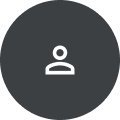
George Sung
Research Areas
Authored Publications
Google Publications
Other Publications
Sort By
StreamVC: Real-Time Low-Latency Voice Conversion
Jiuqiang Tang
Xing Li
ICASSP 2024 (2024)
Preview abstract
We present StreamVC, a streaming voice conversion solution that preserves the content and prosody of any source speech while matching the voice timbre from any target speech. Unlike previous approaches, StreamVC produces the resulting waveform at low latency from the input signal even on a mobile platform, making it applicable to real-time communication scenarios like calls and video conferencing, and addressing use cases such as voice anonymization in these scenarios. Our design leverages the architecture and training strategy of the SoundStream neural audio codec for lightweight high-quality speech synthesis. We demonstrate the feasibility of learning soft speech units causally, as well as the effectiveness of supplying whitened fundamental frequency information to improve pitch stability without leaking the source timbre information.
View details
Preview abstract
We propose a neural network model that can separate target speech sources from interfering sources at different angular regions using two microphones. The model is trained with simulated room impulse responses (RIRs) using omni-directional microphones without needing to collect real RIRs. By relying on specific angular regions and multiple room simulations, the model utilizes consistent time difference of arrival (TDOA) cues, or what we call delay contrast, to separate target and interference sources while remaining robust in various reverberation environments. We demonstrate the model is not only generalizable to a commercially available device with a slightly different microphone geometry, but also outperforms our previous work which uses one additional microphone on the same device. The model runs in real-time on-device and is suitable for low-latency streaming applications such as telephony and video conferencing.
View details
Guided Speech Enhancement Network
Jamie Lin
ICASSP 2023 - 2023 IEEE International Conference on Acoustics, Speech and Signal Processing (ICASSP)
Preview abstract
High quality speech capture has been widely studied for both voice communication and human computer interface reasons. To improve the capture performance, we can often find multi-microphone speech enhancement techniques deployed on various devices. Multi-microphone speech enhancement problem is often decomposed into two decoupled steps: a beamformer that provides spatial filtering and a single-channel speech enhancement model that cleans up the beamformer output. In this work, we propose a speech enhancement solution that takes both the raw microphone and beamformer outputs as the input for an ML model. We devise a simple yet effective training scheme that allows the model to learn from the cues of the beamformer by contrasting the two inputs and greatly boost its capability in spatial rejection, while conducting the general tasks of denoising and dereverberation. The proposed solution takes advantage of classical spatial filtering algorithms instead of competing with them. By design, the beamformer module then could be selected separately and does not require a large amount of data to be optimized for a given form factor, and the network model can be considered as a standalone module which is highly transferable independently from the microphone array. We name the ML module in our solution as GSENet, short for Guided Speech Enhancement Network. We demonstrate its effectiveness on real world data collected on multi-microphone devices in terms of the suppression of noise and interfering speech.
View details
Efficient Heterogeneous Video Segmentation at the Edge
Jamie Lin
Siargey Pisarchyk
David Cong Tian
Sixth Workshop on Computer Vision for AR/VR (CV4ARVR) (2022)
Preview abstract
We introduce an efficient video segmentation system for resource-limited edge devices leveraging heterogeneous compute. Specifically, we design network models by searching across multiple dimensions of specifications for the neural architectures and operations on top of already light-weight backbones, targeting commercially available edge inference engines. We further analyze and optimize the heterogeneous data flows in our systems across the CPU, the GPU and the NPU. Our approach has empirically factored well into our real-time AR system, enabling remarkably higher accuracy with quadrupled effective resolutions, yet at much shorter end-to-end latency, much higher frame rate, and even lower power consumption on edge platforms.
View details
On-device Real-time Hand Gesture Recognition
Chuo-Ling Chang
Esha Uboweja
Kanstantsin Sokal
Valentin Bazarevsky
ICCV Workshop on Computer Vision for Augmented and Virtual Reality, Montreal, Canada, 2021 (2021)
Preview abstract
We present an on-device real-time hand gesture recogni-tion (HGR) system, which detects a set of predefined staticgestures from a single RGB camera. The system consists oftwo parts: a hand skeleton tracker and a gesture classifier.We improve and extend MediaPipe Hands [12] for the handtracker. We experiment with two different gesture classifiers,one heuristics based and one neural network (NN) based.
View details
MediaPipe Hands: On-device Real-time Hand Tracking
Andrey Vakunov
Chuo-Ling Chang
Fan Zhang
Valentin Bazarevsky
CV4ARVR 2020 (2020)
Preview abstract
We present a real-time on-device hand tracking pipeline that predicts hand skeleton from only single camera input for AR/VR applications. The pipeline consists of two models: 1) a palm detector, 2) a hand landmark prediction. It's implemented via MediaPipe which is a cross-platform ML pipeline. The proposed architecture demonstrates realtime inference speed on mobile GPUs and a high prediction quality. MediaPipe Hands is open-sourced at https://github.com/google/mediapipe.
View details
No Results Found