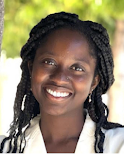
Mercy Nyamewaa Asiedu
I am a research scientist at Google Research, and my research interests lie in developing fair and robust algorithms for equitable health in underserved settings. Before Google, I was a Schmidt Science Fellow at MIT, where I worked on different machine learning areas in medical imaging and clinical NLP. I obtained my PhD from Duke University and my thesis focused on developing devices and algorithms for cervical cancer screening in underserved settings.
Research Areas
Authored Publications
Google Publications
Other Publications
Sort By
A Toolbox for Surfacing Health Equity Harms and Biases in Large Language Models
Heather Cole-Lewis
Nenad Tomašev
Liam McCoy
Leo Anthony Celi
Mike Schaekermann
Alanna Walton
Akeiylah DeWitt
Philip Mansfield
Sushant Prakash
Joelle Barral
Ivor Horn
Karan Singhal
Arxiv (2024) (to appear)
Preview abstract
Large language models (LLMs) hold immense promise to serve complex health information needs but also have the potential to introduce harm and exacerbate health disparities. Reliably evaluating equity-related model failures is a critical step toward developing systems that promote health equity. In this work, we present resources and methodologies for surfacing biases with potential to precipitate equity-related harms in long-form, LLM-generated answers to medical questions and then conduct an empirical case study with Med-PaLM 2, resulting in the largest human evaluation study in this area to date. Our contributions include a multifactorial framework for human assessment of LLM-generated answers for biases, and EquityMedQA, a collection of seven newly-released datasets comprising both manually-curated and LLM-generated questions enriched for adversarial queries. Both our human assessment framework and dataset design process are grounded in an iterative participatory approach and review of possible biases in Med-PaLM 2 answers to adversarial queries. Through our empirical study, we find that the use of a collection of datasets curated through a variety of methodologies, coupled with a thorough evaluation protocol that leverages multiple assessment rubric designs and diverse rater groups, surfaces biases that may be missed via narrower evaluation approaches. Our experience underscores the importance of using diverse assessment methodologies and involving raters of varying backgrounds and expertise. We emphasize that while our framework can identify specific forms of bias, it is not sufficient to holistically assess whether the deployment of an AI system promotes equitable health outcomes. We hope the broader community leverages and builds on these tools and methods towards realizing a shared goal of LLMs that promote accessible and equitable healthcare for all.
View details
Machine learning for healthcare: A bibliometric study of contributions from Africa
Houcemeddine Turki
Anastassios Pouris
Francis-Alfred
Michaelangelo Ifeanyichukwu
Catherine Namayega
Mohamed Ali Hadj Taieb
Sadiq Adewale Adedayo
Chris Fourie
Christopher Brian Currin
Atnafu Lambebo Tonja
Abraham Toluwase Owodunni
Abdulhameed Dere
Chris Chinenye Emezue
Shamsudden Hassan Muhammad
Muhammad Musa Isa
Mohamed Ben Aouicha
Preprints (2023)
Preview abstract
Machine learning has seen enormous growth in the last decade, with healthcare being a
prime application for advanced diagnostics and improved patient care. The application of machine learning for healthcare is particularly pertinent in Africa, where many countries are resource-scarce. However, it is unclear how much research on this topic is arising from African institutes themselves, which is a crucial aspect for applications of machine learning to unique contexts and challenges on the continent. Here, we conduct a bibliometric study of African contributions to research publications related to machine learning for healthcare, as indexed in Scopus, between 1993 and 2022. We identified 3,772 research outputs, with most of these published since 2020.
North African countries currently lead the way with 64.5% of publications for the reported period, yet Sub-Saharan Africa is rapidly increasing its output. We found that international support in the form of funding and collaborations is correlated with research output generally for the continent, with local support garnering less attention. Understanding African research contributions to machine learning for healthcare is a crucial first step in surveying the broader academic landscape, forming stronger research communities, and providing advanced and contextually aware biomedical access to Africa.
View details
A framework for grassroots research collaboration in machine learning and global health
Atnafu Lambebo Tonja
Benjamin Rosman
Chris Emezue
Chris Fourie
Christopher Brian Currin
Daphne Machangara
Houcemeddine Turki
Jade Abbott
Marvellous Ajala
Mennatullah Siam
Sadiq Adewale Adedayo
International Conference on Learning Representations (2023)
Preview abstract
Traditional top-down approaches for global health have historically failed to
achieve social progress (Hoffman et al., 2015; Hoffman & Røttingen, 2015). However, recently, a more holistic, multi-level approach, One Health (OH) (Osterhaus
et al., 2020), is being adopted. Several challenges have been identified for the
implementation of OH (dos S. Ribeiro et al., 2019), including policy and funding,
education and training, and multi-actor, multi-domain, and multi-level collaborations. This is despite the increasing accessibility to knowledge and digital research
tools through the internet. To address some of these challenges, we propose a general framework for grassroots community-based means of participatory research.
Additionally, we present a specific roadmap to create a Machine Learning for
Global Health community in Africa. The proposed framework aims to enable any
small group of individuals with scarce resources to build and sustain an online
community within approximately two years. We provide a discussion of the potential impact of the proposed framework on global health research collaborations.
View details
No Results Found