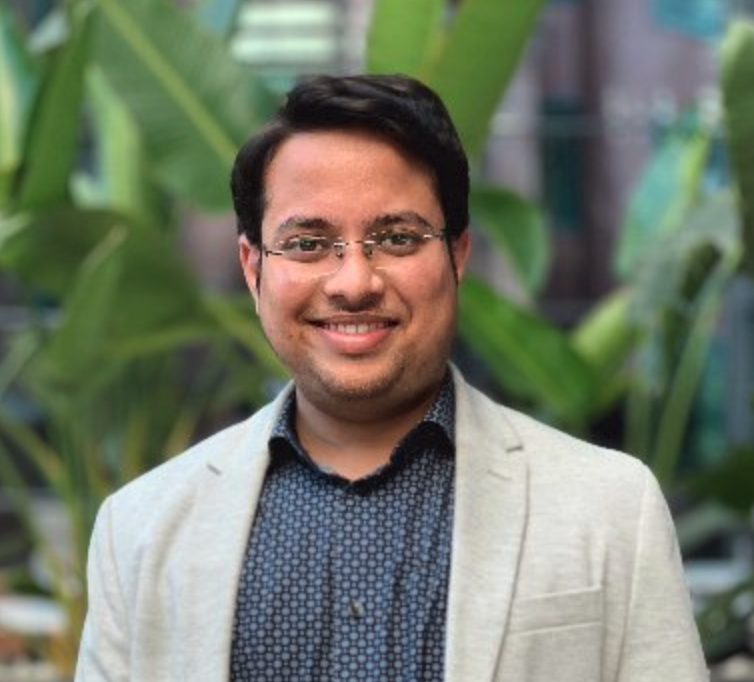
Subhrajit Roy
Subhrajit is a research scientist at Google Brain where he focuses on developing machine learning systems for analysing health data. He has co-developed novel sparse multi-task deep neural networks for adverse event prediction in hospitals and is currently focusing on building inclusive healthcare technologies. Previously, he has worked at IBM Research Australia for three years as a research staff member. During this time, he co-designed portable machine learning systems for seizure management in epileptic patients. Recognizing the potential of this work, Forbes magazine included him in their prestigious 30 Under 30 list. He has authored 35+ scientific papers. His work has been cited 1000+ times and featured in IEEE Spectrum, IEEE Pulse, VentureBeat, The World Economic Forum, amongst others. He also serves as an Associate Editor of the Neuromorphic Engineering section of Frontiers in Neuroscience and a reviewer for several AI conferences. He earned his BE degree from Jadavpur University, India and PhD from Nanyang Technological University, Singapore.
Research Areas
Authored Publications
Sort By
Batch Calibration: Rethinking Calibration For In-Context Learning And Prompt Engineering
Han Zhou
Lev Proleev
International Conference on Learning Representations (ICLR)(2024)
Preview abstract
Prompting and in-context learning (ICL) have become efficient learning paradigms for large language models (LLMs). However, LLMs suffer from prompt brittleness and various bias factors in the prompt, including but not limited to the formatting, the choice verbalizers, and the ICL examples. To address this problem that results in unexpected performance degradation, calibration methods have been developed to mitigate the effects of these biases while recovering LLM performance. In this work, we first conduct a systematic analysis of the existing calibration methods, where we both provide a unified view and reveal the failure cases. Inspired by these analyses, we propose Batch Calibration (BC), a simple yet intuitive method that controls the contextual bias from the batched input, unifies various prior approaches, and effectively addresses the aforementioned issues. BC is zero-shot, inference-only, and incurs negligible additional costs. In the few-shot setup, we further extend BC to allow it to learn the contextual bias from labeled data. We validate the effectiveness of BC with PaLM 2-(S, M, L) and CLIP models and demonstrate state-of-the-art performance over previous calibration baselines across more than 10 natural language understanding and image classification tasks.
View details
Healthsheet: development of a transparency artifact for health datasets
Lauren Wilcox
Razvan Adrian Amironesei
Nyalleng Moorosi
ACM FAccT Conference 2022, ACM(2022)
Preview abstract
Machine learning (ML) approaches have demonstrated promising results in a wide range of healthcare applications. Data plays a crucial role in developing ML-based healthcare systems that directly affect people’s lives. Many of the ethical issues surrounding the use of ML in healthcare stem from structural inequalities underlying the way we collect, use, and handle data. Developing guidelines to improve documentation practices regarding the creation, use, and maintenance of ML healthcare datasets is therefore of critical importance. In this work, we introduce Healthsheet, a contextualized adaptation of the original datasheet questionnaire for health-specific applications. Through a series of semi-structured interviews, we adapt the datasheets for healthcare data documentation. As part of the Healthsheet development process and to understand the obstacles researchers face in creating datasheets, we worked with three publicly-available healthcare datasets as our case studies, each with different types of structured data: Electronic health Records (EHR), clinical trial study data, and smartphone-based performance outcome measures. Our findings from the interviewee study and case studies show 1) that datasheets should be contextualized for healthcare, 2) that despite incentives to adopt accountability practices such as datasheets, there is a lack of consistency in the broader use of these practices 3) how the ML for health community views datasheets and particularly Healthsheets as diagnostic tool to surface the limitations and strength of datasets and 4) the relative importance of different fields in the datasheet to healthcare concerns.
View details
Diagnosing failures of fairness transfer across distribution shift in real-world medical settings
Sanmi Koyejo
Eva Schnider
Krista Opsahl-Ong
Alex Brown
Christina Chen
Silvia Chiappa
Proceedings of Neural Information Processing Systems 2022(2022)
Preview abstract
Diagnosing and mitigating changes in model fairness under distribution shift is an important component of the safe deployment of machine learning in healthcare settings. Importantly, the success of any mitigation strategy strongly depends on the structure of the shift. Despite this, there has been little discussion of how to empirically assess the structure of a distribution shift that one is encountering in practice. In this work, we adopt a causal framing to motivate conditional independence tests as a key tool for characterizing distribution shifts. Using our approach in two medical applications, we show that this knowledge can help diagnose failures of fairness transfer, including cases where real-world shifts are more complex than is often assumed in the literature. Based on these results, we discuss potential remedies at each step of the machine learning pipeline.
View details
Multi-task prediction of organ dysfunction in the ICU using sequential sub-network routing
Eric Loreaux
Anne Mottram
Hugh Montgomery
Ali Connell
Nenad Tomašev
Martin Seneviratne
Journal of the American Medical Informatics Association (JAMIA)(2021)
Preview abstract
Introduction:
Multi-task learning (MTL) using electronic health records (EHRs) allows concurrent prediction of multiple endpoints. MTL has shown promise in improving model performance and training efficiency; however it often suffers from negative transfer - impaired learning if tasks are not appropriately selected. We introduce a sequential sub-network routing (SeqSNR) architecture which uses soft parameter sharing to find related tasks and encourage cross-learning between them.
Materials and Methods:
Using the Medical Information Mart for Intensive Care (MIMIC-III) dataset, we train deep neural network models to predict the onset of six endpoints including specific organ dysfunctions and general clinical outcomes: acute kidney injury, continuous renal replacement therapy, mechanical ventilation, vasoactive medications, mortality, and length of stay. We compare single task models (ST) with naive multi-task (shared bottom, SB) and SeqSNR in terms of discriminative performance and label efficiency.
Results:
SeqSNR showed a modest yet statistically significant performance boost across at least 4 out of 6 tasks compared to SB and ST. When the size of the training dataset was reduced for a given task, SeqSNR outperformed ST for all cases showing an average AU PRC boost of 2.1%, 2.9%, and 2.1% for tasks using 1%, 5%, and 10% of labels respectively.
Discussion and Conclusion:
Multi-task learning has variable performance compared to single-task learning, with the possibility for negative transfer. The SeqSNR architecture outperforms SB and ST in discriminative performance and shows superior performance in terms of label efficiency. SeqSNR should be considered for multi-task predictive modeling using EHR data.
View details