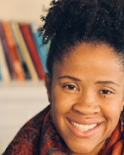
Jamila Smith-Loud
Jamila's research primarily focuses on social, equity and human rights related assessments to support and help shape how Google puts values such as ethics, fairness and social benefit into action.
Prior to joining Google, Jamila was the Manager of Strategic Initiatives at a Los Angeles-based civil rights nonprofit, Advancement Project, where she supported the development of racial equity initiatives and policy development through research, analysis, and advocacy. Jamila was also a Fellow with the Political and Legal Anthropology Review Journal where her research focused on rights based discourse and the intersections of law, power, identity, and cultural change. Jamila was born and raised in Los Angeles, and is a graduate of UC Berkeley and Howard University School of Law.
Research Areas
Authored Publications
Google Publications
Other Publications
Sort By
A Toolbox for Surfacing Health Equity Harms and Biases in Large Language Models
Heather Cole-Lewis
Nenad Tomašev
Liam McCoy
Leo Anthony Celi
Alanna Walton
Akeiylah DeWitt
Philip Mansfield
Sushant Prakash
Joelle Barral
Ivor Horn
Karan Singhal
Arxiv (2024) (to appear)
Preview abstract
Large language models (LLMs) hold immense promise to serve complex health information needs but also have the potential to introduce harm and exacerbate health disparities. Reliably evaluating equity-related model failures is a critical step toward developing systems that promote health equity. In this work, we present resources and methodologies for surfacing biases with potential to precipitate equity-related harms in long-form, LLM-generated answers to medical questions and then conduct an empirical case study with Med-PaLM 2, resulting in the largest human evaluation study in this area to date. Our contributions include a multifactorial framework for human assessment of LLM-generated answers for biases, and EquityMedQA, a collection of seven newly-released datasets comprising both manually-curated and LLM-generated questions enriched for adversarial queries. Both our human assessment framework and dataset design process are grounded in an iterative participatory approach and review of possible biases in Med-PaLM 2 answers to adversarial queries. Through our empirical study, we find that the use of a collection of datasets curated through a variety of methodologies, coupled with a thorough evaluation protocol that leverages multiple assessment rubric designs and diverse rater groups, surfaces biases that may be missed via narrower evaluation approaches. Our experience underscores the importance of using diverse assessment methodologies and involving raters of varying backgrounds and expertise. We emphasize that while our framework can identify specific forms of bias, it is not sufficient to holistically assess whether the deployment of an AI system promotes equitable health outcomes. We hope the broader community leverages and builds on these tools and methods towards realizing a shared goal of LLMs that promote accessible and equitable healthcare for all.
View details
Preview abstract
In the last few months racial violence in the US context has been increasingly visible and salient for some parts of the population who have been long shielded from this reality. For others this reality is an ever present aspect of life along with the understanding that the adoption of fair procedures and the exposing of values of formal equality by politicians, bureaucrats and even corporate leaders often have no logical or rational connection to actual justice. Fairness and justice are terms that are often used in a way that makes them indistinguishable from each other, particularly in the context of AI/ML Fairness. But what we know from decades long fights against discrimination, racism and inequity outcomes can be fair without being just.
Increasing scholarship in AI Ethics and ML Fairness are examining and considering various perceptions and definitions of fairness. This definitional approach fails to critically assess the inherent implications of fairness constructs, which is conceptually rooted in notions of formal equality and procedural conceptions of justice. This approach and understanding of fairness misses the opportunity to assess and understand potential harms, as well the substantive justice approach which could lead to not only different outcomes but also different measurement approaches.
When thinking through a parallel of “fairness” as procedural justice with regard to Brown v. Board of Education (1955) the argument and ultimately the legal victory resulted in ensuring a process for school desegregation but soon revealed that it failed to provide adequate and quality education to predominantly black schools with resulting in decades long denial of the economic and employment opportunities that are intrinsically linked to receiving a good education.
We argue that "fairness" has become a red herring in the discussion of AI and data ethics.
Fairness has focused on making liberal claims about the "amount of fairness" that a system can contain. As a result rights-claiming then becomes a focus on questions of quantities (equality of odds, demographic parity) and not substantive advancement.
View details
Closing the AI accountability gap: defining an end-to-end framework for internal algorithmic auditin
Becky White
Inioluwa Deborah Raji
Margaret Mitchell
Timnit Gebru
FAT* Barcelona, 2020, ACM Conference on Fairness, Accountability, and Transparency (ACM FAT* (2020)
Preview abstract
Rising concern for the societal implications of artificial intelligencesystems has inspired a wave of academic and journalistic literaturein which deployed systems are audited for harm by investigatorsfrom outside the organizations deploying the algorithms. However,it remains challenging for practitioners to identify the harmfulrepercussions of their own systems prior to deployment, and, oncedeployed, emergent issues can become difficult or impossible totrace back to their source.In this paper, we introduce a framework for algorithmic auditingthat supports artificial intelligence system development end-to-end,to be applied throughout the internal organization development life-cycle. Each stage of the audit yields a set of documents that togetherform an overall audit report, drawing on an organization’s valuesor principles to assess the fit of decisions made throughout the pro-cess. The proposed auditing framework is intended to contribute toclosing theaccountability gapin the development and deploymentof large-scale artificial intelligence systems by embedding a robustprocess to ensure audit integrity.
View details
Towards a Critical Race Methodology in Algorithmic Fairness
Alex Hanna
ACM Conference on Fairness, Accountability, and Transparency (ACM FAT*) (2020)
Preview abstract
We examine the way race and racial categories are adopted in algorithmic fairness frameworks. Current methodologies fail to adequately account for the socially constructed nature of race, instead adopting a conceptualization of race as a fixed attribute. Treating race as an attribute, rather than a structural, institutional, and relational phenomenon, can serve to minimize the structural aspects of algorithmic unfairness. In this work, we focus on the history of racial categories and turn to critical race theory and sociological work on race and ethnicity to ground conceptualizations of race for fairness research, drawing on lessons from public health, biomedical research, and social survey research. We argue that algorithmic fairness researchers need to take into account the multidimensionality of race, take seriously the processes of conceptualizing and operationalizing race, focus on social processes which produce racial inequality, and consider perspectives of those most affected by sociotechnical systems.
View details
No Results Found