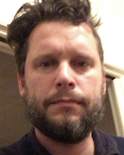
Andrew Smart
I'm a researcher in the Responsible AI Impact Lab working on machine learning fairness and the governance of AI. My background is in anthropology, philosophy, cognitive science and brain imaging. I'm interested in the relationships between social ontology, causality and how to estimate the risks and impacts of using machine learning in high-stakes domains.
Authored Publications
Google Publications
Other Publications
Sort By
Preview abstract
Large technology firms face the problem of moderating content on their platforms for compliance with laws and policies. To accomplish this at the scale of billions of pieces of content per day, a combination of human and machine review are necessary to label content. However, human error and subjective methods of measure are inherent in many audit procedures. This paper introduces statistical analysis methods and mathematical techniques to determine, quantify, and minimize these sources of risk. Through these methodologies it can be shown that we are able to reduce reviewer bias.
View details
Identifying Sociotechnical Harms of Algorithmic Systems: Scoping a Taxonomy for Harm Reduction
Shalaleh Rismani
Kathryn Henne
AJung Moon
Paul Nicholas
N'Mah Yilla-Akbari
Jess Gallegos
Emilio Garcia
Gurleen Virk
Proceedings of the 2023 AAAI/ACM Conference on AI, Ethics, and Society, Association for Computing Machinery, 723–741
Preview abstract
Understanding the broader landscape of potential harms from algorithmic systems enables practitioners to better anticipate consequences of the systems they build. It also supports the prospect of incorporating controls to help minimize harms that emerge from the interplay of technologies and social and cultural dynamics. A growing body of scholarship has identified a wide range of harms across different algorithmic and machine learning (ML) technologies. However, computing research and practitioners lack a high level and synthesized overview of harms from algorithmic systems arising at the micro-, meso-, and macro-levels of society. We present an applied taxonomy of sociotechnical harms to support more systematic surfacing of potential harms in algorithmic systems. Based on a scoping review of prior research on harms from AI systems (n=172), we identified five major themes related to sociotechnical harms — allocative, quality-of-service, representational, social system, and interpersonal harms. We describe these categories of harm, and present case studies that illustrate the usefulness of the taxonomy. We conclude with a discussion of challenges and under-explored areas of harm in the literature, which present opportunities for future research.
View details
Beyond the ML Model: Applying Safety Engineering Frameworks to Text-to-Image Development
Shalaleh Rismani
Renelito Delos Santos
AJung Moon
AIES 2023 (2023)
Preview abstract
Inappropriate design and deployment of machine learning (ML) systems lead to negative downstream social and ethical impacts -- described here as social and ethical risks -- for users, society, and the environment. Despite the growing need to regulate ML systems, current processes for assessing and mitigating risks are disjointed and inconsistent. We interviewed 30 industry practitioners on their current social and ethical risk management practices and collected their first reactions on adapting safety engineering frameworks into their practice -- namely, System Theoretic Process Analysis (STPA) and Failure Mode and Effects Analysis (FMEA). Our findings suggest STPA/FMEA can provide an appropriate structure for social and ethical risk assessment and mitigation processes. However, we also find nontrivial challenges in integrating such frameworks in the fast-paced culture of the ML industry. We call on the CHI community to strengthen existing frameworks and assess their efficacy, ensuring that ML systems are safer for all people.
View details
Healthsheet: development of a transparency artifact for health datasets
Lauren Wilcox
Razvan Adrian Amironesei
Nyalleng Moorosi
ACM FAccT Conference 2022, ACM (2022)
Preview abstract
Machine learning (ML) approaches have demonstrated promising results in a wide range of healthcare applications. Data plays a crucial role in developing ML-based healthcare systems that directly affect people’s lives. Many of the ethical issues surrounding the use of ML in healthcare stem from structural inequalities underlying the way we collect, use, and handle data. Developing guidelines to improve documentation practices regarding the creation, use, and maintenance of ML healthcare datasets is therefore of critical importance. In this work, we introduce Healthsheet, a contextualized adaptation of the original datasheet questionnaire for health-specific applications. Through a series of semi-structured interviews, we adapt the datasheets for healthcare data documentation. As part of the Healthsheet development process and to understand the obstacles researchers face in creating datasheets, we worked with three publicly-available healthcare datasets as our case studies, each with different types of structured data: Electronic health Records (EHR), clinical trial study data, and smartphone-based performance outcome measures. Our findings from the interviewee study and case studies show 1) that datasheets should be contextualized for healthcare, 2) that despite incentives to adopt accountability practices such as datasheets, there is a lack of consistency in the broader use of these practices 3) how the ML for health community views datasheets and particularly Healthsheets as diagnostic tool to surface the limitations and strength of datasets and 4) the relative importance of different fields in the datasheet to healthcare concerns.
View details
Towards Accountability for Machine Learning Datasets
Alex Hanna
Christina Greer
Margaret Mitchell
Proceedings of FAccT 2021 (2021) (to appear)
Preview abstract
Rising concern for the societal implications of artificial intelligence systems has inspired demands for greater transparency and accountability. However the datasets which empower machine learning are often used, shared and re-used with little visibility into the processes of deliberation which led to their creation. Which stakeholder groups had their perspectives included when the dataset was conceived? Which domain experts were consulted regarding how to model subgroups and other phenomena? How were questions of representational biases measured and addressed? Who labeled the data? In this paper, we introduce a rigorous framework for dataset development transparency which supports decision-making and accountability. The framework uses the cyclical, infrastructural and engineering nature of dataset development to draw on best practices from the software development lifecycle. Each stage of the data development lifecycle yields a set of documents that facilitate improved communication and decision-making, as well as drawing attention the value and necessity of careful data work. The proposed framework is intended to contribute to closing the accountability gap in artificial intelligence systems, by making visible the often overlooked work that goes into dataset creation.
View details
The Use and Misuse of Counterfactuals in Ethical Machine Learning
Atoosa Kasirzadeh
FAccT '21: Proceedings of the 2021 ACM Conference on Fairness, Accountability, and TransparencyMarch 2021 (2021)
Preview abstract
The use of counterfactuals for considerations of algorithmic
fairness and explainability is gaining prominence within the
machine learning community and industry. This paper argues for more caution with the use of counterfactuals when
the facts to be considered are social categories such as race or
gender. We review a broad body of papers from philosophy
and social sciences on social ontology and the semantics of
counterfactuals, and we conclude that the counterfactual approach in machine learning fairness and social explainability
can require an incoherent theory of what social categories are.
Our findings suggest that most often the social categories may
not admit counterfactual manipulation, and hence may not
appropriately satisfy the demands for evaluating the truth or
falsity of counterfactuals. This is important because the widespread use of counterfactuals in machine learning can lead
to misleading results when applied in high-stakes domains.
Accordingly, we argue that even though counterfactuals play
an essential part in some causal inferences, their use for questions of algorithmic fairness and social explanations can create more problems than they resolve. Our positive result is
a set of tenets about using counterfactuals for fairness and
explanations in machine learning.
View details
Preview abstract
In response to growing concerns of bias, discrimination, and unfairness perpetuated by algorithmic systems, the datasets used to train and evaluate machine learning models have come under increased scrutiny. Many of these examinations have focused on the contents of machine learning datasets, finding glaring underrepresentation of minoritized groups. In contrast, relatively little work has been done to examine the norms, values, and assumptions embedded in these datasets. In this work, we conceptualize machine learning datasets as a type of informational infrastructure, and motivate a genealogy as method in examining the histories and modes of constitution at play in their creation. We present a critical history of ImageNet as an exemplar, utilizing critical discourse analysis of major texts around ImageNet’s creation and impact. We find that assumptions around ImageNet and other large computer vision datasets more generally rely on three themes: the aggregation and accumulation of more data, the computational construction of meaning, and making certain types of data labor invisible. By tracing the discourses that surround this influential benchmark, we contribute to the ongoing development of the standards and norms around data development in machine learning and artificial intelligence research.
View details
Preview abstract
In this paper we argue that standard calls for explainability that focus on the epistemic inscrutability of black-box machine learning models may be misplaced. If we presume, for the sake of this paper, that machine learning can be a source of knowledge, then it makes sense to wonder what kind of justification it involves. How do we rationalize on the one hand the seeming justificatory black box with the observed widespread adoption of machine learning? We argue that, in general, people implicitly adopt reliabilism regarding machine learning. Reliabilism is an epistemological theory of epistemic justification according to which a belief is warranted if it has been produced by a reliable process or method. We argue that, in cases where model deployments require moral justification, reliabilism is not sufficient, and instead justifying deployment requires establishing robust human processes as a moral “wrapper” around machine outputs. We then suggest that, in certain high-stakes domains with moral consequences, reliabilism does not provide another kind of necessary justification—moral justification. Finally, we offer cautions relevant to the (implicit or explicit)adoption of the reliabilist interpretation of machine learning.
View details
Extending the Machine Learning Abstraction Boundary: A Complex Systems Approach to Incorporate Societal Context
Jill Kuhlberg
William Samuel Isaac
arXiv (2020), pp. 11
Preview abstract
Machine learning (ML) fairness research tends to focus primarily on mathematically-based interventions on often opaque algorithms or models and/or their immediate inputs and outputs. Recent re-search has pointed out the limitations of fairness approaches that rely on oversimplified mathematical models that abstract away the underlying societal context where models are ultimately deployed and from which model inputs and complex socially constructed concepts such as fairness originate. In this paper, we outline three new tools to improve the comprehension, identification and representation of societal context. First, we propose a complex adaptive systems(CAS) based model and definition of societal context that may help researchers and product developers expand the abstraction boundary of ML fairness work to include societal context. Second, we introduce collaborative causal theory formation (CCTF)as a key capability for establishing a socio-technical frame that incorporates diverse mental models and associated causal theories in modeling the problem and solution space for ML-based products. Finally, we identify system dynamics (SD) as an established, transparent and rigorous framework for practicing CCTF during all phases of the ML product development process. We conclude with a discussion of how these systems-based approaches to understanding the societal context within which socio-technical systems are embedded can improve the development of fair and inclusive ML-based products.
View details
Towards a Critical Race Methodology in Algorithmic Fairness
Alex Hanna
ACM Conference on Fairness, Accountability, and Transparency (ACM FAT*) (2020)
Preview abstract
We examine the way race and racial categories are adopted in algorithmic fairness frameworks. Current methodologies fail to adequately account for the socially constructed nature of race, instead adopting a conceptualization of race as a fixed attribute. Treating race as an attribute, rather than a structural, institutional, and relational phenomenon, can serve to minimize the structural aspects of algorithmic unfairness. In this work, we focus on the history of racial categories and turn to critical race theory and sociological work on race and ethnicity to ground conceptualizations of race for fairness research, drawing on lessons from public health, biomedical research, and social survey research. We argue that algorithmic fairness researchers need to take into account the multidimensionality of race, take seriously the processes of conceptualizing and operationalizing race, focus on social processes which produce racial inequality, and consider perspectives of those most affected by sociotechnical systems.
View details
Participatory Problem Formulation for Fairer Machine Learning Through Community Based System Dynamics
Jill Kuhlberg
William Samuel Isaac
Machine Learning in Real Life (ML-IRL) ICLR 2020 Workshop (2020), pp. 6
Preview abstract
Recent research on algorithmic fairness has highlighted that the problem formulation phase of ML system development can be a key source of bias that has significant downstream impacts on ML system fairness outcomes. However, very little attention has been paid to methods for improving the fairness efficacy of this critical phase of ML system development. Current practice neither accounts for the dynamic complexity of high-stakes domains nor incorporates the perspectives of vulnerable stakeholders. In this paper we introduce community based system dynamics (CBSD) as an approach to enable the participation of typically excluded stakeholders in the problem formulation phase of the ML system development process and facilitate the deep problem understanding required to mitigate bias during this crucial stage.
View details
Closing the AI accountability gap: defining an end-to-end framework for internal algorithmic auditin
Becky White
Inioluwa Deborah Raji
Margaret Mitchell
Timnit Gebru
FAT* Barcelona, 2020, ACM Conference on Fairness, Accountability, and Transparency (ACM FAT* (2020)
Preview abstract
Rising concern for the societal implications of artificial intelligencesystems has inspired a wave of academic and journalistic literaturein which deployed systems are audited for harm by investigatorsfrom outside the organizations deploying the algorithms. However,it remains challenging for practitioners to identify the harmfulrepercussions of their own systems prior to deployment, and, oncedeployed, emergent issues can become difficult or impossible totrace back to their source.In this paper, we introduce a framework for algorithmic auditingthat supports artificial intelligence system development end-to-end,to be applied throughout the internal organization development life-cycle. Each stage of the audit yields a set of documents that togetherform an overall audit report, drawing on an organization’s valuesor principles to assess the fit of decisions made throughout the pro-cess. The proposed auditing framework is intended to contribute toclosing theaccountability gapin the development and deploymentof large-scale artificial intelligence systems by embedding a robustprocess to ensure audit integrity.
View details
Fairness Preferences, Actual and Hypothetical: A Study of Crowdworker Incentives
Angie Peng
Jeff Naecker
Nyalleng Moorosi
Proceedings of ICML 2020 Workshop on Participatory Approaches to Machine Learning (to appear)
Preview abstract
How should we decide which fairness criteria or
definitions to adopt in machine learning systems?
To answer this question, we must study the fair-
ness preferences of actual users of machine learn-
ing systems. Stringent parity constraints on treat-
ment or impact can come with trade-offs, and
may not even be preferred by the social groups
in question (Zafar et al., 2017). Thus it might
be beneficial to elicit what the group’s prefer-
ences are, rather than rely on a priori defined
mathematical fairness constraints. Simply asking
for self-reported rankings of users is challenging
because research has shown that there are often
gaps between people’s stated and actual prefer-
ences(Bernheim et al., 2013).
This paper outlines a research program and ex-
perimental designs for investigating these ques-
tions. Participants in the experiments are invited
to perform a set of tasks in exchange for a base
payment—they are told upfront that they may
receive a bonus later on, and the bonus could de-
pend on some combination of output quantity and
quality. The same group of workers then votes on
a bonus payment structure, to elicit preferences.
The voting is hypothetical (not tied to an outcome)
for half the group and actual (tied to the actual
payment outcome) for the other half, so that we
can understand the relation between a group’s
actual preferences and hypothetical (stated) pref-
erences. Connections and lessons from fairness
in machine learning are explored.
View details
Bringing the People Back In: Contesting Benchmark Machine Learning Datasets
Alex Hanna
Razvan Amironesei
Hilary Nicole
Morgan Klaus Scheuerman
Participatory Approaches to Machine Learning, ICML 2020 Workshop (2020)
Preview abstract
In response to algorithmic unfairness embedded in sociotechnical systems, significant attention has been focused on the contents of machine learning datasets which have revealed biases towards white, cisgender, male, and Western data subjects. In contrast, comparatively less attention has been paid to the histories, values, and norms embedded in such datasets. In this work, we outline a research program - a genealogy of machine learning data - for investigating how and why these datasets have been created, what and whose values influence the choices of data to collect, the contextual and contingent conditions of their creation. We describe the ways in which benchmark datasets in machine learning operate as infrastructure and pose four research questions for these datasets. This interrogation forces us to "bring the people back in" by aiding us in understanding the labor embedded in dataset construction, and thereby presenting new avenues of contestation for other researchers encountering the data.
View details
No Results Found