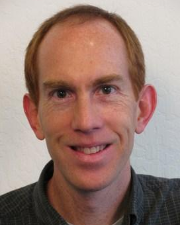
Rich Washington
Rich Washington is a Software Engineer at Google in Paris, France. His current work is on Operations Research. Previously within Google he worked on YouTube video annotation, web search quality, spelling correction, and biologically-inspired vision algorithms.
From 1997-2004 he worked at NASA Ames Research Center on Mars rover autonomy and plan execution. He holds a BA in Mathematics from Johns Hopkins University and a PhD in Computer Science (Artificial Intelligence) from Stanford University.
Research Areas
Authored Publications
Sort By
Google
Recursive Sparse Spatiotemporal Coding
Thomas Dean
Proceedings of the Fifth IEEE International Workshop on Multimedia Information Processing and Retrieval, IEEE Computer Society (2009)
On the Prospects for Building a Working Model of the Visual Cortex
Thomas Dean
Glenn Carroll
Proceedings of AAAI-07, MIT Press, Cambridge, Massachusetts (2007), pp. 1597-1600