Publications
Our teams aspire to make discoveries that impact everyone, and core to our approach is sharing our research and tools to fuel progress in the field.
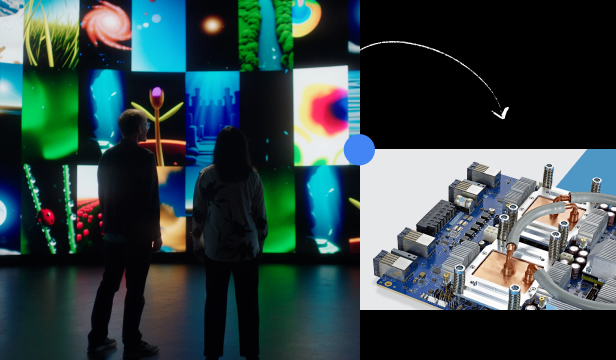
Our teams aspire to make discoveries that impact everyone, and core to our approach is sharing our research and tools to fuel progress in the field.
Sort By
1 - 15 of 15778 publications
Preview abstract
Electric vehicle (EV) adoption in long-distance logistics faces challenges like range anxiety and uneven distribution of charging stations. Two pivotal questions emerge: How can EVs be efficiently routed in a charging network considering range limits, charging speeds and prices And, can the existing charging infrastructure sustain the increasing demand for EVs in long-distance logistics? This paper addresses these questions by introducing a novel theoretical and computational framework to study the EV network flow problems. We present an EV network flow model that incorporates range restrictions and nonlinear charging rates, and identify conditions under which polynomial-time solutions can be obtained for optimal single EV routing, maximum flow, and minimum cost flow problems. We develop efficient computational methods for computing the optimal routing and flow vector using a novel graph augmentation technique. Our findings provide insights for optimizing EV routing in logistics, ensuring an efficient and sustainable future.
View details
Hierarchical Text Spotter for Joint Text Spotting and Layout Analysis
Winter Conference on Applications of Computer Vision 2024(2024) (to appear)
Preview abstract
We propose Hierarchical Text Spotter (HTS), the first method for the joint task of word-level text spotting and geometric layout analysis.
HTS can annotate text in images with a hierarchical representation of 4 levels: character, word, line, and paragraph.
The proposed HTS is characterized by two novel components:
(1) a Unified-Detector-Polygon (UDP) that produces Bezier Curve polygons of text lines and an affinity matrix for paragraph grouping between detected lines;
(2) a Line-to-Character-to-Word (L2C2W) recognizer that splits lines into characters and further merges them back into words.
HTS achieves state-of-the-art results on multiple word-level text spotting benchmark datasets as well as geometric layout analysis tasks.
Code will be released upon acceptance.
View details
Dynamic Inference of Likely Symbolic Tensor Shapes in Python Machine Learning Programs
Koushik Sen
International Conference on Software Engineering: Software Engineering in Practice (ICSE-SEIP)(2024) (to appear)
Preview abstract
In machine learning programs, it is often tedious to annotate the dimensions of shapes of various tensors that get created during execution. We present a dynamic likely tensor shape inference analysis that annotates the dimensions of shapes of tensor expressions with symbolic dimension values. Such annotations can be used for understanding the machine learning code written in popular frameworks, such as TensorFlow, PyTorch, JAX, and for finding bugs related to tensor shape mismatch.
View details
First Passage Percolation with Queried Hints
Kritkorn Karntikoon
Aaron Schild
Yiheng Shen
Ali Sinop
AISTATS(2024)
Preview abstract
Optimization problems are ubiquitous throughout the modern world. In many of these applications, the input is inherently noisy and it is expensive to probe all of the noise in the input before solving the relevant optimization problem. In this work, we study how much of that noise needs to be queried in order to obtain an approximately optimal solution to the relevant problem. We focus on the shortest path problem in graphs, where one may think of the noise as coming from real-time traffic. We consider the following model: start with a weighted base graph $G$ and multiply each edge weight by an independently chosen, uniformly random number in $[1,2]$ to obtain a random graph $G'$. This model is called \emph{first passage percolation}. Mathematicians have studied this model extensively when $G$ is a $d$-dimensional grid graph, but the behavior of shortest paths in this model is still poorly understood in general graphs. We make progress in this direction for a class of graphs that resembles real-world road networks. Specifically, we prove that if the geometric realization of $G$ has constant doubling dimension, then for a given $s-t$ pair, we only need to probe the weights on $((\log n) / \epsilon)^{O(1)}$ edges in $G'$ in order to obtain a $(1 + \epsilon)$-approximation to the $s-t$ distance in $G'$. We also demonstrate experimentally that this result is pessimistic -- one can even obtain a short path in $G'$ with a small number of probes to $G'$.
View details
SPHEAR: Spherical Head Registration for Complete Statistical 3D Modeling
Andrei Zanfir
Teodor Szente
Mihai Zanfir
International Conference on 3D Vision(2024)
Preview abstract
We present SPHEAR, an accurate, differentiable parametric statistical 3D human head model, enabled by a novel 3D registration method based on spherical embeddings. We shift the paradigm away from the classical Non-Rigid Registration methods, which operate under various surface priors, increasing reconstruction fidelity and minimizing required human intervention. Additionally, SPHEAR is a complete model that allows not only to sample diverse synthetic head shapes and facial expressions, but also gaze directions, high-resolution color textures, surface normal maps, and hair cuts represented in detail, as strands. SPHEAR can be used for automatic realistic visual data generation, semantic annotation, and general reconstruction tasks. Compared to state-of-the-art approaches, our components are fast and memory efficient, and experiments support the validity of our design choices and the accuracy of registration, reconstruction and generation techniques.
View details
Preview abstract
Artificial Intelligence (AI) holds the promise of transforming healthcare by improving patient outcomes, increasing accessibility and efficiency, and decreasing the cost of care. Realizing this vision of a healthier world for everyone everywhere requires partnerships and trust between healthcare systems, clinicians, payers, technology companies, pharmaceutical companies, and governments to drive innovations in machine learning and artificial intelligence to patients. Google is one example of a technology company that is partnering with healthcare systems, clinicians, and researchers to develop technology solutions that will directly improve the lives of patients. In this chapter we share landmark trials of the use of AI in healthcare. We also describe the application of our novel system of organizing information to unify data in electronic health records (EHRs) and bring an integrated view of patient records to clinicians. We discuss our consumer focused innovation in dermatology to help guide search journeys for personalized information about skin conditions. Finally, we share a perspective on how to embed ethics and a concern for all patients into the development of AI.
View details
Large Scale Self-Supervised Pretraining for Active Speaker Detection
Alice Chuang
Keith Johnson
Tony (Tuấn) Nguyễn
Wei Xia
Yunfan Ye
ICASSP 2024(2024) (to appear)
Preview abstract
In this work we investigate the impact of a large-scale self-supervised pretraining strategy for active speaker detection (ASD) on an unlabeled dataset consisting of over 125k hours of YouTube videos. When compared to a baseline trained from scratch on much smaller in-domain labeled datasets we show that with pretraining we not only have a more stable supervised training due to better audio-visual features used for initialization, but also improve the ASD mean average precision by 23\% on a challenging dataset collected with Google Nest Hub Max devices capturing real user interactions.
View details
Preview abstract
We propose a neural network model that can separate target speech sources from interfering sources at different angular regions using two microphones. The model is trained with simulated room impulse responses (RIRs) using omni-directional microphones without needing to collect real RIRs. By relying on specific angular regions and multiple room simulations, the model utilizes consistent time difference of arrival (TDOA) cues, or what we call delay contrast, to separate target and interference sources while remaining robust in various reverberation environments. We demonstrate the model is not only generalizable to a commercially available device with a slightly different microphone geometry, but also outperforms our previous work which uses one additional microphone on the same device. The model runs in real-time on-device and is suitable for low-latency streaming applications such as telephony and video conferencing.
View details
Preview abstract
Algorithms for the computation of alternative routes in road networks power many geographic navigation systems. A good set of alternative routes offers meaningful options to the user of the system and can support applications such as routing that is robust to failures (e.g., road closures, extreme traffic congestion, etc.) and routing with diverse preferences and objective functions. Algorithmic techniques for alternative route computation include the penalty method, via-node type algorithms (which deploy bidirectional search and finding plateaus), and, more recently, electrical-circuit based algorithms. In this work we focus on the practically important family of via-node type algorithms and we aim to produce high quality alternative routes for road netowrks. We study alternative route computation in the presence of a fast routing infrastructure that relies on hierarchical routing (namely, CRP). We propose new approaches that rely on deep learning methods. Our training methodology utilizes the hierarchical partition of the graph and builds models to predict which boundary road segments in the partition should be crossed by the alternative routes. We describe our methods in detail and evaluate them against the previously studied architectures, as well as against a stronger baseline that we define in this work, showing improvements in quality in the road networks of Seattle, Paris, and Bangalore.
View details
Preview abstract
Predictive uncertainty-a model's self awareness regarding its accuracy on an input-is key for both building robust models via training interventions and for test-time applications such as selective classification. We propose a novel instance-conditioned reweighting approach that captures predictive uncertainty using an auxiliary network and unifies these train- and test-time applications. The auxiliary network is trained using a meta-objective in a bilevel optimization framework. A key contribution of our proposal is the meta-objective of minimizing the dropout variance, an approximation of Bayesian Predictive uncertainty. We show in controlled experiments that we effectively capture the diverse specific notions of uncertainty through this meta-objective, while previous approaches only capture certain aspects. These results translate to significant gains in real-world settings-selective classification, label noise, domain adaptation, calibration-and across datasets-Imagenet, Cifar100, diabetic retinopathy, Camelyon, WILDs, Imagenet-C,-A,-R, Clothing1M, etc. For Diabetic Retinopathy, we see upto 3.4%/3.3% accuracy and AUC gains over SOTA in selective classification. We also improve upon large-scale pretrained models such as PLEX.
View details
Delphic Offline Reinforcement Learning under Nonidentifiable Hidden Confounding
Alizée Pace
Hugo Yèche
Bernhard Schölkopf
Gunnar Rätsch
The Twelfth International Conference on Learning Representations(2024)
Preview abstract
A prominent challenge of offline reinforcement learning (RL) is the issue of hidden confounding. There, unobserved variables may influence both the actions taken by the agent and the outcomes observed in the data. Hidden confounding can compromise the validity of any causal conclusion drawn from the data and presents a major obstacle to effective offline RL. In this paper, we tackle the problem of hidden confounding in the nonidentifiable setting. We propose a definition of uncertainty due to confounding bias, termed delphic uncertainty, which uses variation over compatible world models, and differentiate it from the well known epistemic and aleatoric uncertainties. We derive a practical method for estimating the three types of uncertainties, and construct a pessimistic offline RL algorithm to account for them. Our method does not assume identifiability of the unobserved confounders, and attempts to reduce the amount of confounding bias. We demonstrate through extensive experiments and ablations the efficacy of our approach on a sepsis management benchmark, as well as real electronic health records. Our results suggest that nonidentifiable confounding bias can be addressed in practice to improve offline RL solutions.
View details
Preview abstract
Graph Neural Networks (GNNs) are popular models for machine learning on graphs that typically follow the message-passing paradigm, whereby the feature of a node is updated recursively upon aggregating information over its neighbors. While exchanging messages over the input graph endows GNNs with a strong inductive bias, it can also make GNNs susceptible to \emph{over-squashing}, thereby preventing them from capturing long-range interactions in the given graph. To rectify this issue, {\em graph rewiring} techniques have been proposed as a means of improving information flow by altering the graph connectivity. In this work, we identify three desiderata for graph-rewiring: (i) reduce over-squashing, (ii) respect the locality of the graph, and (iii) preserve the sparsity of the graph. We highlight fundamental trade-offs that occur between {\em spatial} and {\em spectral} rewiring techniques; while the former often satisfy (i) and (ii) but not (iii), the latter generally satisfy (i) and (iii) at the expense of (ii). We propose a novel rewiring framework that satisfies all of (i)--(iii) through a locality-aware sequence of rewiring operations. We then discuss a specific instance of such rewiring framework and validate its effectiveness on several real-world benchmarks, showing that it either matches or significantly outperforms existing rewiring approaches.
View details
Preview abstract
We present PhoMoH, a neural network methodology to construct generative models of photo-realistic 3D geometry and appearance of human heads including hair, beards, an oral cavity, and clothing. In contrast to prior work, PhoMoH models the human head using neural fields, thus supporting complex topology. Instead of learning a head model from scratch, we propose to augment an existing expressive head model with new features. Concretely, we learn a highly detailed geometry network layered on top of a mid-resolution head model together with a detailed, local geometry-aware, and disentangled color field. Our proposed architecture allows us to learn photo-realistic human head models from relatively little data. The learned generative geometry and appearance networks can be sampled individually and enable the creation of diverse and realistic human heads. Extensive experiments validate our method qualitatively and across different metrics.
View details
Quantum Computation of Stopping power for Inertial Fusion Target Design
Dominic Berry
Alina Kononov
Alec White
Joonho Lee
Andrew Baczewski
Proceedings of the National Academy of Sciences, 121(2024), e2317772121
Preview abstract
Stopping power is the rate at which a material absorbs the kinetic energy of a charged particle passing through it - one of many properties needed over a wide range of thermodynamic conditions in modeling inertial fusion implosions. First-principles stopping calculations are classically challenging because they involve the dynamics of large electronic systems far from equilibrium, with accuracies that are particularly difficult to constrain and assess in the warm-dense conditions preceding ignition. Here, we describe a protocol for using a fault-tolerant quantum computer to calculate stopping power from a first-quantized representation of the electrons and projectile. Our approach builds upon the electronic structure block encodings of Su et al. [PRX Quantum 2, 040332 2021], adapting and optimizing those algorithms to estimate observables of interest from the non-Born-Oppenheimer dynamics of multiple particle species at finite temperature. We also work out the constant factors associated with a novel implementation of a high order Trotter approach to simulating a grid representation of these systems. Ultimately, we report logical qubit requirements and leading-order Toffoli costs for computing the stopping power of various projectile/target combinations relevant to interpreting and designing inertial fusion experiments. We estimate that scientifically interesting and classically intractable stopping power calculations can be quantum simulated with
roughly the same number of logical qubits and about one hundred times more Toffoli gates than is required for state-of-the-art quantum simulations of industrially relevant molecules such as FeMoCo or P450.
View details
Multimodal Modeling for Spoken Language Identification
Shikhar Bharadwaj
Sriram (Sri) Ganapathy
Sid Dalmia
Wei Han
Yu Zhang
Proceedings of 2024 IEEE International Conference on Acoustics, Speech and Signal Processing (ICASSP 2024)(2024)
Preview abstract
Spoken language identification refers to the task of automatically predicting the spoken language in a given utterance. Conventionally, it is modeled as a speech-based language identification task. Prior techniques have been constrained to a single modality; however in the case of video data there is a wealth of other metadata that may be beneficial for this task. In this work, we propose MuSeLI, a Multimodal Spoken Language Identification method, which delves into the use of various metadata sources to enhance language identification. Our study reveals that metadata such as video title, description and geographic location provide substantial information to identify the spoken language of the multimedia recording. We conduct experiments using two diverse public datasets of YouTube videos, and obtain state-of-the-art results on the language identification task. We additionally conduct an ablation study that describes the distinct contribution of each modality for language recognition.
View details