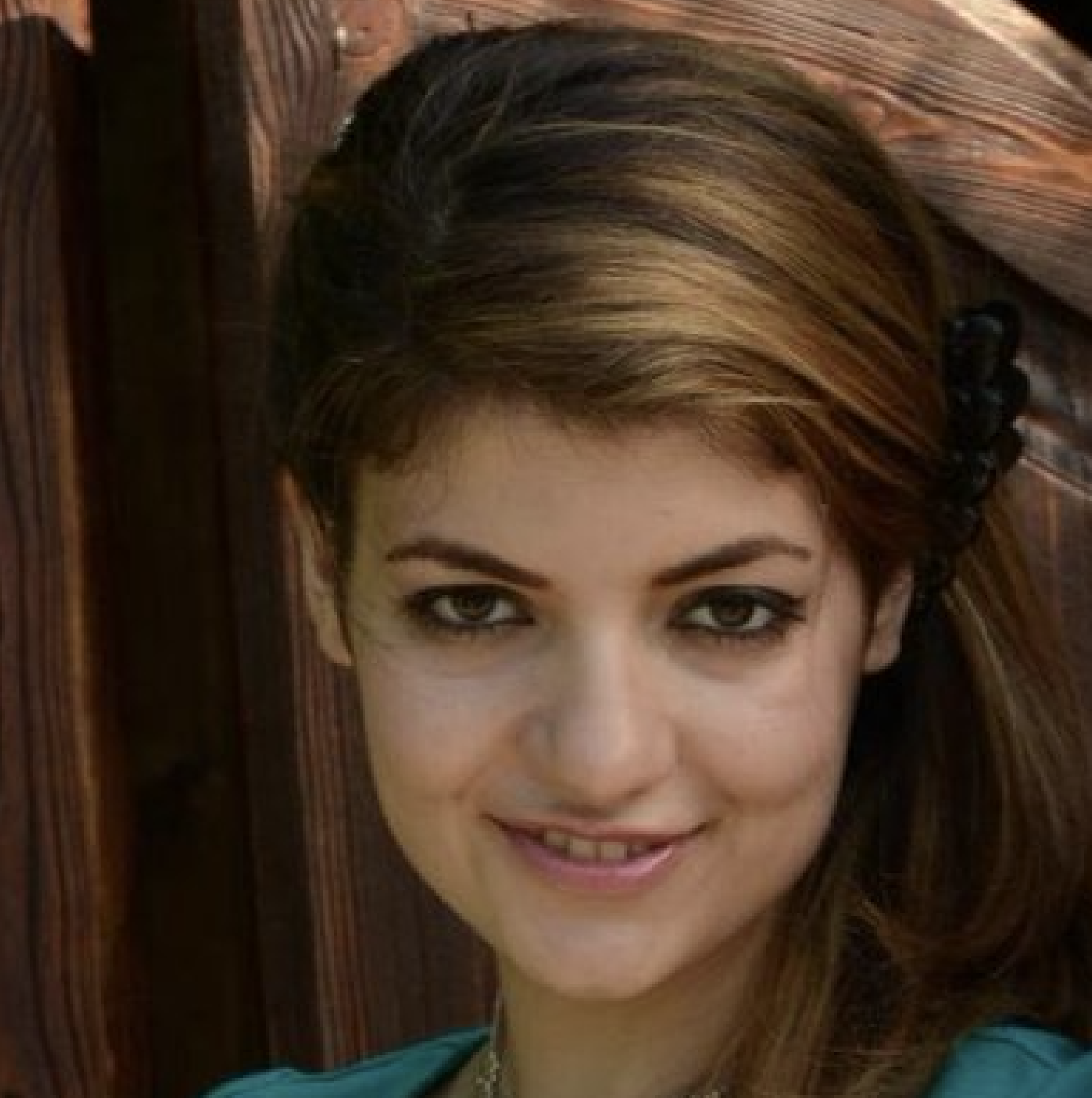
Negar Rostamzadeh
Negar Rostamzadeh is a Staff Research Scientist at Google Responsible AI team.
Research Areas
Authored Publications
Sort By
Preview abstract
Generative AI (GAI) is proliferating, and among its many applications are to support creative work (e.g., generating text, images, music) and to enhance
accessibility (e.g., captions of images and audio). As GAI evolves, creatives must consider how (or how not) to incorporate these tools into their practices.
In this paper, we present interviews at the intersection of these applications. We learned from 10 creatives with disabilities who intentionally use and
do not use GAI in and around their creative work. Their mediums ranged from audio engineering to leatherwork, and they collectively experienced a variety
of disabilities, from sensory to motor to invisible disabilities. We share cross-cutting themes of their access hacks, how creative practice and access
work become entangled, and their perspectives on how GAI should and should not ft into their workfows. In turn, we ofer qualities of accessible creativity
with responsible AI that can inform future research.
View details
A Toolbox for Surfacing Health Equity Harms and Biases in Large Language Models
Heather Cole-Lewis
Nenad Tomašev
Liam McCoy
Leo Anthony Celi
Alanna Walton
Akeiylah DeWitt
Philip Mansfield
Sushant Prakash
Joelle Barral
Ivor Horn
Karan Singhal
Arxiv(2024) (to appear)
Preview abstract
Large language models (LLMs) hold immense promise to serve complex health information needs but also have the potential to introduce harm and exacerbate health disparities. Reliably evaluating equity-related model failures is a critical step toward developing systems that promote health equity. In this work, we present resources and methodologies for surfacing biases with potential to precipitate equity-related harms in long-form, LLM-generated answers to medical questions and then conduct an empirical case study with Med-PaLM 2, resulting in the largest human evaluation study in this area to date. Our contributions include a multifactorial framework for human assessment of LLM-generated answers for biases, and EquityMedQA, a collection of seven newly-released datasets comprising both manually-curated and LLM-generated questions enriched for adversarial queries. Both our human assessment framework and dataset design process are grounded in an iterative participatory approach and review of possible biases in Med-PaLM 2 answers to adversarial queries. Through our empirical study, we find that the use of a collection of datasets curated through a variety of methodologies, coupled with a thorough evaluation protocol that leverages multiple assessment rubric designs and diverse rater groups, surfaces biases that may be missed via narrower evaluation approaches. Our experience underscores the importance of using diverse assessment methodologies and involving raters of varying backgrounds and expertise. We emphasize that while our framework can identify specific forms of bias, it is not sufficient to holistically assess whether the deployment of an AI system promotes equitable health outcomes. We hope the broader community leverages and builds on these tools and methods towards realizing a shared goal of LLMs that promote accessible and equitable healthcare for all.
View details
Creative ML Assemblages: The Interactive Politics of People, Processes, and Products
Ramya Malur Srinivasan
Katharina Burgdorf
Jennifer Lena
ACM Conference on Computer Supported Cooperative Work and Social Computing(2024) (to appear)
Preview abstract
Creative ML tools are collaborative systems that afford artistic creativity through their myriad interactive relationships. We propose using ``assemblage thinking" to support analyses of creative ML by approaching it as a system in which the elements of people, organizations, culture, practices, and technology constantly influence each other. We model these interactions as ``coordinating elements" that give rise to the social and political characteristics of a particular creative ML context, and call attention to three dynamic elements of creative ML whose interactions provide unique context for the social impact a particular system as: people, creative processes, and products. As creative assemblages are highly contextual, we present these as analytical concepts that computing researchers can adapt to better understand the functioning of a particular system or phenomena and identify intervention points to foster desired change. This paper contributes to theorizing interactions with AI in the context of art, and how these interactions shape the production of algorithmic art.
View details
Generative AI in Creative Practice: ML-Artist Folk Theories of T2I Use, Harm, and Harm-Reduction
Shalaleh Rismani
Proceedings of the CHI Conference on Human Factors in Computing Systems (CHI '24), Association for Computing Machinery(2024), pp. 1-17 (to appear)
Preview abstract
Understanding how communities experience algorithms is necessary to mitigate potential harmful impacts. This paper presents folk theories of text-to-image (T2I) models to enrich understanding of how artist communities experience creative machine learning (ML) systems. This research draws on data collected from a workshop with 15 artists from 10 countries who incorporate T2I models in their creative practice. Through reflexive thematic analysis of workshop data, we highlight theorization of T2I use, harm, and harm-reduction. Folk theories of use envision T2I models as an artistic medium, a mundane tool, and locate true creativity as rising above model affordances. Theories of harm articulate T2I models as harmed by engineering efforts to eliminate glitches and product policy efforts to limit functionality. Theories of harm-reduction orient towards protecting T2I models for creative practice through transparency and distributed governance. We examine how these theories relate, and conclude by discussing how folk theorization informs responsible AI efforts.
View details
Beyond the ML Model: Applying Safety Engineering Frameworks to Text-to-Image Development
Shalaleh Rismani
Renelito Delos Santos
AJung Moon
AIES 2023(2023)
Preview abstract
Inappropriate design and deployment of machine learning (ML) systems lead to negative downstream social and ethical impacts -- described here as social and ethical risks -- for users, society, and the environment. Despite the growing need to regulate ML systems, current processes for assessing and mitigating risks are disjointed and inconsistent. We interviewed 30 industry practitioners on their current social and ethical risk management practices and collected their first reactions on adapting safety engineering frameworks into their practice -- namely, System Theoretic Process Analysis (STPA) and Failure Mode and Effects Analysis (FMEA). Our findings suggest STPA/FMEA can provide an appropriate structure for social and ethical risk assessment and mitigation processes. However, we also find nontrivial challenges in integrating such frameworks in the fast-paced culture of the ML industry. We call on the CHI community to strengthen existing frameworks and assess their efficacy, ensuring that ML systems are safer for all people.
View details
Identifying Sociotechnical Harms of Algorithmic Systems: Scoping a Taxonomy for Harm Reduction
Shalaleh Rismani
Kathryn Henne
AJung Moon
Paul Nicholas
N'Mah Yilla-Akbari
Jess Gallegos
Emilio Garcia
Gurleen Virk
Proceedings of the 2023 AAAI/ACM Conference on AI, Ethics, and Society, Association for Computing Machinery, 723–741
Preview abstract
Understanding the broader landscape of potential harms from algorithmic systems enables practitioners to better anticipate consequences of the systems they build. It also supports the prospect of incorporating controls to help minimize harms that emerge from the interplay of technologies and social and cultural dynamics. A growing body of scholarship has identified a wide range of harms across different algorithmic and machine learning (ML) technologies. However, computing research and practitioners lack a high level and synthesized overview of harms from algorithmic systems arising at the micro-, meso-, and macro-levels of society. We present an applied taxonomy of sociotechnical harms to support more systematic surfacing of potential harms in algorithmic systems. Based on a scoping review of prior research on harms from AI systems (n=172), we identified five major themes related to sociotechnical harms — allocative, quality-of-service, representational, social system, and interpersonal harms. We describe these categories of harm, and present case studies that illustrate the usefulness of the taxonomy. We conclude with a discussion of challenges and under-explored areas of harm in the literature, which present opportunities for future research.
View details
Healthsheet: development of a transparency artifact for health datasets
Lauren Wilcox
Razvan Adrian Amironesei
Nyalleng Moorosi
ACM FAccT Conference 2022, ACM(2022)
Preview abstract
Machine learning (ML) approaches have demonstrated promising results in a wide range of healthcare applications. Data plays a crucial role in developing ML-based healthcare systems that directly affect people’s lives. Many of the ethical issues surrounding the use of ML in healthcare stem from structural inequalities underlying the way we collect, use, and handle data. Developing guidelines to improve documentation practices regarding the creation, use, and maintenance of ML healthcare datasets is therefore of critical importance. In this work, we introduce Healthsheet, a contextualized adaptation of the original datasheet questionnaire for health-specific applications. Through a series of semi-structured interviews, we adapt the datasheets for healthcare data documentation. As part of the Healthsheet development process and to understand the obstacles researchers face in creating datasheets, we worked with three publicly-available healthcare datasets as our case studies, each with different types of structured data: Electronic health Records (EHR), clinical trial study data, and smartphone-based performance outcome measures. Our findings from the interviewee study and case studies show 1) that datasheets should be contextualized for healthcare, 2) that despite incentives to adopt accountability practices such as datasheets, there is a lack of consistency in the broader use of these practices 3) how the ML for health community views datasheets and particularly Healthsheets as diagnostic tool to surface the limitations and strength of datasets and 4) the relative importance of different fields in the datasheet to healthcare concerns.
View details
Preview abstract
Testing, within the machine learning (ML) community, has been predominantly about assessing a learned model's predictive performance measured against a test dataset. This test dataset is often a held-out subset of the dataset used to train the model, and hence expected to follow the same data distribution as the training dataset. While recent work on robustness testing within ML has pointed to the importance of testing against distributional shifts, these efforts also focus on estimating the likelihood of the model making an error against a reference dataset/distribution. In this paper, we argue that this view of testing actively discourages researchers and developers from looking into many other sources of robustness failures, for instance corner cases. We draw parallels with decades of work within software engineering testing focused on assessing a software system against various stress conditions, including corner cases, as opposed to solely focusing on average-case behaviour. Finally, we put forth a set of recommendations to broaden the view of machine learning testing to a rigorous practice.
View details
Preview abstract
In order to build trust that a machine learned model is appropriate and responsible within a systems context involving technical and
human components, a broad range of factors typically need to be considered. However in practice model evaluations frequently focus
on only a narrow range of expected predictive behaviours. This paper examines the critical evaluation gap between the idealized
breadth of concerns and the observed narrow focus of actual evaluations. In doing so, we demonstrate which values are centered—and
which are marginalized—within the machine learning community. Through an empirical study of machine learning papers from
recent high profile conferences, we demonstrate the discipline’s general focus on a small set of evaluation methods. By considering the
mathematical formulations of evaluation metrics and the test datasets over which they are calculated, we draw attention to which
properties of models are centered in the field. This analysis also reveals an important gap: the properties of models which are frequently
neglected or sidelined during evaluation. By studying the structure of this gap, we demonstrate the machine learning discipline’s
implicit assumption of a range of commitments which have normative impacts; these include commitments to consequentialism,
abstractability from context, the quantifiability of impacts, the irrelevance of non-predictive features, and the equivalence of different
failure modes. Shedding light on these assumptions and commitments enables us to question their appropriateness for different ML
system contexts, and points the way towards more diverse and contextualized evaluation methodologies which can be used to more
robustly examine the trustworthiness of ML models.
View details