massive scale
of modern kernels and corresponding interleaving space.
The lack of accurate and scalable approaches to analyze concurrent
kernel executions makes existing testing approaches
heavily rely on expensive dynamic executions to measure
the effectiveness of a new test. Unfortunately, the high cost
incurred by dynamic executions limits the breadth of the
exploration and puts latency pressure on finding effective
concurrent test inputs and schedules, hindering the overall
testing effectiveness.
This paper proposes Snowcat, a kernel concurrency testing
framework that generates effective test inputs and schedules
using a learned kernel block-coverage predictor. Using a
graph neural network, the coverage predictor takes a concurrent
test input and scheduling hints and outputs a prediction
on whether certain important code blocks will be executed.
Using this predictor, Snowcat can skip concurrent tests that
are likely to be fruitless and prioritize the promising ones
for actual dynamic execution.
After testing the Linux kernel for over a week, Snowcat
finds ∼17% more potential data races, by prioritizing tests of
more fruitful schedules than existing work would have chosen.
Snowcat can also find effective test inputs that expose
new concurrency bugs with higher probability (1.4×∼2.6×),
or reproduce known bugs more quickly (15×) than state-ofart
testing tools. More importantly, Snowcat is shown to be
more efficient at reaching a desirable level of race coverage
in the continuous setting, as the Linux kernel evolves from
version to version. In total, Snowcat discovered 17 new concurrency
bugs in Linux kernel 6.1, of which 13 are confirmed
and 6 are fixed.
View details
Propeller: A Profile Guided, Relinking Optimizer for Warehouse-Scale Applications
Han Shen
Rahman Lavaee
ACM, pp. 617-631
Preview abstract
While profile guided optimizations (PGO) and link time optimiza-tions (LTO) have been widely adopted, post link optimizations (PLO)have languished until recently when researchers demonstrated that late injection of profiles can yield significant performance improvements. However, the disassembly-driven, monolithic design of post link optimizers face scaling challenges with large binaries andis at odds with distributed build systems. To reconcile and enable post link optimizations within a distributed build environment, we propose Propeller, a relinking optimizer for warehouse scale work-loads. To enable flexible code layout optimizations, we introduce basic block sections, a novel linker abstraction. Propeller uses basic block sections to enable a new approach to PLO without disassembly. Propeller achieves scalability by relinking the binary using precise profiles instead of rewriting the binary. The overhead of relinking is lowered by caching and leveraging distributed compiler actions during code generation. Propeller has been deployed to production at Google with over tens of millions of cores executing Propeller optimized code at any time. An evaluation of internal warehouse-scale applications show Propeller improves performance by 1.1% to 8% beyond PGO and ThinLTO. Compiler tools such as Clang improve by 7% while MySQL improves by 1%. Compared to the state of the art binary optimizer, Propeller achieves comparable performance while lowering memory overheads by 30%-70% on large benchmarks.
View details
Code Generation for Data-Dependent Stencils
Mohammed Essadki
Bertrand Michel
Bruno Maugars
Nicolas Vasilache
CGO, IEEE (2023)
Preview abstract
Numerical simulation often resorts to iterative in-place stencils such as the Gauss-Seidel or Successive Overrelaxation (SOR) methods. Writing high performance implementations of such stencils requires significant effort and time; it also involves non-local transformations beyond the stencil kernel itself. While automated code generation is a mature technology for image processing stencils, convolutions and out-of place iterative stencils (such as the Jacobi method), the optimization of in-place stencils requires manual craftsmanship. Building on recent advances in tensor compiler construction, we propose the first domain-specific code generator for iterative in-place stencils. Starting from a generic tensor compiler implemented in the MLIR framework, tensor abstractions are incrementally refined and lowered down to parallel, tiled, fused and vectorized code. We used our generator to implement a realistic, implicit solver for structured meshes, and demonstrate results competitive with an industrial computational fluid dynamics framework. We also compare with stand-alone stencil kernels for dense tensors.
View details
PTStore: Lightweight Architectural Support for Page Table Isolation
Wende Tan
Yangyu Chen
Yuan Li
Ying Liu
Jianping Wu
Chao Zhang
2023 60th ACM/IEEE Design Automation Conference (DAC), IEEE, pp. 1-6
Preview abstract
Page tables are critical data structures in kernels, serving as the trust base of most mitigation solutions. Their integrity is thus crucial but is often taken for granted. Existing page table protection solutions usually provide insufficient security guarantees, require heavy hardware, or introduce high overheads. In this paper, we present a novel lightweight hardware-software co-design solution, PTStore, consisting of a secure region storing page tables and tokens verifying page table pointers. Evaluation results on FPGA-based prototypes show that PTStore only introduces <0.92% hardware overheads and <0.86% performance overheads, but provides strong security guarantees, showing that PTStore is efficient and effective.
View details
Practical Design Considerations for Wide Locally Recoverable Codes (LRCs)
Shashwat Silas
Dave Clausen
File and Storage Technologies (FAST), USENIX (2023)
Preview abstract
Most of the data in large-scale storage clusters is erasure coded. At exascale, optimizing erasure codes for low storage overhead, efficient reconstruction, and easy deployment is of critical importance. Locally recoverable codes (LRCs) have deservedly gained central importance in this field, because they can balance many of these requirements. In our work we study wide LRCs; LRCs with large number of blocks per stripe and low storage overhead. These codes are a natural next step for practitioners to unlock higher storage savings, but they come with their own challenges. Of particular interest is their reliability, since wider stripes are prone to more simultaneous failures.
We conduct a practically-minded analysis of several popular and novel LRCs. We find that wide LRC reliability is a subtle phenomenon that is sensitive to several design choices, some of which are overlooked by theoreticians, and others by practitioners. Based on these insights, we construct novel LRCs called Uniform Cauchy LRCs, which show excellent performance in simulations, and a 33% improvement in reliability on unavailability events observed by a wide LRC deployed in a Google storage cluster. We also show that these codes are easy to deploy in a manner that improves their robustness to common maintenance events. Along the way, we also give a remarkably simple and novel construction of distance optimal LRCs (other constructions are also known), which may be of interest to theory-minded readers.
View details
RL4ReAl: Reinforcement Learning for Register Allocation
S. VenkataKeerthy
Siddharth Jain
Anilava Kundu
Rohit Aggarwal
Ramakrishna Upadrasta
CC 2023, ACM
Preview abstract
We aim to automate decades of research and experience in register allocation, leveraging machine learning. We tackle this problem by embedding a multi-agent reinforcement learning algorithm within LLVM, training it with the state of the art techniques. We formalize the constraints that precisely define the problem for a given instruction-set architecture, while ensuring that the generated code preserves semantic correctness. We also develop a gRPC-based framework providing a modular and efficient compiler interface for training and inference. Our approach is architecture independent: we show experimental results targeting Intel x86 and ARM AArch64. Our results match or out-perform the
heavily tuned, production-grade register allocators of LLVM.
View details
Profiling Hyperscale Big Data Processing
Aasheesh Kolli
Abraham Gonzalez
Samira Khan
Sihang Liu
Krste Asanovic
ISCA (2023)
Preview abstract
Computing demand continues to grow exponentially, largely driven by "big data" processing on hyperscale data stores. At the same time, the slowdown in Moore's law is leading the industry to embrace custom computing in large-scale systems. Taken together, these trends motivate the need to characterize live production traffic on these large data processing platforms and understand the opportunity of acceleration at scale.
This paper addresses this key need. We characterize three important production distributed database and data analytics platforms at Google to identify key hardware acceleration opportunities and perform a comprehensive limits study to understand the trade-offs among various hardware acceleration strategies.
We observe that hyperscale data processing platforms spend significant time on distributed storage and other remote work across distributed workers. Therefore, optimizing storage and remote work in addition to compute acceleration is critical for these platforms. We present a detailed breakdown of the compute-intensive functions in these platforms and identify dominant key data operations related to datacenter and systems taxes. We observe that no single accelerator can provide a significant benefit but collectively, a sea of accelerators, can accelerate many of these smaller platform-specific functions. We demonstrate the potential gains of the sea of accelerators proposal in a limits study and analytical model. We perform a comprehensive study to understand the trade-offs between accelerator location (on-chip/off-chip) and invocation model (synchronous/asynchronous). We propose and evaluate a chained accelerator execution model where identified compute-intensive functions are accelerated and pipelined to avoid invocation from the core, achieving a 3x improvement over the baseline system while nearly matching identical performance to an ideal fully asynchronous execution model.
View details
Preview abstract
MLIR has recently introduced support for declaratively specifying and controlling compiler transformations via the transform dialect. It allows one to request compiler transformations using compiler IR itself, which can be embedded into the original IR that is being transformed (similarly to pragmas) or supplied separately (similarly to scheduling languages). This tutorial presents the concepts of the MLIR transform dialect and related infrastructure. It will be accompanied by a practical demonstration of three use scenarios.
- Composing transform dialect operations available in (upstream) MLIR to perform a sequence of optimizing transformations that results in efficient code for an MLIR linear algebra operation.
- Defining new transform dialect operations and adapting existing transformation code to work with the transform dialect infrastructure.
- Setting up and using the transform dialect infrastructure in a downstream out-of-tree project with custom dialects, transformations and passes.
After following the tutorial, the attendees will be able to apply the transform dialect in their work and extend it when necessary. Basic familiarity with MLIR is a prerequisite.
View details