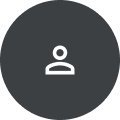
Cristian Sminchisescu
Cristian Sminchisescu is a Research Scientist and Engineering Manager at Google, and a Professor at Lund University. He has obtained a doctorate in computer science and applied mathematics with focus on imaging, vision and robotics at INRIA, under an Eiffel excellence fellowship of the French Ministry of Foreign Affairs, and has done postdoctoral research in the Artificial intelligence Laboratory at the University of Toronto. He has held a Professor equivalent title at the Romanian Academy and a Professor rank, status appointment at Toronto, and has advised research at both institutions. During 2004-07, he was a faculty member at the Toyota Technological Institute at the University of Chicago, and later on the Faculty of the Institute for Numerical Simulation in the Mathematics Department at Bonn University. Over time, his work has been funded by the US National Science Foundation, the Romanian Science Foundation, the German Science Foundation, the Swedish Science Foundation, the European Commission under a Marie Curie Excellence Grant, and the European Research Council under an ERC Consolidator Grant. Cristian Sminchisescu's research interests are in the area of computer vision (3d human sensing, reconstruction and recognition) and machine learning (optimization and sampling algorithms, kernel methods and deep learning). The visual recognition methodology developed in his group was a winner of the PASCAL VOC object segmentation and labeling challenge during 2009-12, as well as the Reconstruction Meets Recognition Challenge (RMRC) 2013-14. His work on deep learning of graph matching has received the best paper award honorable mention at CVPR 2018. Cristian Sminchisescu regularly serves as an Area Chair for computer vision and machine learning conferences (CVPR, ECCV, ICCV, AAAI, neurIPS). He has been an Associate Editor of IEEE Transactions for Pattern Analysis and Machine Intelligence (PAMI) and the International Journal of Computer Vision (IJCV), was a Program Chair for ECCV 2018, and is General Chair for CVPR 2025.
Authored Publications
Google Publications
Other Publications
Sort By
DiffHuman: Probabilistic Photorealistic 3D Reconstruction of Humans
Akash Sengupta
Enric Corona
Andrei Zanfir
Proceedings of the IEEE/CVF Conference on Computer Vision and Pattern Recognition (CVPR) (2024)
Preview abstract
We present DiffHuman, a probabilistic method for photorealistic 3D human reconstruction from a single RGB image. Despite the ill-posed nature of this problem, most methods are deterministic and output a single solution, often resulting in a lack of geometric detail and blurriness in unseen or uncertain regions. In contrast, DiffHuman predicts a distribution over 3D reconstructions conditioned on an image, which allows us to sample multiple detailed 3D avatars that are consistent with the input image. DiffHuman is implemented as a conditional diffusion model that denoises partial observations of an underlying pixel-aligned 3D representation. In testing, we can sample a 3D shape by iteratively denoising renderings of the predicted intermediate representation. Further, we introduce an additional generator neural network that approximates rendering with considerably reduced runtime (55x speed up), resulting in a novel dual-branch diffusion framework. We evaluate the effectiveness of our approach through various experiments. Our method can produce diverse, more detailed reconstructions for the parts of the person not observed in the image, and has competitive performance for the surface reconstruction of visible parts.
View details
Preview abstract
We present PhoMoH, a neural network methodology to construct generative models of photo-realistic 3D geometry and appearance of human heads including hair, beards, an oral cavity, and clothing. In contrast to prior work, PhoMoH models the human head using neural fields, thus supporting complex topology. Instead of learning a head model from scratch, we propose to augment an existing expressive head model with new features. Concretely, we learn a highly detailed geometry network layered on top of a mid-resolution head model together with a detailed, local geometry-aware, and disentangled color field. Our proposed architecture allows us to learn photo-realistic human head models from relatively little data. The learned generative geometry and appearance networks can be sampled individually and enable the creation of diverse and realistic human heads. Extensive experiments validate our method qualitatively and across different metrics.
View details
SPHEAR: Spherical Head Registration for Complete Statistical 3D Modeling
Andrei Zanfir
Teodor Szente
Mihai Zanfir
International Conference on 3D Vision (2024)
Preview abstract
We present SPHEAR, an accurate, differentiable parametric statistical 3D human head model, enabled by a novel 3D registration method based on spherical embeddings. We shift the paradigm away from the classical Non-Rigid Registration methods, which operate under various surface priors, increasing reconstruction fidelity and minimizing required human intervention. Additionally, SPHEAR is a complete model that allows not only to sample diverse synthetic head shapes and facial expressions, but also gaze directions, high-resolution color textures, surface normal maps, and hair cuts represented in detail, as strands. SPHEAR can be used for automatic realistic visual data generation, semantic annotation, and general reconstruction tasks. Compared to state-of-the-art approaches, our components are fast and memory efficient, and experiments support the validity of our design choices and the accuracy of registration, reconstruction and generation techniques.
View details
Structured 3D Features for Reconstructing Controllable Avatars
Enric Corona
Mihai Zanfir
Andrei Zanfir
Proceedings of the IEEE/CVF Conference on Computer Vision and Pattern Recognition (CVPR) (2023)
Preview abstract
We introduce Structured 3D Features, a model based on a novel implicit 3D representation that pools pixel-aligned image features onto dense 3D points sampled from a parametric, statistical human mesh surface. The 3D points have associated semantics and can move freely in 3D space. This allows for optimal coverage of the person of interest, beyond just the body shape, which in turn, additionally helps modeling accessories, hair, and loose clothing. Owing to this, we present a complete 3D transformer-based attention framework which, given a single image of a person in an unconstrained pose, generates an animatable 3D reconstruction with albedo and illumination decomposition, as a result of a single end-to-end model, trained semi-supervised, and with no additional postprocessing. We show that our S3F
model surpasses the previous state-of-the-art on various tasks, including monocular 3D reconstruction, as well as albedo & shading estimation. Moreover, we show that the proposed methodology allows novel view synthesis, relighting, and re-posing the reconstruction, and can naturally be extended to handle multiple input images (e.g. different views of a person, or the same view, in different poses, in video). Finally, we demonstrate the editing capabilities of our model for 3D virtual try-on applications.
View details
DreamHuman: Animatable 3D Avatars from Text
Andrei Zanfir
Mihai Fieraru
Advances in Neural Information Processing Systems (2023)
Preview abstract
We present DreamHuman, a method to generate realistic animatable 3D human avatar models solely from textual descriptions. Recent text-to-3D methods have made considerable strides in generation, but are still lacking in important aspects. Control and often spatial resolution remain limited, existing methods produce fixed rather than animated 3D human models, and anthropometric consistency for complex structures like people remains a challenge. DreamHuman connects large text-to-image synthesis models, neural radiance fields, and statistical human body models in a novel modeling and optimization framework. This makes it possible to generate dynamic 3D human avatars with high-quality textures and learned, instance-specific, surface deformations. We demonstrate that our method is capable to generate a wide variety of animatable, realistic 3D human models from text. Our 3D models have diverse appearance, clothing, skin tones and body shapes, and significantly outperform both generic text-to-3D approaches and previous text-based 3D avatar generators in visual fidelity.
View details
Photorealistic Monocular 3D Reconstruction of Humans Wearing Clothing
Mihai Zanfir
Proceedings of the IEEE/CVF Conference on Computer Vision and Pattern Recognition (CVPR), IEEE (2022)
Preview abstract
We present PHORHUM, a novel, end-to-end trainable, deep neural network methodology for photorealistic 3D human reconstruction given just a monocular RGB image. Our pixel-aligned method estimates detailed 3D geometry and, for the first time, the unshaded surface color together with the scene illumination. Observing that 3D supervision alone is not sufficient for high fidelity color reconstruction, we introduce patch-based rendering losses that enable reliable color reconstruction on visible parts of the human, and detailed and plausible color estimation for the non-visible parts. Moreover, our method specifically addresses methodological and practical limitations of prior work in terms of representing geometry, albedo, and illumination effects, in an end-to-end model where factors can be effectively disentangled. In extensive experiments, we demonstrate the versatility and robustness of our approach. Our state-of-the-art results validate the method qualitatively and for different metrics, for both geometric and color reconstruction.
View details
Calibration of Neural Networks using Splines
Kartik Gupta
Amir Rahimi
Thalaiyasingam Ajanthan
Richard Ian Hartley
International Conference on Learning Representations (ICLR) (2021)
Preview abstract
Calibrating neural networks is of utmost importance when employing them in safety-critical applications where the downstream decision making depends on the predicted probabilities. Measuring calibration error amounts to comparing two empirical distributions. In this work, we introduce a binning-free calibration measure inspired by the classical Kolmogorov-Smirnov (KS) statistical test in which the main idea is to compare the respective cumulative probability distributions. From this, by approximating the empirical cumulative distribution using a differentiable function via splines, we obtain a recalibration function, which maps the network outputs to actual (calibrated) class assignment probabilities. The spline-fitting is performed using a held-out calibration set and the obtained recalibration function is evaluated on an unseen test set. We tested our method against existing calibration approaches on various image classification datasets and our spline-based recalibration approach consistently outperforms existing methods on KS error as well as other commonly used calibration measures.
View details
H-NeRF: Neural Radiance Fields for Rendering and Temporal Reconstruction of Humans in Motion
Hongyi Xu
Advances in Neural Information Processing Systems (NeurIPS) (2021)
Preview abstract
We present neural radiance fields for rendering and temporal (4D) reconstruction of humans in motion (H-NeRF), as captured by a sparse set of cameras or even from a monocular video. Our approach combines ideas from neural scene representation, novel-view synthesis, and implicit statistical geometric human representations, coupled using novel loss functions. Instead of learning a radiance field with a uniform occupancy prior, we constrain it by a structured implicit human body model, represented using signed distance functions. This allows us to robustly fuse information from sparse views and generalize well beyond the poses or views observed in training. Moreover, we apply geometric constraints to co-learn the structure of the observed subject -- including both body and clothing -- and to regularize the radiance field to geometrically plausible solutions. Extensive experiments on multiple datasets demonstrate the robustness and the accuracy of our approach, its generalization capabilities significantly outside a small training set of poses and views, and statistical extrapolation beyond the observed shape.
View details
THUNDR: Transformer-based 3D HUmaN Reconstruction with Markers
Mihai Zanfir
Andrei Zanfir
Proceedings of the IEEE/CVF International Conference on Computer Vision (2021)
Preview abstract
We present THUNDR, a transformer-based deep neural network methodology to reconstruct the 3D pose and shape of people, given monocular RGB images. Key to our methodology is an intermediate 3D marker representation, where we aim to combine the predictive power of model-free output architectures and the regularizing, anthropometrically-preserving properties of a statistical human surface models like GHUM—a recently introduced, expressive full body statistical 3d human model, trained end-to-end. Our novel transformer-based prediction pipeline can focus on image regions relevant to the task, supports self-supervised regimes, and ensures that solutions are consistent with human anthropometry. We show state-of-the-art results on Human3.6M and 3DPW, for both the fully-supervised and the self-supervised models, for the task of inferring 3D human shape, joint positions, and global translation. Moreover, we observe very solid 3d reconstruction performance for difficult human poses collected in the wild. Models will be made available for research.
View details
imGHUM: Implicit Generative Models of 3D Human Shape and Articulated Pose
Hongyi Xu
Proceedings of the IEEE/CVF International Conference on Computer Vision, IEEE (2021), pp. 5461-5470
Preview abstract
We present imGHUM, the first holistic generative model of 3D human shape and articulated pose, represented as a signed distance function. In contrast to prior work, we model the full human body implicitly as a function zero-level-set and without the use of an explicit template mesh. We propose a novel network architecture and a learning paradigm, which make it possible to learn a detailed implicit generative model of human pose, shape, and semantics, on par with state-of-the-art mesh-based models. Our model features desired detail for human models, such as articulated pose including hand motion and facial expressions, a broad spectrum of shape variations, and can be queried at arbitrary resolutions and spatial locations. Additionally, our model has attached spatial semantics making it straightforward to establish correspondences between different shape instances, thus enabling applications that are difficult to tackle using classical implicit representations. In extensive experiments, we demonstrate the model accuracy and its applicability to current research problems.
View details
Neural Descent for Visual 3D Human Pose and Shape
Andrei Zanfir
Mihai Zanfir
Proceedings of the IEEE/CVF Conference on Computer Vision and Pattern Recognition (CVPR) (2021), pp. 14484-14493
Preview abstract
We present a deep neural network methodology to reconstruct the 3d pose and shape of people, given image or video inputs. We rely on a recently introduced, expressive full body statistical 3d human model, GHUM, with facial expression and hand detail and aim to learn to reconstruct the model pose and shape states in a self-supervised regime. Central to our methodology, is a learning to learn approach, referred to as HUman Neural Descent (HUND) that avoids both second-order differentiation when training the model parameters, and expensive state gradient descent in order to accurately minimize a semantic differentiable rendering loss at test time. Instead, we rely on novel recurrent stages to update the pose and shape parameters such that not only losses are minimized effectively but the process is regularized in order to ensure progress.
The newly introduced architecture is tested extensively, and achieves state-of-the-art results on datasets like H3.6M and 3DPW, as well as in complex imagery collected in-the-wild.
View details
GHUM & GHUML: Generative 3D Human Shape and Articulated Pose Models
Hongyi Xu
Andrei Zanfir
IEEE/CVF Conference on Computer Vision and Pattern Recognition (Oral) (2020), pp. 6184-6193
Preview abstract
We present a statistical, articulated 3D human shape modeling pipeline, within a fully trainable, modular, deep learning framework. Given high-resolution complete 3D body scans of humans, captured in various poses, together with additional closeups of their head and facial expressions, as well as hand articulation, and given initial, artist designed, gender neutral rigged quad-meshes, we train all model parameters including non-linear shape spaces based on variational auto-encoders, pose-space deformation correctives, skeleton joint center predictors, and blend skinning functions, in a single consistent learning loop. The models are simultaneously trained with all the 3d dynamic scan data (over60,000diverse human configurations in our new dataset) in order to capture correlations and en-sure consistency of various components. Models support facial expression analysis, as well as body (with detailed hand) shape and pose estimation. We provide fully train-able generic human models of different resolutions – the moderate-resolution GHUM consisting of 10,168 vertices and the low-resolution GHUML(ite) of 3,194 vertices –, run comparisons between them, analyze the impact of different components and illustrate their reconstruction from image data. The models are available for research.
View details
Range Conditioned Dilated Convolutions for Scale Invariant 3D Object Detection
Pei Sun
Drago Anguelov
Conference on Robot Learning (2020)
Preview abstract
This paper presents a novel 3D object detection framework that processes LiDAR data directly on a representation of the sensor's native range images. When operating in the range image view, one faces learning challenges, including occlusion and considerable scale variation, limiting the obtainable accuracy. To address these challenges, a range-conditioned dilated block (RCD) is proposed to dynamically adjust a continuous dilation rate as a function of the measured range, achieving scale invariance. Furthermore, soft range gating helps mitigate the effect of occlusion. An end-to-end trained box-refinement network brings additional performance improvements in occluded areas, and produces more accurate bounding box predictions. On the Waymo Open Dataset, currently the largest and most diverse publicly released autonomous driving dataset, our improved range-based detector outperforms state of the art at long range detection. Our framework is superior to prior multiview, voxel-based methods over all ranges, setting a new baseline for range-based 3D detection on this large scale public dataset.
View details
Preview abstract
We present a self-supervised framework, Consistency Guided Scene Flow Estimation (CGSF), to jointly estimate 3D scene structure and motion from stereo videos. The model takes two temporal stereo pairs as input, and predicts disparity and scene flow expressed as optical flow + disparity change. The model self-adapts at test time by iteratively refining its predictions. The refinement process is guided by a consistency loss, which combines stereo and temporal photo-consistency with a new geometric term that couples the disparity and 3D motion. To handle the noise in the consistency loss, we further propose a learned, output refinement network, which takes the initial predictions, the loss, and the gradient as input, and efficiently predicts a correlated output update. We perform extensive experimental validation on benchmark datasets and daily scenes captured by a stereo camera. We demonstrate the proposed model can reliably predict disparity and scene flow in many challenging scenarios, and achieves better generalization than the state-of-the-arts.
View details
Weakly Supervised 3D Human Pose and Shape Reconstruction with Normalizing Flows
Andrei Zanfir
Hongyi Xu
European Conference on Computer Vision (ECCV) (2020), pp. 465-481
Preview abstract
Monocular 3D human pose and shape estimation is challenging due to the many degrees of freedom of the human body and thedifficulty to acquire training data for large-scale supervised learning incomplex visual scenes. In this paper we present practical semi-supervisedand self-supervised models that support training and good generalizationin real-world images and video. Our formulation is based on kinematiclatent normalizing flow representations and dynamics, as well as differ-entiable, semantic body part alignment loss functions that support self-supervised learning. In extensive experiments using 3D motion capturedatasets like CMU, Human3.6M, 3DPW, or AMASS, as well as imagerepositories like COCO, we show that the proposed methods outperformthe state of the art, supporting the practical construction of an accuratefamily of models based on large-scale training with diverse and incom-pletely labeled image and video data.
View details
Preview abstract
We present GLNet, a self-supervised framework for learning depth, optical flow, camera pose and intrinsic parameters from monocular video – addressing the difficulty of acquiring realistic ground-truth for such processes under a variety of conditions where we would like them to operate. We propose three contributions for self-supervised systems: 1) we design new loss functions that capture multiple geometric constraints (e.g. epipolar geometry) as well as adaptive photometric costs that support multiple moving objects, rigid and non-rigid, 2) we extend the model such that it predicts camera intrinsics, making it applicable to uncalibrated images or video, and 3) we propose several online finetuning strategies that rely on the symmetry of our self-supervised loss in both training and testing, in particular optimizing both parameters and/or the output of different tasks and leveraging their mutual interactions. The idea of jointly optimizing the system output, under all geometric and photometric constraints can be viewed as a dense generalization of classical bundle adjustment. We demonstrate the effectiveness of our method on KITTI and Cityscapes, where we outperform previous self-supervised approaches. We also show good generalization for transfer learning.
View details
Preview abstract
We introduce new, fine-grained action and emotion
recognition tasks defined on non-staged videos, recorded
during robot-assisted therapy sessions of children with
autism. The tasks present several challenges: a large
dataset with long videos, a large number of highly variable
actions, children that are only partially visible, have
different ages and may show unpredictable behaviour, as
well as non-standard camera viewpoints. We investigate
how state-of-the-art 3d human pose reconstruction methods
perform on the newly introduced tasks and propose extensions
to adapt them to deal with these challenges. We also
analyze multiple approaches in action and emotion recognition
from 3d human pose data, establish several baselines,
and discuss results and their implications in the context of
child-robot interaction.
View details
Preview abstract
We propose an automatic person-to-person appearance
transfer model based on explicit parametric 3d human representations
and learned, constrained deep translation network
architectures for photographic image synthesis. Given
a single source image and a single target image, each
corresponding to different human subjects, wearing different
clothing and in different poses, our goal is to photorealistically
transfer the appearance from the source image
onto the target image while preserving the target shape
and clothing segmentation layout. Our solution to this new
problem is formulated in terms of a computational pipeline
that combines (1) 3d human pose and body shape estimation
from monocular images, (2) identifying 3d surface colors elements
(mesh triangles) visible in both images, that can be
transferred directly using barycentric procedures, and (3)
predicting surface appearance missing in the first image but
visible in the second one using deep learning-based image
synthesis techniques. Our model achieves promising results
as supported by a perceptual user study where the participants
rated around 65% of our results as good, very good
or perfect, as well in automated tests (Inception scores and
a Faster-RCNN human detector responding very similarly
to real and model generated images). We further show how
the proposed architecture can be profiled to automatically
generate images of a person dressed with different clothing
transferred from a person in another image, opening
paths for applications in entertainment and photo-editing
(e.g. embodying and posing as friends or famous actors),
the fashion industry, or affordable online shopping of clothing.
View details
Preview abstract
Semantic video segmentation is challenging due to the
sheer amount of data that needs to be processed and labeled
in order to construct accurate models. In this paper
we present a deep, end-to-end trainable methodology
for video segmentation that is capable of leveraging the information
present in unlabeled data, besides sparsely labeled
frames, in order to improve semantic estimates. Our
model combines a convolutional architecture and a spatiotemporal
transformer recurrent layer that is able to temporally
propagate labeling information by means of optical
flow, adaptively gated based on its locally estimated uncertainty.
The flow, the recognition and the gated temporal
propagation modules can be trained jointly, end-to-end.
The temporal, gated recurrent flow propagation component
of our model can be plugged into any static semantic segmentation
architecture and turn it into a weakly supervised
video processing one. Our experiments in the challenging
CityScapes and Camvid datasets, and for multiple deep architectures,
indicate that the resulting model can leverage
unlabeled temporal frames, next to a labeled one, in order
to improve both the video segmentation accuracy and the
consistency of its temporal labeling, at no additional annotation
cost and with little extra computation.
View details
Preview abstract
Human sensing has greatly benefited from recent advances in deep learning, parametric human modeling, and large scale 2d and 3d datasets. However, existing 3d models make strong assumptions about the scene, considering either a single person per image, full views of the person, a simple background or many cameras. In this paper, we leverage state-of-the-art deep multi-task neural networks and parametric human and scene modeling, towards a fully automatic monocular visual sensing system for multiple interacting people, which (i) infers the 2d and 3d pose and shape of multiple people from a single image, relying on detailed semantic representations at both model and image level, to guide a combined optimization with feedforward and feedback components, (ii) automatically integrates scene constraints including ground plane support and simultaneous volume occupancy by multiple people, and (iii) extends the single image model to video by optimally solving the temporal person assignment problem and imposing coherent temporal pose and motion reconstructions while preserving image alignment fidelity. We perform experiments on both single and multi-person datasets, and systematically evaluate each component of the model, showing improved performance and extensive multiple human sensing capability. We also apply our method to images with multiple people, severe occlusions and diverse backgrounds captured in challenging natural scenes, and obtain results of good perceptual quality.
View details
Preview abstract
We propose drl-RPN, a deep reinforcement learningbased
visual recognition model consisting of a sequential
region proposal network (RPN) and an object detector. In
contrast to typical RPNs, where candidate object regions
(RoIs) are selected greedily via class-agnostic NMS, drlRPN
optimizes an objective closer to the final detection
task. This is achieved by replacing the greedy RoI selection
process with a sequential attention mechanism which is
trained via deep reinforcement learning (RL). Our model is
capable of accumulating class-specific evidence over time,
potentially affecting subsequent proposals and classification
scores, and we show that such context integration significantly
boosts detection accuracy. Moreover, drl-RPN
automatically decides when to stop the search process and
has the benefit of being able to jointly learn the parameters
of the policy and the detector, both represented as deep networks.
Our model can further learn to search over a wide
range of exploration-accuracy trade-offs making it possible
to specify or adapt the exploration extent at test time.
The resulting search trajectories are image- and categorydependent,
yet rely only on a single policy over all object
categories. Results on the MS COCO and PASCAL
VOC challenges show that our approach outperforms established,
typical state-of-the-art object detection pipelines.
View details
Preview abstract
The problem of graph matching under node and pairwise constraints is fundamental in areas as diverse as combinatorial optimization, machine learning or computer vision, where representing both the relations between nodes and their neighborhood structure is essential. We present an end-to-end model that makes it possible to learn all parameters of the graph matching process, including the unary and pairwise node neighborhoods, represented as deep feature extraction hierarchies. The challenge is in the formulation of the different matrix computation layers of the model in a way that enables the consistent, efficient propagation of gradients in the complete pipeline from the loss function, through the combinatorial optimization layer solving the matching problem, and the feature extraction hierarchy. Our computer vision experiments and ablation studies on challenging datasets like PASCAL VOC keypoints, Sintel and CUB show that matching models refined end-to-end are superior to counterparts based on feature hierarchies trained for other problems.
View details
Efficient Closed-Form Solution to Generalized Boundary Detection
Marius Leordeanu
Proceedings of European Conference on Computer Vision (ECCV'12) (2012)
Preview abstract
Boundary detection is essential for a variety of computer vision tasks such as segmentation and recognition. We propose a unified formulation for boundary detection, with closed-form solution, which is applicable to the localization of different types of boundaries, such as intensity edges and occlusion boundaries from video and RGB-D cameras. Our algorithm simultaneously combines low- and mid-level image representations, in a single eigenvalue problem, and we solve over an infinite set of putative boundary orientations. Moreover, our method achieves state of the art results at a significantly lower computational cost than current methods. We also propose a novel method for soft-segmentation that can be used in conjunction with our boundary detection algorithm and improve its accuracy at a negligible extra computational cost.
View details
No Results Found