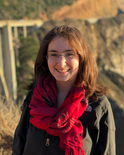
Vera Axelrod
I joined Google's natural language research efforts in 2016, focusing on reference problems such as coreference resolution and language identification.
I completed my BS in mathematics at Rensselaer Polytechnic Institute in 2013 and worked for three years on Google's Ad Traffic Quality team before moving to Research.
Research Areas
Authored Publications
Google Publications
Other Publications
Sort By
Multimodal Language Identification
Shikhar Bharadwaj
Sriram (Sri) Ganapathy
Sid Dalmia
Wei Han
Yu Zhang
Proceedings of 2024 IEEE International Conference on Acoustics, Speech and Signal Processing (ICASSP 2024) (2024)
Preview abstract
Spoken language identification refers to the task of automatically predicting the spoken language in a given utterance. Conventionally, it is modeled as a speech-based language identification task. Prior techniques have been constrained to a single modality; however in the case of video data there is a wealth of other metadata that may be beneficial for this task. In this work, we propose MuSeLI, a Multimodal Spoken Language Identification method, which delves into the use of various metadata sources to enhance language identification. Our study reveals that metadata such as video title, description and geographic location provide substantial information to identify the spoken language of the multimedia recording. We conduct experiments using two diverse public datasets of YouTube videos, and obtain state-of-the-art results on the language identification task. We additionally conduct an ablation study that describes the distinct contribution of each modality for language recognition.
View details
XTREME-UP: A User-Centric Scarce-Data Benchmark for Under-Represented Languages
Sebastian Ruder
Shruti Rijhwani
Jean-Michel Sarr
Cindy Wang
John Wieting
Christo Kirov
Dana L. Dickinson
Bidisha Samanta
Connie Tao
David Adelani
Reeve Ingle
Dmitry Panteleev
Findings of the Association for Computational Linguistics: EMNLP 2023, Association for Computational Linguistics, Singapore, pp. 1856-1884
Preview abstract
Data scarcity is a crucial issue for the development of highly multilingual NLP systems. Yet for many under-represented languages (ULs) — languages for which NLP research is particularly far behind in meeting user needs — it is feasible to annotate small amounts of data. Motivated by this, we propose XTREME-UP, a benchmark defined by: its focus on the scarce-data scenario rather than zero-shot; its focus on user-centric tasks — tasks with broad adoption by speakers of high-resource languages; and its focus on under-represented languages where this scarce-data scenario tends to be most realistic. XTREME-UP evaluates the capabilities of language models across 88 under-represented languages over 9 key user-centric technologies including ASR, OCR, MT, and information access tasks that are of general utility. We create new datasets for OCR, autocomplete, semantic parsing, and transliteration, and build on and refine existing datasets for other tasks. XTREME-UP provides methodology for evaluating many modeling scenarios including text only, multi-modal (vision, audio, and text), supervised parameter tuning, and in-context learning. We evaluate commonly used models on the benchmark. We release all code and scripts to train and evaluate models.
View details
Label Aware Speech Representation Learning For Language Identification
Shikhar Bharadwaj
Sriram Ganapathy
Wei Han
Proceedings of Interspeech 2023, pp. 5351-5355
Preview abstract
The speech representation learning approaches, for nonsemantic tasks like language recognition, have either explored supervised embedding extraction methods using a classifier model or the self-supervised representation learning approach using raw data. In this paper, we propose a novel framework of combining the self-supervised representation learning with the language label information for the pre-training task. This framework, termed as label aware speech representation learning (LASR), uses a triplet based objective function to incorporate the language labels along with the self-supervised loss function. The speech representations are further fine-tuned for the identification task. The language recognition experiments are performed on two public datasets - FLEURS and Dhwani. In these experiments, we illustrate that the proposed LASR framework improves over the state-of-art systems in terms of recognition performance. We also report an analysis of the robustness of the LASR approach to noisy/missing labels as well as the application of the LASR model for downstream multi-lingual speech recognition tasks.
View details
FLEURS: Few-shot Learning Evaluation of Universal Representations of Speech
Alexis Conneau
Simran Khanuja
Yu Zhang
Siddharth Dalmia
Clara Rivera
IEEE Spoken Language Technology Workshop (SLT) (2022)
Preview abstract
We introduce FLEURS, the Few-shot Learning Evaluation of Universal Representations of Speech benchmark. FLEURS is an n-way parallel speech dataset in 102 languages built on top of the machine translation FLoRes-101 benchmark, with approximately 12 hours of speech supervision per language. FLEURS can be used for a variety of speech tasks, including Automatic Speech Recognition (ASR), Speech Language Identification (Speech LangID), Translation and Retrieval. In this paper, we provide baselines for the tasks based on multilingual pre-trained models like mSLAM. The goal of FLEURS is to enable speech technology in more languages and catalyze research in low-resource speech understanding.
View details
Building Machine Translation Systems for the Next Thousand Languages
Julia Kreutzer
Mengmeng Niu
Pallavi Nikhil Baljekar
Xavier Garcia
Maxim Krikun
Pidong Wang
Apu Shah
Macduff Richard Hughes
Google Research (2022)
Mind the GAP: A Balanced Corpus of Gendered Ambiguous Pronouns
Transactions of the Association for Computational Linguistics, vol. 6 (2018), pp. 605-618
Preview abstract
Coreference resolution is an important task for natural language understanding, and the resolution of ambiguous pronouns a longstanding challenge. Nonetheless, existing corpora do not capture ambiguous pronouns in sufficient volume or diversity to accurately indicate the practical utility of models. Furthermore, we find gender bias in existing corpora and systems favoring masculine entities. To address this, we present and release GAP, a gender-balanced labeled corpus of 8,908 ambiguous pronoun–name pairs sampled to provide diverse coverage of challenges posed by real-world text. We explore a range of baselines that demonstrate the complexity of the challenge, the best achieving just 66.9% F1. We show that syntactic structure and continuous neural models provide promising, complementary cues for approaching the challenge.
View details
No Results Found