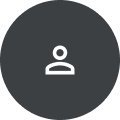
Cynthia Bennett
Research Areas
Authored Publications
Sort By
Preview abstract
Generative AI (GAI) is proliferating, and among its many applications are to support creative work (e.g., generating text, images, music) and to enhance
accessibility (e.g., captions of images and audio). As GAI evolves, creatives must consider how (or how not) to incorporate these tools into their practices.
In this paper, we present interviews at the intersection of these applications. We learned from 10 creatives with disabilities who intentionally use and
do not use GAI in and around their creative work. Their mediums ranged from audio engineering to leatherwork, and they collectively experienced a variety
of disabilities, from sensory to motor to invisible disabilities. We share cross-cutting themes of their access hacks, how creative practice and access
work become entangled, and their perspectives on how GAI should and should not ft into their workfows. In turn, we ofer qualities of accessible creativity
with responsible AI that can inform future research.
View details
“They only care to show us the wheelchair”: disability representation in text-to-image AI models
Avery Mack
Rida Qadri
CHI Conference on Human-Computer Interaction(2024)
Preview abstract
This paper reports on disability representation in images output from text-to-image (T2I) generative AI systems. Through eight focus groups with 25 people
with disabilities, we found that models repeatedly presented reductive archetypes for different disabilities. Often these representations reflected broader
societal stereotypes and biases, which our participants were concerned to see reproduced through T2I. Our participants discussed further challenges with
using these models including the current reliance on prompt engineering to reach satisfactorily diverse results. Finally, they offered suggestions for
how to improve disability representation with solutions like showing multiple, heterogeneous images for a single prompt and including the prompt with images
generated. Our discussion reflects on tensions and tradeoffs we found among the diverse perspectives shared to inform future research on representation-oriented
generative AI system evaluation metrics and development processes.
View details
Understanding Use Cases for AI-Powered Visual Interpretation Services
Ricardo Gonzalez
Jazmin Collins
Shiri Azenkot
CHI Conference on Human-Computer Interaction(2024)
Preview abstract
"Scene description" applications that describe visual content in a photo are useful daily tools for blind and low vision (BLV) people. Researchers have
studied their use, but they have only explored those that leverage remote sighted assistants; little is known about applications that use AI to generate
their descriptions. Thus, to investigate their use cases, we conducted a two-week diary study where 16 BLV participants used an AI-powered scene description
application we designed. Through their diary entries and follow-up interviews, users shared their information goals and assessments of the visual descriptions
they received. We analyzed the entries and found frequent use cases, such as identifying visual features of known objects, and surprising ones, such as avoiding contact with dangerous objects. We also found users scored the descriptions relatively low on average,
2.76 out of 5 (SD=1.49) for satisfaction and 2.43 out of 4 (SD=1.16) for trust, showing that descriptions still need signifcant improvements to deliver
satisfying and trustworthy experiences. We discuss future opportunities for AI as it becomes a more powerful accessibility tool for BLV users.
View details
From Provenance to Aberrations: Image Creator and Screen Reader User Perspectives on Alt Text for AI-Generated Images
Maitraye Das
Alexander J. Fiannaca
CHI Conference on Human Factors in Computing Systems(2024)
Preview abstract
AI-generated images are proliferating as a new visual medium. However, state-of-the-art image generation models do not output alternative (alt) text with
their images, rendering them largely inaccessible to screen reader users (SRUs). Moreover, less is known about what information would be most desirable
to SRUs in this new medium. To address this, we invited AI image creators and SRUs to evaluate alt text prepared from various sources and write their own
alt text for AI images. Our mixed-methods analysis makes three contributions. First, we highlight creators’ perspectives on alt text, as creators are well-positioned
to write descriptions of their images. Second, we illustrate SRUs’ alt text needs particular to the emerging medium of AI images. Finally, we discuss the
promises and pitfalls of utilizing text prompts written as input for AI models in alt text generation, and areas where broader digital accessibility guidelines
could expand to account for AI images.
View details
AI’s Regimes of Representation: A Community-centered Study of Text-to-Image Models in South Asia
Rida Qadri
Proceedings of the 2023 ACM Conference on Fairness, Accountability, and Transparency, Association for Computing Machinery, 506–517
Preview abstract
This paper presents a community-centered study of cultural limitations of text-to-image (T2I) models in the South Asian context. We theorize these failures using scholarship on dominant media regimes of representations and locate them within participants’ reporting of their existing social marginalizations. We thus show how generative AI can reproduce an outsiders gaze for viewing South Asian cultures, shaped by global and regional power inequities. By centering communities as experts and soliciting their perspectives on T2I limitations, our study adds rich nuance into existing evaluative frameworks and deepens our understanding of the culturally-specific ways AI technologies can fail in non-Western and Global South settings. We distill lessons for responsible development of T2I models, recommending concrete pathways forward that can allow for recognition of structural inequalities.
View details
Characterizing Image Accessibility on Wikipedia across Languages
Elisa Kreiss
Tiziano Piccardi
Jesus Adolfo Hermosillo
Michael S. Bernstein
Christopher Potts
Wiki Workshop 2023 (to appear)
Preview abstract
We make a first attempt to characterize image accessibility on Wikipedia across languages, present new experimental results that can inform efforts to assess description quality, and offer some strategies to improve Wikipedia's image accessibility.
View details
Context Matters for Image Description Evaluation: Challenges for Referenceless Metrics
Elisa Kreiss
Shayan Hooshmand
Eric Zelikman
Christopher Potts
EMNLP 2022(2022) (to appear)
Preview abstract
Few images on the Web receive alt-text descriptions that would make them accessible to blind and low vision (BLV) users. Image-based NLG systems have progressed to the point where they can begin to address this persistent societal problem, but these systems will not be fully successful unless we evaluate them on metrics that guide their development correctly. Here, we argue against current referenceless metrics -- those that don't rely on human-generated ground-truth descriptions -- on the grounds that they do not align with the needs of BLV users. The fundamental shortcoming of these metrics is that they cannot take context into account, whereas contextual information is highly valued by BLV users. To substantiate these claims, we present a study with BLV participants who rated descriptions along a variety of dimensions. An in-depth analysis reveals that the lack of context-awareness makes current referenceless metrics inadequate for advancing image accessibility, requiring a rethinking of referenceless evaluation metrics for image-based NLG systems.
View details
Beyond Safety: Toward a Value-Sensitive Approach to the Design of AI Systems
Alex Fiannaca
NeurIPS 2022 Human-Centered AI Workshop(2022) (to appear)
Preview abstract
As modern, pre-trained ML models have proliferated in recent years , many researchers and practitioners have made significant efforts to prevent AI systems from causing harm. This focus on safety is critical, but a singular focus on safety can come at the exclusion of considering other important stakeholder values and the interactions between those values in the AI systems we build. In this position paper, we propose that the AI community should incorporate ideas from the Value-Sensitive Design framework from the Human-Computer Interaction community to ensure the needs and values of all stakeholders are reflected in the systems we build. We share observations and reflections from our experiences working on AI-supported accessibility technologies and with members of various disability communities to illustrate the tensions that sometimes arise between safety and other values.
View details